Wikis
Info-nuggets to help anyone understand various concepts of MLOps, their significance, and how they are managed throughout the ML lifecycle.
Precision-recall curve
The precision-recall curve depicts the tradeoff between precision and recalls for various thresholds.
When the classes are severely imbalanced, precision-recall is a helpful indicator of prediction success. In information retrieval, recall measures the amount of truly relevant results returned, whereas precision measures the relevancy of the results.
The precision-recall curve depicts the tradeoff between precision and recalls for various thresholds. A high area under the curve denotes both high recall and high precision, where High precision is correlated with a low false positive rate. In contrast, high recall is correlated with a low false negative rate. High scores indicate that the classifier is producing accurate (high precision) results and that the results are mostly positive (high recall).
Although it produces various results, most of the predicted results from a system with high recall but low precision are inaccurate compared to the training labels. In contrast, a system with high precision but low recall produces very few results, yet most of its predicted labels match the training labels. An ideal system with high precision and recall will provide a large variety of results, all of which will be correctly labelled.
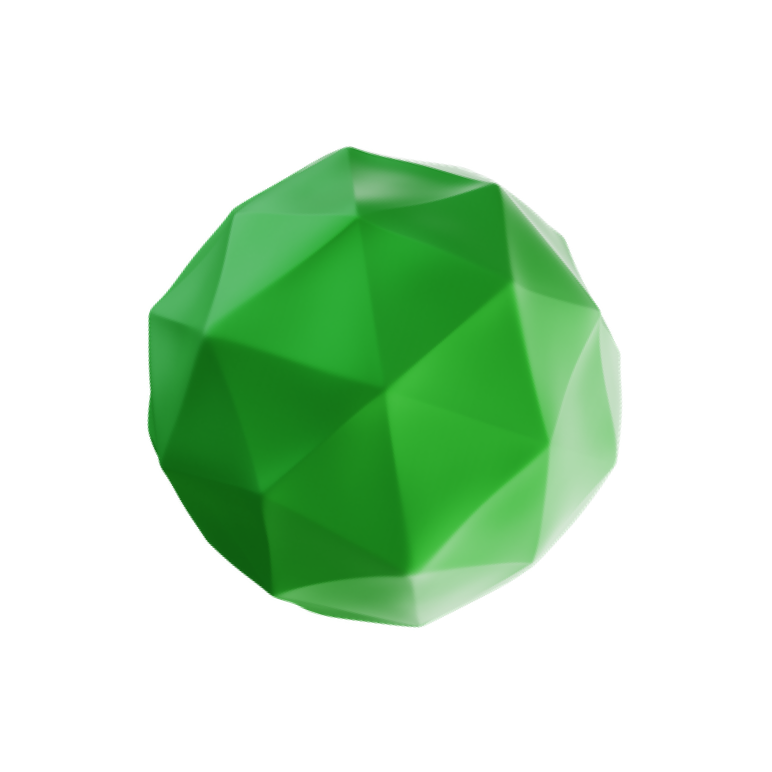
Is Explainability critical for your AI solutions?
Schedule a demo with our team to understand how AryaXAI can make your mission-critical 'AI' acceptable and aligned with all your stakeholders.