Wikis
Info-nuggets to help anyone understand various concepts of MLOps, their significance, and how they are managed throughout the ML lifecycle.
Fairness/ Bias Monitoring
Bias is the situation where the model consistently predicts distorted results because of incorrect assumptions.
Monitoring fairness and bias in AI
Fairness or bias can be broadly defined as the absence of discrimination or favouritism toward a person or group based on their characteristics. Even with perfect data, our modelling techniques may still result in bias. In its simplest terms, bias is the situation where the model consistently predicts distorted results because of incorrect assumptions. When we train our model on a training set and evaluate it on a training set, a biased model produces significant losses or errors.
Data scientists and ML engineers can regularly check predictions for bias with the help of bias monitoring. It gives them more profound insight into their training data and models to detect and mitigate bias and justify ML predictions.
Different ways to calculate bias
Disparity Impact ratio:
Disparity metrics can evaluate and contrast a model's performance across several groups as ratios or differnces.
- Disparity in model performance: These metrics sets determine the disparity (difference) in values of the selected performance metric across several subgroups.
- Disparity in selection rate: This measure includes the difference in selection rates between various subgroups.
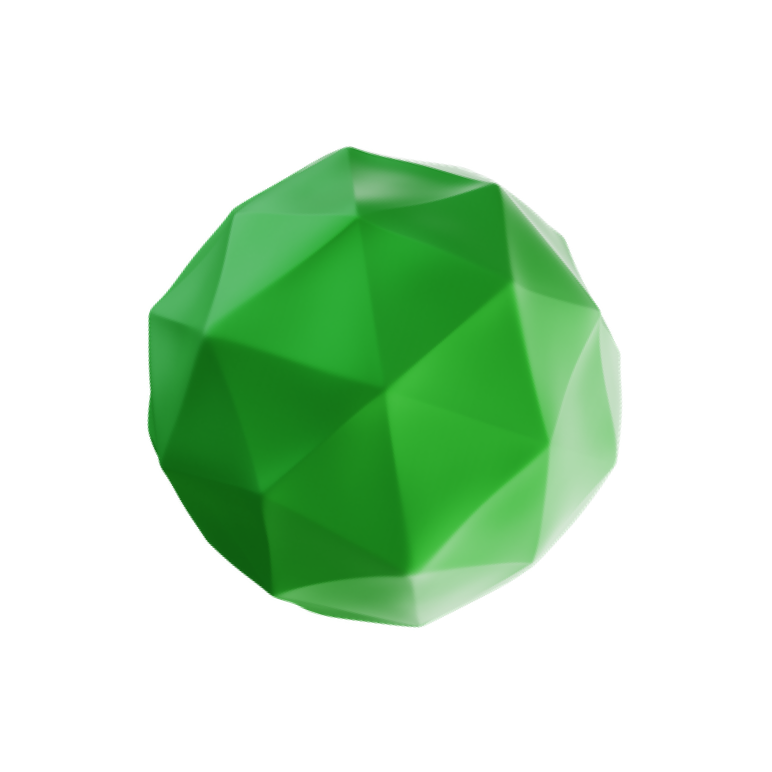
Is Explainability critical for your AI solutions?
Schedule a demo with our team to understand how AryaXAI can make your mission-critical 'AI' acceptable and aligned with all your stakeholders.