AryaXAI Research
Our research is dedicated to addressing fundamental challenges in making AI acceptable, safe, and aligned. We strive to ensure AI operates with certainty, consistently serving the core objectives of its users.
Our Mission
Our mission is to tackle fundamental challenges in AI, focusing on critical areas such as explainability, safety, and alignment—key pillars for achieving meaningful and reliable AI outcomes.
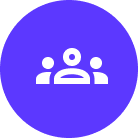
Build new explainability tools
Invent, test and validate new explainability techniques
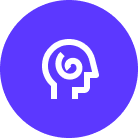
Experimenting alignment
From synthetic alignment to model editing, find ways to align
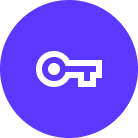
Break and fix 'AI' to make it safe
Identify gaps, risks and find ways to mitigate them
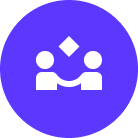
Make it scalable and usable
From modifying frameworks to writing CUDA kernels to make them scalable.
AI Explainability
As AI models become increasingly complex, achieving interpretability and explainability has become a significant challenge, often making it nearly impossible to fully comprehend their inner workings.
Our research is dedicated to developing innovative explainability techniques and evaluation methods that deliver accurate, model-consistent, and diverse explanations, ensuring AI remains interpretable for all stakeholders. These advancements pave the way for more effective practices such as model pruning, editing, and compression, enabling the creation of optimized, efficient models—an alternative to merely scaling systems through brute force.
New AI explainability techniques
Backtrace, is a new technique created by the AryaXAI research team to explain any deep learning model, more accurately. Backtrace can work for any deep learning model from ResNet to LLMs. Check more examples in our research papers or explore hands-on examples in the Playground
Continue to find more new XAI techniques.
Explainability evaluations
Our research emphasizes designing robust evaluation frameworks to assess the quality and effectiveness of AI explanations. By developing metrics that measure accuracy, consistency, and diversity of explanations, we aim to ensure AI systems provide clear, reliable, and stakeholder-appropriate insights into their decision-making processes.
Explainability-Driven Model Refinement
Our research explores explainability as a foundation for advanced techniques like model pruning, editing, and compression. By understanding how models make decisions, we can identify and remove redundancies, fine-tune parameters, and streamline architectures—enabling the creation of more efficient and high-performing AI systems without compromising their integrity or functionality.
AI Alignment
Our research focuses on pioneering innovative approaches to model alignment, ensuring AI systems operate in harmony with user goals and ethical standards. This includes exploring methods like synthetic model training and model pruning to fine-tune performance. By aligning models effectively, we aim to enhance their reliability, efficiency, and real-world applicability.
Synthetic Alignment
Exploring advanced synthetic alignment techniques that use synthetic data to improve model alignment with user objectives. This approach enhances model adaptability and ensures AI systems can handle diverse real-world scenarios at scale.
Adaptive Model Pruning
Developing methods to streamline AI models by removing unnecessary components without compromising functionality. This not only optimizes model performance but also ensures alignment with intended goals by eliminating potential sources of bias or inefficiency.
Feedback-Driven Alignment
Integrating continuous feedback loops to refine and align AI behavior with user values and expectations. By leveraging real-world feedback, we ensure models remain adaptable, responsive, and closely aligned with evolving requirements.
AI Safety
Our research in model safety focuses on identifying vulnerabilities and gaps in AI systems to ensure robust and secure operation. By uncovering potential weaknesses, we aim to develop innovative methods to address and rectify these issues, minimizing risks and ensuring AI models perform reliably and responsibly in real-world scenarios.
Robustness and Stress Testing
Creating rigorous testing frameworks to evaluate model performance across diverse and dynamic environments. This ensures AI systems maintain their integrity and safety, even under challenging or unexpected conditions.
Vulnerability Detection
Researching advanced techniques to identify weaknesses and potential failure points in AI models. This includes analyzing edge cases, adversarial scenarios, and performance under stress to ensure models remain secure and dependable.
Risk Mitigation Strategies
Developing proactive measures and protocols to address identified gaps in AI models. This involves designing mechanisms to prevent unintended behaviors and minimize the impact of vulnerabilities on real-world applications.
Featured Paper
Research Papers

xai_evals : A Framework for Evaluating Post-Hoc Local Explanation Methods

Bridging the Gap in XAI—The Need for Reliable Metrics in Explainability and Compliance
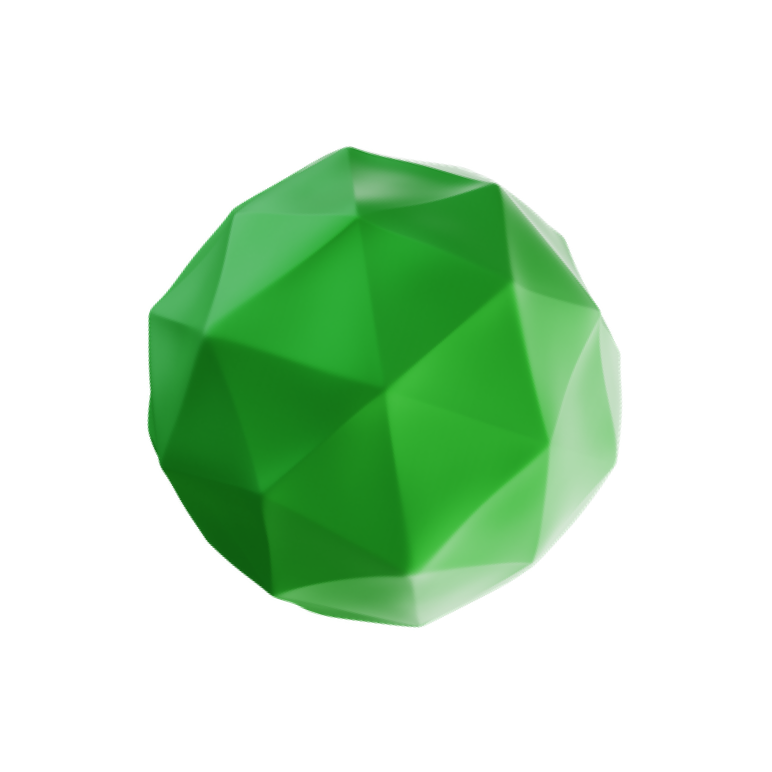
Is Explainability critical for your AI solutions?
Schedule a demo with our team to understand how AryaXAI can make your mission-critical 'AI' acceptable and aligned with all your stakeholders.