Mamba
Innovative state-space model (SSM) designed to handle complex, data-intensive sequences
Mamba is an innovative state-space model (SSM) designed to efficiently handle complex, data-intensive sequences. Researchers Albert Gu and Tri Dao developed the model, which was published in the paper "Mamba: Linear-Time Sequence Modeling with Selective State Spaces."
Mamba is highly efficient in processing complex sequences in language processing, genomics, and audio analysis. Its linear-time sequence modelling with selective state spaces ensures exceptional performance across all modalities.
What sets Mamba apart is its distinctive Selective State Space Modeling (SSM) layer, rapid processing capacity, and a hardware-aware parallel algorithm in recurrent mode. These features contribute to swift inference and linear scaling concerning sequence length.
Mamba, in comparison to Transformers like GPT-4, stands out for its efficiency in processing long sequences within natural language processing. While Transformers have set benchmarks in the field, their efficiency diminishes with longer inputs. Mamba addresses this limitation with its unique architecture, offering enhanced efficiency and streamlined processing for extended sequences. This positions Mamba as a promising alternative in scenarios where the effective handling of lengthy inputs is crucial.
Liked the content? you'll love our emails!
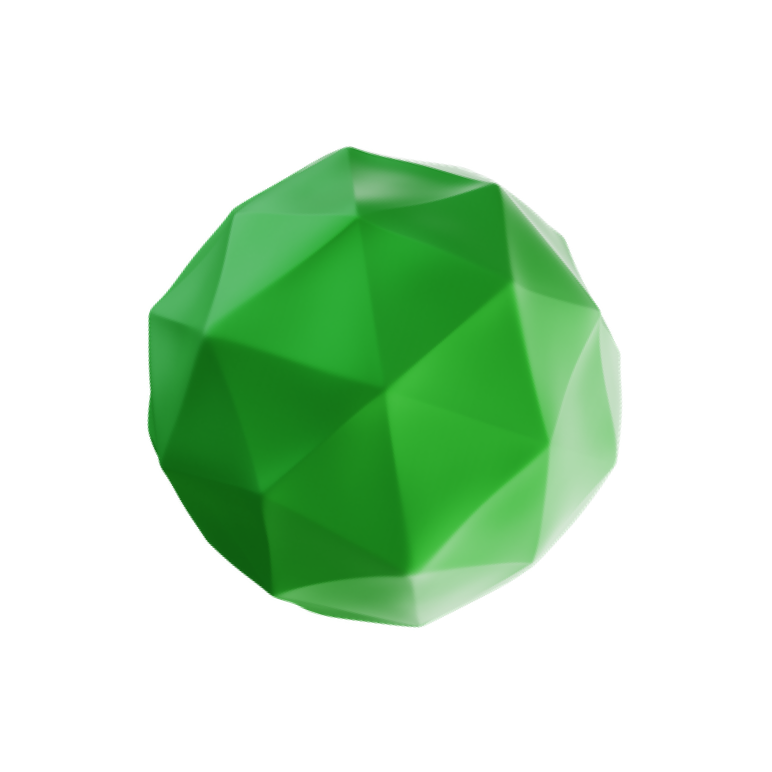
Is Explainability critical for your 'AI' solutions?
Schedule a demo with our team to understand how AryaXAI can make your mission-critical 'AI' acceptable and aligned with all your stakeholders.