ML Observability: Redesigning the ML lifecycle
August 26, 2022
.png)
Many businesses today interact with Machine Learning systems on a regular basis. There are more technologies being embedded in the decision-making processes than ever before, with the objective of propelling the movement towards leveraging AI in business.
However, even today, the adoption of AI in vertical-specific use cases is largely driven by general-purpose tools. To understand more about this, let us first dive into the topic of the ML adoption journey.
The ML adoption journey is confusing and complex, with multiple options available
Every ML lifecycle roughly follows the same workflow, consisting of various micro and macro steps. Depending on the scope and requirement, the adoption environment can be broadly classified into two categories:
Horizontal platforms
Horizontal platforms are general-purpose platforms that are designed for everyone, regardless of the industry. They don’t own the industry's core problem - any user can come onboard and start using the platform. Any data scientist can use these tools/APIs and deploy AI capabilities. They are use-case agnostic and focus on broad-range problem statements.
Vertical platforms
Vertical platforms are industry-specific platforms, specialized for a specific vertical with all relevant, vertical-specific requirements. They focus on a single use case that is central to sustaining the company. Rather than having a generalized problem-solving approach and then applying it to industry-specific problems, these systems are built uniquely to solve vertical-specific problems, doing the best job.
Next, comes the implementation. Here’s a quick overview of the steps involved in the journey:
1. Problem understanding
2. Data preparation:
- Data collection
- Data Validation
3. Model building:
- Model exploration
- Model training
- Model evaluation
4. Production:
- Model deployment
- Model monitoring
The teams first collect and prepare the necessary data, develop and test the model, and then deploy and monitor the model. All of these steps together combined create the Machine learning model lifecycle. Each step is unique in itself, requiring different variations in resources and time.
Making the shift from POC to a model that actually works is drastically different in the real world. There are hundreds of things that can go wrong while applying the model to a real-world use case - changing data distributions, changes in data, changes in performance in training vs. production, etc. From the moment your model is deployed, it starts degrading. Hence businesses should never stop at model deployment. What’s next?
Beyond Monitoring - ML observability
Initially, the success of such ML projects was dependent on successful model deployment. However, It is important to note that Machine learning models are dynamic in nature - their performance needs to be monitored, or it degrades over time.
Practitioners would want to be the first to know when a problem arises and work on resolving it quickly. This practice is referred to as ML monitoring.
ML monitoring in machine learning is the method of tracking the performance metrics of a model from development to production.
Monitoring encompasses establishing alerts on key model performance metrics such as accuracy and drift. The practice helps identify precisely when the model performance starts diminishing. Monitoring the automated workflows helps to maintain the required accuracy and keeps transformations error-free.
While businesses want to know when a problem has arisen, they are more interested in knowing why the problem arose in the first place. This is where ML Observability comes in.
ML observability provides deep insights into the model health. It entails tracking the performance of ML systems across their lifecycle, right from when it's being built, to pre and post-production, but ML observability also brings a proactive approach to investigating model issues and highlighting the root cause of the problem.
Observability covers a larger scope compared to ML monitoring - it understands why the problem exists, and the best way to resolve it.
Observability examines the outcomes of the system as a whole rather than just the monitors for each system component.
Why is ML observability needed?
Enterprises invest heavily in building, testing and maintaining ML models for mission-critical functions, yet face several challenges with model performance, explainability or ensuring consistency in production. The deployed model might work for one product and not another, or work for a particular type of prediction and not another, or its performance may simply degrade over time.
ML observability is used to handle the root-cause analysis across the ML project lifecycle. It helps to understand the ‘why’ and figure out ‘what’ needs to be done to resolve the problem, bringing organizations a step closer to responsible AI.
Businesses can gain granular insights into overall model health. ML observability provides a quick, easily interpretable visualization, with the ability to slice and dice into the problem, suitable for multiple stakeholders, even non-technical ones. It helps to pinpoint why the model is not performing as expected in production and gives clarity on rectifying it - be it retraining the model, updating datasets, adding new features, etc.
Hence, this radically changes the wheel of ML journey -
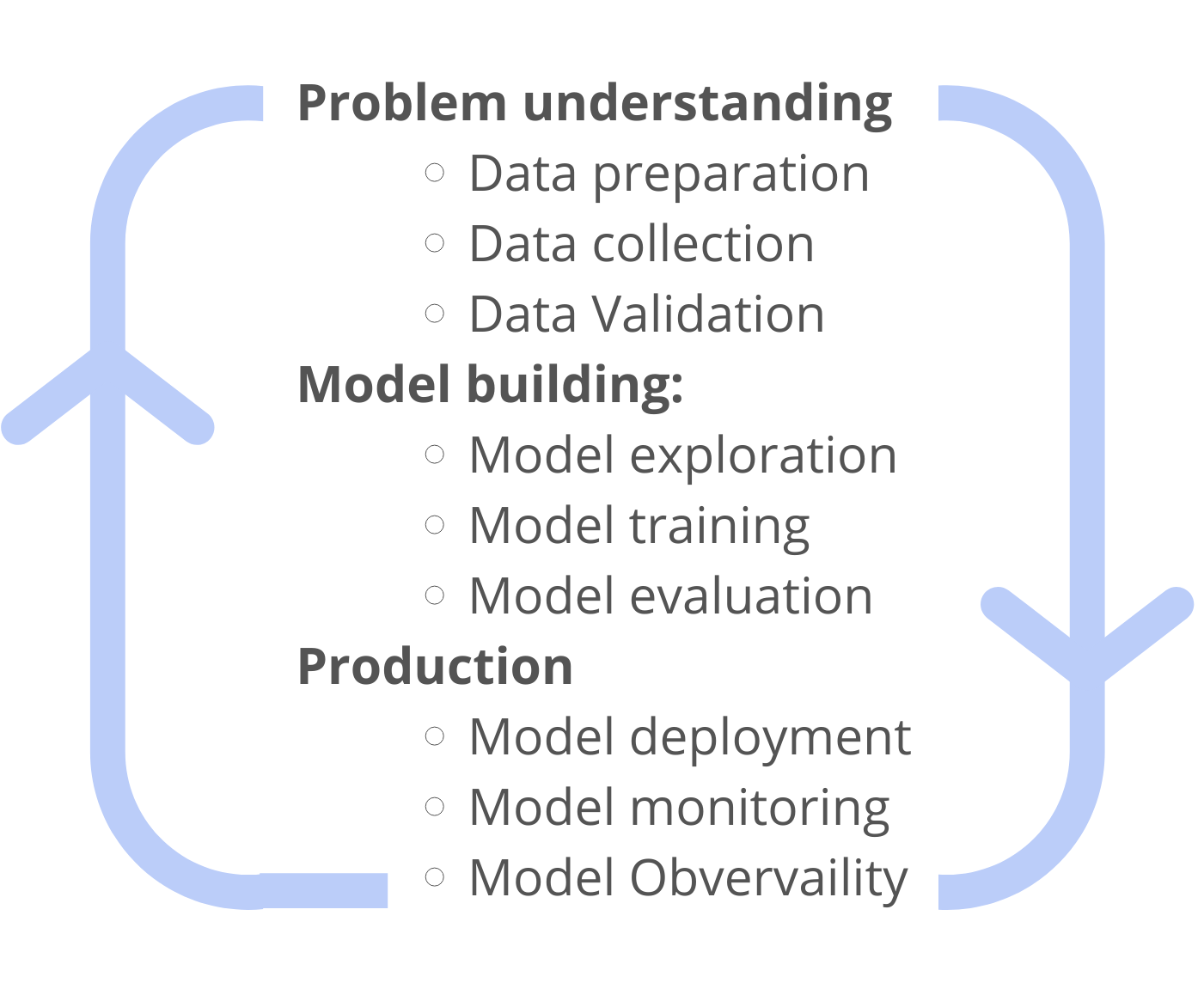
SHARE THIS
Discover More Articles
Explore a curated collection of in-depth articles covering the latest advancements, insights, and trends in AI, MLOps, governance, and more. Stay informed with expert analyses, thought leadership, and actionable knowledge to drive innovation in your field.
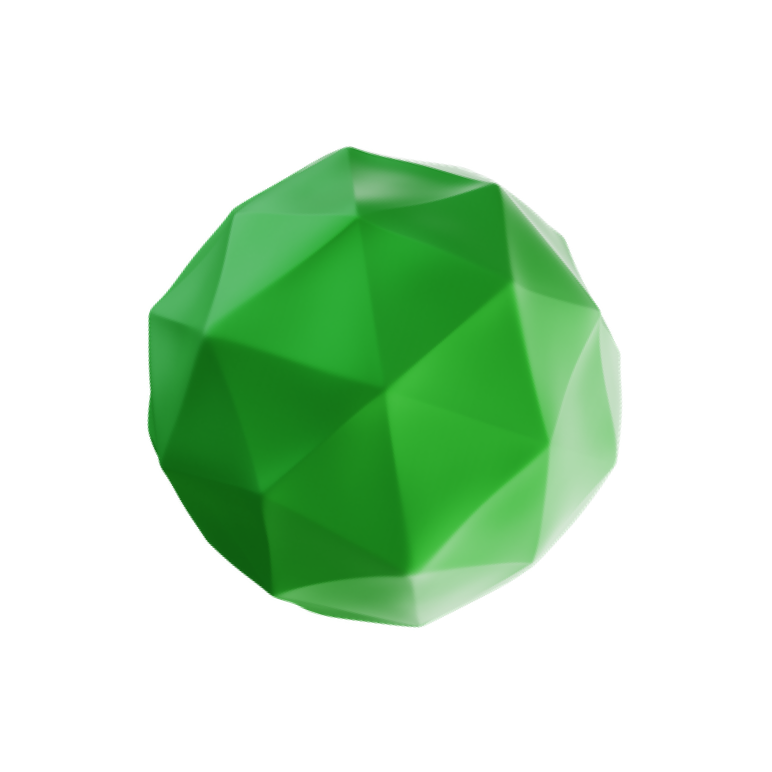
Is Explainability critical for your 'AI' solutions?
Schedule a demo with our team to understand how AryaXAI can make your mission-critical 'AI' acceptable and aligned with all your stakeholders.