The Ethics of AI-Powered Decision-Making: Can We Eliminate Bias?
7 minutes
March 18, 2025
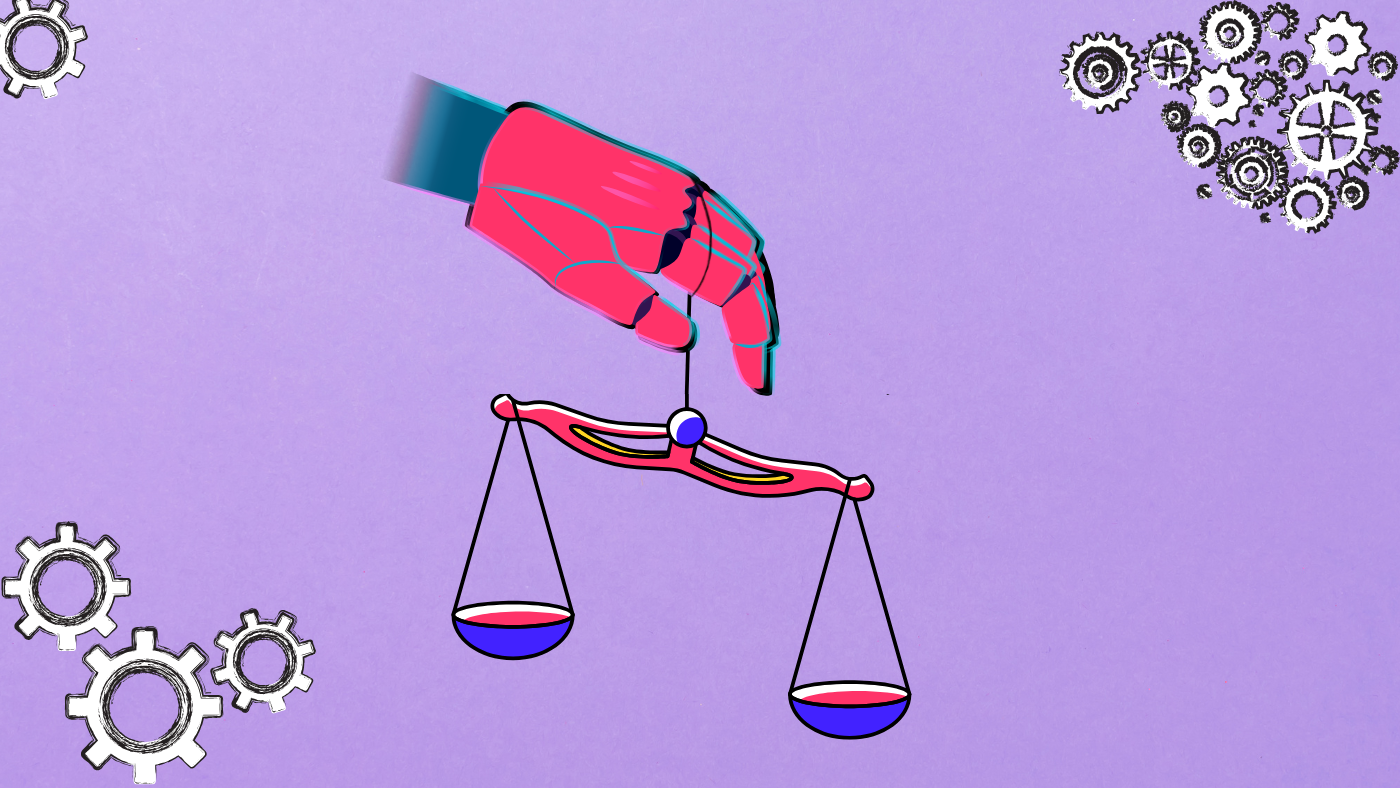
The Ethical Dilemma of AI Bias
Artificial Intelligence (AI) is reshaping industries, driving efficiencies, and automating complex decision-making processes. However, one of the biggest ethical challenges in AI is bias. AI systems, despite their promise of objectivity, are only as fair as the data and algorithms they rely on.
AI is only as unbiased as the data and algorithms behind it. If training data contains historical prejudices or societal biases, AI models will inevitably learn and replicate them. Additionally, algorithmic choices, feature selection, and data processing methodologies can all introduce or exacerbate bias in AI-powered decision-making.
Ethical AI is critical for fostering trust, ensuring fairness, and adhering to regulatory frameworks such as the EU AI Act and other global policies. Businesses and institutions that fail to implement ethical AI practices risk reputational damage, regulatory penalties, and loss of consumer trust.
This raises a fundamental question: Can AI-powered decision-making ever be truly free of bias? While complete bias elimination may be unattainable, AI can be designed to minimize bias, ensuring decisions are as fair and transparent as possible.
Understanding AI Bias: How It Creeps Into Decision-Making
Bias in AI manifests in various forms, originating from the way data is collected, processed, and interpreted by algorithms. It can be introduced at multiple stages, including dataset creation, model training, and user interactions. Some of the most common types of AI bias include:
1. Data Bias
AI models learn from historical data, and if that data is incomplete, imbalanced, or contains historical inequalities, the AI system will inherit those biases. For example:
- Sampling Bias: If a dataset underrepresents certain demographics, AI models may make inaccurate or unfair predictions for those groups. A hiring algorithm trained mostly on male resumes may favor male candidates.
- Historical Bias: If past data reflects discriminatory practices, AI can perpetuate them. For instance, AI-powered credit scoring systems may disadvantage minority groups due to past lending discrimination.
- Labeling Bias: If data is labeled with human judgment, subjective biases can influence outcomes. For example, sentiment analysis tools trained on biased internet comments may unfairly associate certain dialects or languages with negative emotions.
2. Algorithmic Bias
Even if the data is unbiased, AI models can still develop biases due to the way they process information and make predictions. Some ways algorithmic bias can emerge include:
- Feature Selection Bias: If an algorithm overemphasizes certain features, it can reinforce stereotypes. For example, a job-matching AI may prioritize Ivy League degrees, disproportionately favoring privileged applicants.
- Optimization Bias: Many AI models prioritize accuracy over fairness. If an AI system is optimized for profit rather than ethical considerations, it may disadvantage certain groups.
- Proxy Bias: When models use correlated but inappropriate features as decision-making criteria. For example, ZIP codes used in loan approvals may inadvertently reflect racial segregation patterns.
3. User Bias
AI models adapt based on human interactions, which can introduce additional biases over time. Some common types of user bias include:
- Confirmation Bias: Users may reinforce AI biases by interacting more with AI-generated recommendations that align with their existing beliefs.
- Feedback Loop Bias: AI-powered platforms like social media algorithms can amplify biases by continuously serving content that aligns with past engagement patterns.
- Cultural Bias: AI tools deployed in different cultural contexts may misinterpret user input if they were not trained on diverse datasets.
Bias persists because AI models are built on human and historical data. Even with the best efforts, eliminating bias entirely is challenging because societal inequities and subjective decision-making influence AI training and development. However, recognizing these biases is the first step toward developing fairer AI systems. (For a deeper dive into bias types, see our previous blog on Biases in ML models).
Ethical Frameworks for AI Decision-Making
As AI systems play an increasingly critical role in decision-making, ensuring fairness, transparency, and accountability is essential. Ethical frameworks help mitigate bias, misinformation, and unintended consequences, making AI more responsible and aligned with human values.
1. Fairness & Transparency: The Need for Explainable AI (XAI)
AI decisions must be interpretable to build trust and prevent bias. Explainable AI (XAI) ensures that AI models provide clear reasoning behind their outputs, making them auditable and understandable for users. Techniques like AryaXAI’s DLBacktrace allow developers to trace AI decisions back to specific neural activations, identifying potential biases and improving transparency. Without XAI, AI models risk becoming black boxes, making it difficult to assess fairness in automated decisions.
2. Accountability: Who is Responsible for Biased AI Decisions?
One of the biggest ethical challenges in AI governance is determining responsibility for biased decisions. If an AI system unfairly denies a loan, misdiagnoses a patient, or misinterprets legal information, who is accountable? Is it the developer, the deploying organization, or regulatory bodies? Ethical AI frameworks call for clear responsibility allocation, ensuring that businesses take ownership of AI outcomes, regularly audit their models, and implement bias mitigation strategies to maintain fairness.
3. Regulatory Landscape: Enforcing Fairness in AI
Governments and organizations are actively establishing regulations to ensure AI fairness and ethical deployment. Some key frameworks include:
- EU AI Act – Imposes strict guidelines on high-risk AI applications, particularly those affecting human rights, financial access, and employment.
- U.S. AI Bill of Rights – Defines core principles for non-discriminatory AI, emphasizing fairness, privacy, and accountability.
- ISO AI Ethics Standards – Global standards promoting responsible AI development, risk assessment, and fairness benchmarks.
As AI adoption accelerates, these frameworks will play a crucial role in ensuring safe, unbiased, and ethical AI across industries. Organizations that proactively align with these regulations will enhance trust, minimize legal risks, and build more responsible AI systems for the future.
How to Mitigate Bias in AI-Powered Decision-Making
While eliminating bias entirely may be unrealistic, organizations can adopt strategies to minimize its impact:
1. Explainable AI (XAI): Ensuring Model Transparency
XAI techniques like DLBacktrace enable organizations to understand and audit AI decisions. Transparent AI models allow stakeholders to detect and rectify biases before they lead to harmful outcomes.
2. Diverse & Representative Data: Reducing Bias at the Source
AI models should be trained on datasets that are diverse, inclusive, and representative of real-world populations. Techniques such as data augmentation and bias-balancing algorithms can improve fairness.
3. Human Oversight: The Role of AI Ethics Committees and Auditors
Organizations should establish AI ethics committees to monitor AI decisions, ensure compliance with ethical guidelines, and intervene when biases are detected.
4. Bias Testing & Fairness Audits: Proactively Detecting Bias
Regular AI audits using bias detection tools can help identify and mitigate disparities before AI systems go into production. Open-source fairness tools like AI Fairness 360 (AIF360) from IBM assist in bias testing and mitigation.
The Future of Ethical AI: Can We Achieve Truly Fair AI?
The ultimate goal of AI ethics is not necessarily to eliminate bias entirely (which may be impossible) but to achieve “minimally biased” AI—systems that actively mitigate harm and promote fairness.
Key advancements shaping the future of ethical AI include:
- Improved Fairness Metrics: New algorithms that quantitatively measure and reduce bias.
- Better Model Interpretability: Enhanced XAI frameworks to increase transparency.
- Stronger Ethical AI Governance: Evolving regulations to ensure AI accountability and fairness.
Organizations that prioritize responsible AI not only ensure compliance but also gain a competitive edge. Ethical AI fosters trust, improves brand reputation, and enhances customer satisfaction, making it a business advantage rather than just a regulatory necessity.
Conclusion
While AI bias remains a persistent challenge, organizations can take proactive steps to minimize its impact through explainability, diverse data, human oversight, and fairness audits. The pursuit of ethical AI is an ongoing journey—one that requires continuous improvements in governance, interpretability, and fairness measures.
By striving for responsible AI, businesses and policymakers can shape a future where AI-driven decision-making is more transparent, accountable, and equitable for all.
SHARE THIS
Discover More Articles
Explore a curated collection of in-depth articles covering the latest advancements, insights, and trends in AI, MLOps, governance, and more. Stay informed with expert analyses, thought leadership, and actionable knowledge to drive innovation in your field.
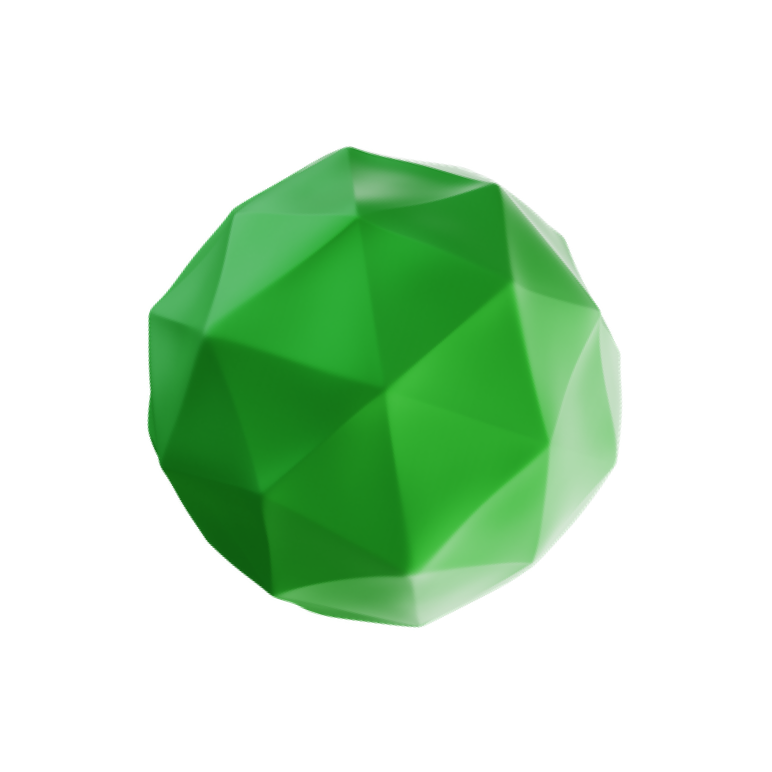
Is Explainability critical for your AI solutions?
Schedule a demo with our team to understand how AryaXAI can make your mission-critical 'AI' acceptable and aligned with all your stakeholders.