ML Observability
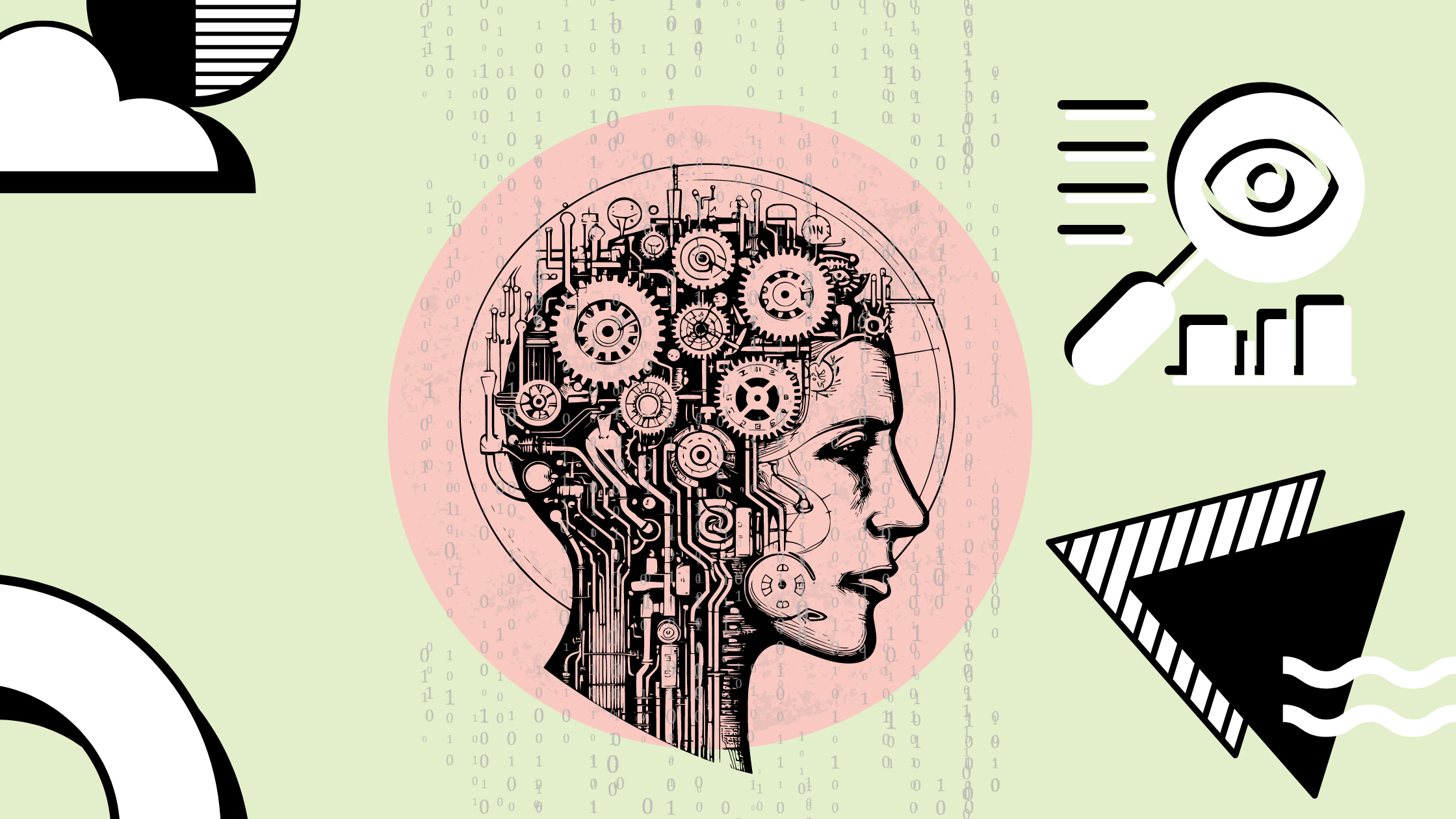
Article
July 5, 2024
Maximizing Machine Learning Efficiency with MLOps and Observability
As organizations navigate real-world complexities, it is essential to prioritize both MLOps and observability to create a solid foundation for building, maintaining, and scaling trustworthy ML models.
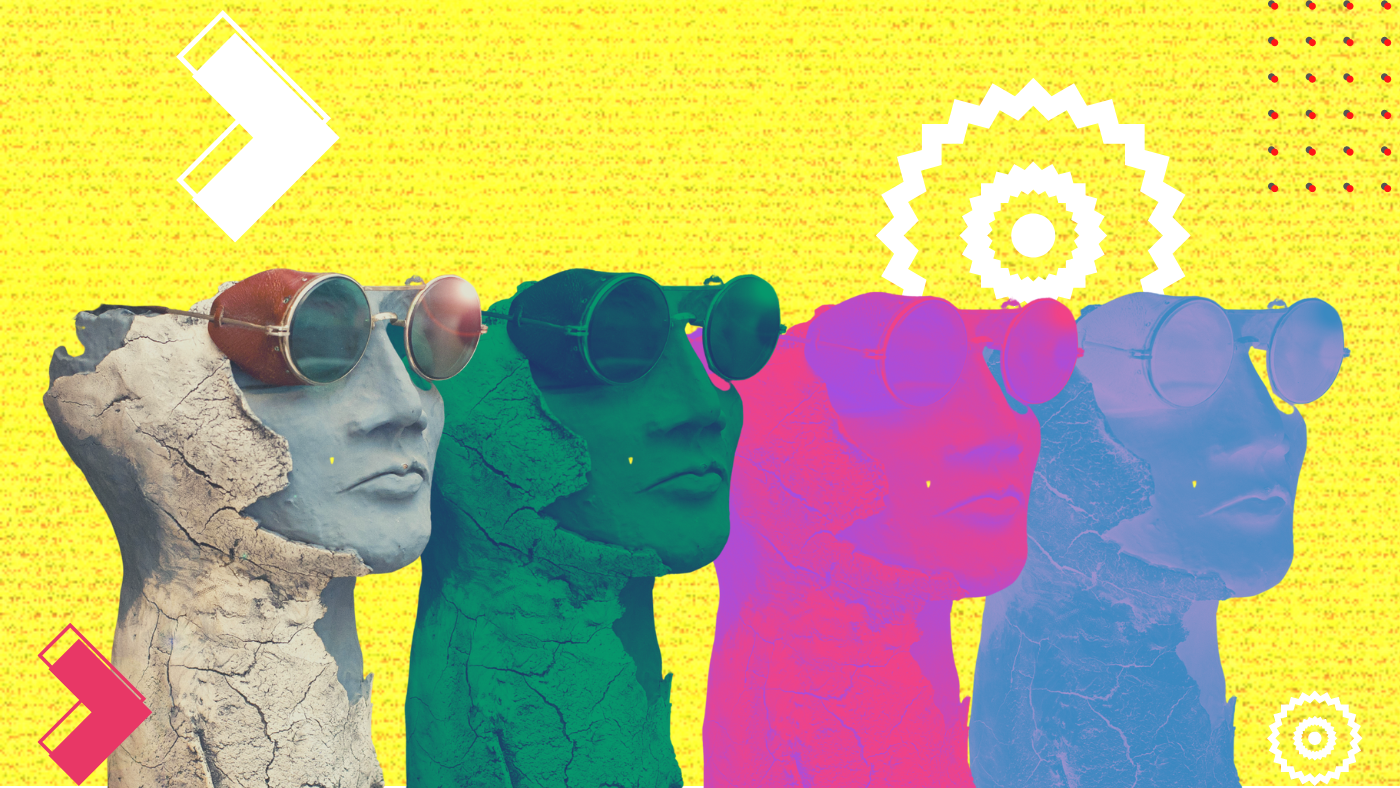
Article
March 14, 2023
AryaXAI Synthetics: Using synthetic ‘AI’ to compliment ‘ML Observability’
AryaXAI synthetics to resolve critical data gaps, test models at scale and preserve data privacy

News
December 27, 2022
Vinay Kumar Sankarapu, Co-Founder & CEO of Arya.ai – Interview Series
Interview with Unite.AI
.png)
Article
August 26, 2022
ML Observability: Redesigning the ML lifecycle
While businesses want to know when a problem has arisen, they are more interested in knowing why the problem arose in the first place. This is where ML Observability comes in.
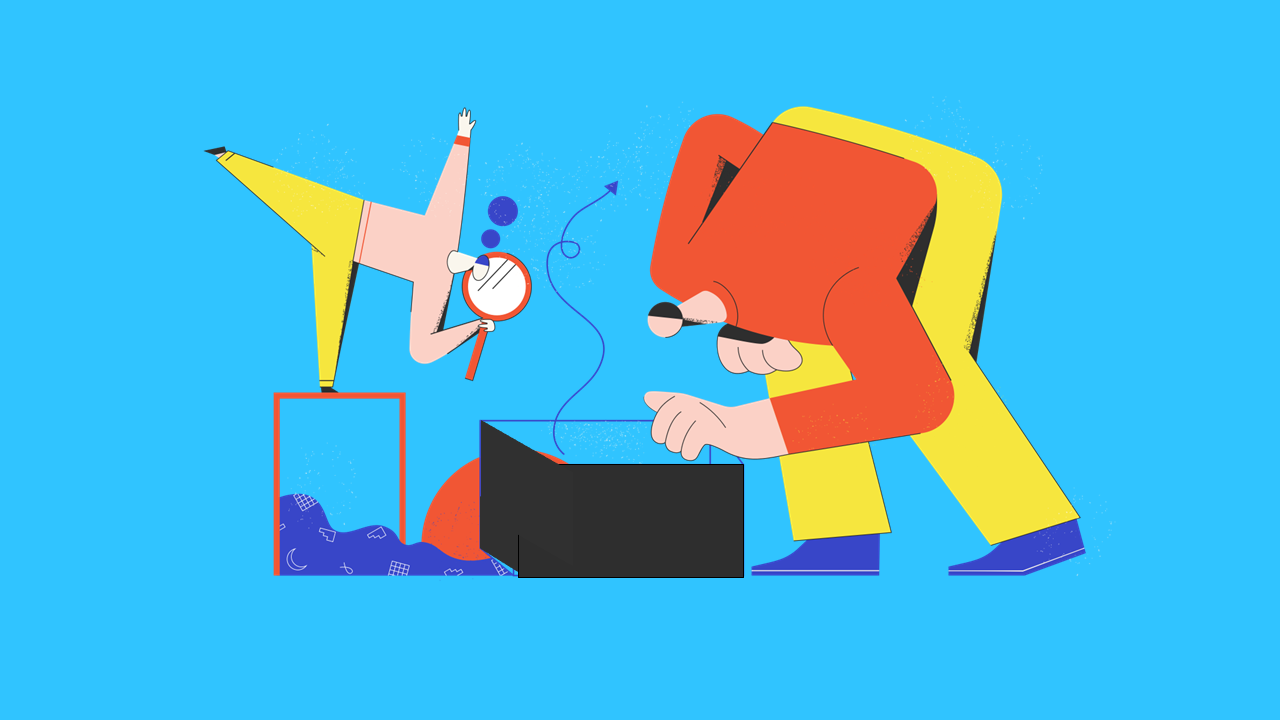
Article
August 24, 2022
Deep dive into Explainable AI: Current methods and challenges
As organizations scale their AI and ML efforts, they are now reaching an impasse - explaining and justifying the decisions by AI models. Also, the formation of various regulatory compliance and accountability systems, legal frameworks and requirements of Ethics and Trustworthiness, mandate making AI systems adhere to transparency and traceability
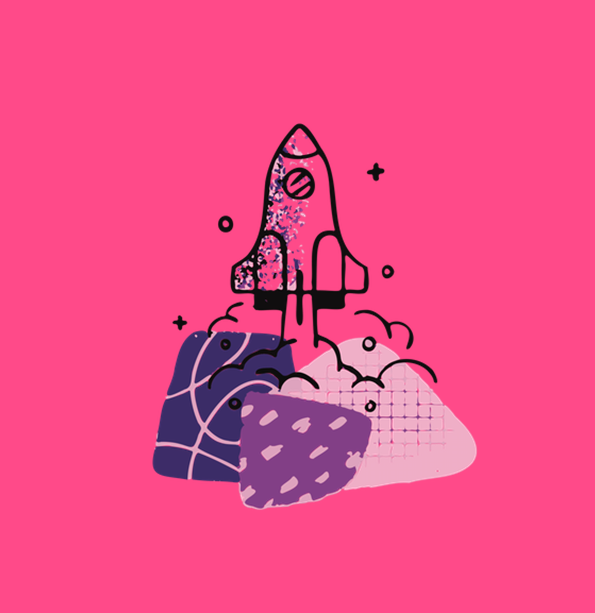
Article
August 18, 2022
AryaXAI - A distinctive approach to explainable AI
With packaged AI APIs in the market, more people are using AI than ever before, without the constraint of compute, data or R&D. This provides an easy entry point to use AI and gets users hooked for more. However, the first legal framework for AI is here! One of the many mandates in the proposal is to make AI systems adhere to transparency and traceability. These additional requirements highlight the ever-increasing need for Explainable AI.
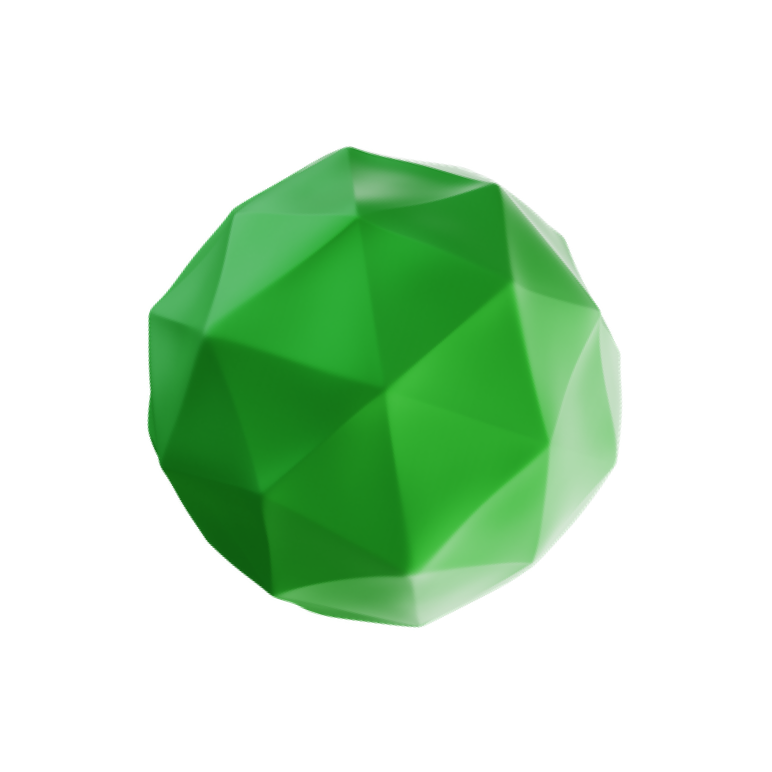
Is Explainability critical for your AI solutions?
Schedule a demo with our team to understand how AryaXAI can make your mission-critical 'AI' acceptable and aligned with all your stakeholders.