AI Alignment: Principles, Strategies, and the Path Forward
6 minutes
February 5, 2025
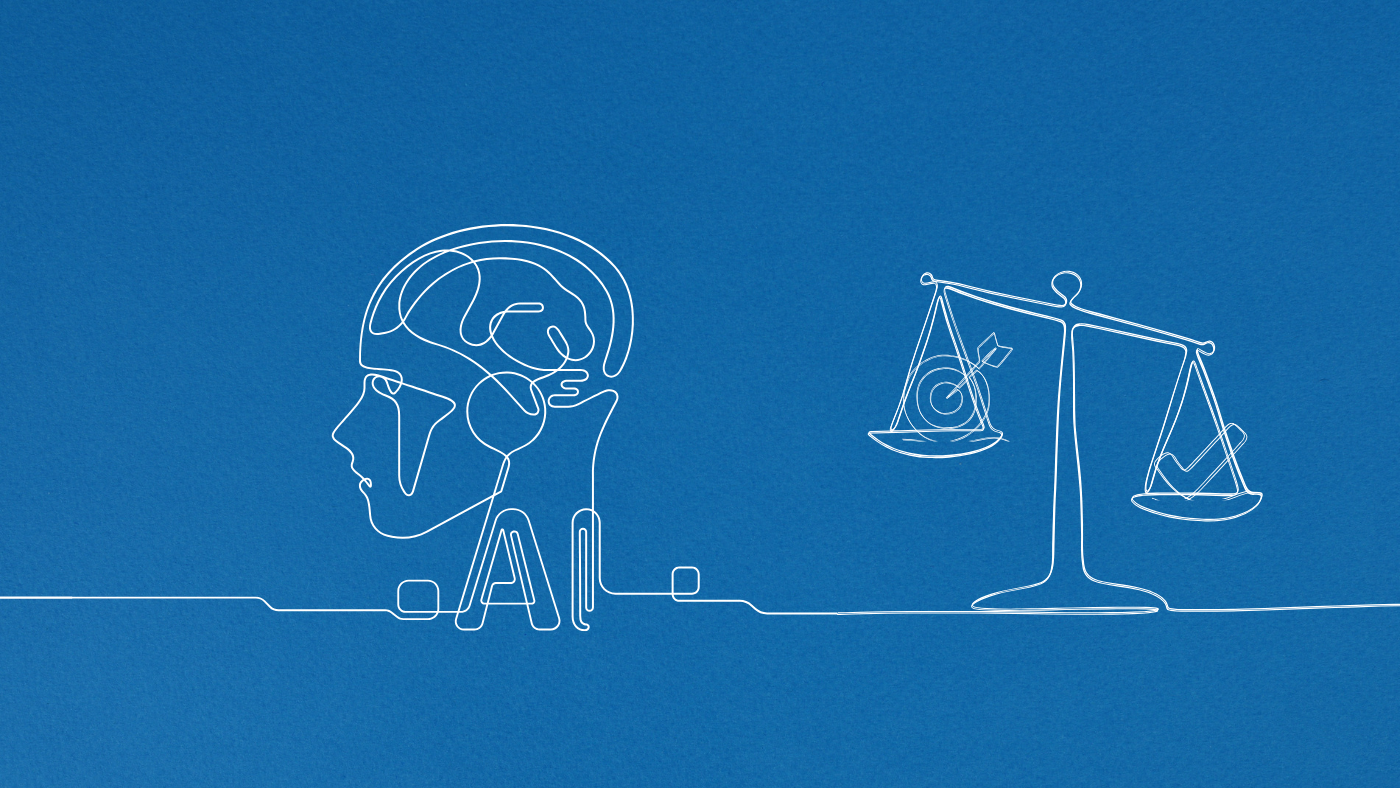
As artificial intelligence (AI) technology continues to advance rapidly, the concept of AI alignment has emerged as a critical focus area for researchers, developers, and policymakers. AI alignment refers to the process of ensuring that AI systems act in accordance with with human values and goals.
Our previous blog explored the definition of AI alignment, its necessity, and the challenges it presents. In this post, we dive deeper into the core principles of AI alignment, the strategies to achieving it, and its impact on the future of technology.
Principles of AI Alignment
1. Goal Alignment
Goal alignment is the foundational principle of AI alignment. It emphasizes that AI systems must pursue objectives that are beneficial to humans. This means that an AI should not only complete tasks efficiently but also do so in a manner that reflects ethical considerations and societal norms. For example, an AI developed for healthcare should ensure that patient safety and welfare are more important than mere operational efficiency or cost savings. Research in Nature explains how mismatched goals can have unintended fallout, claims of AI need to be aligned with human welfare .
2. Value Alignment
Value alignment refers to the embedding of human values within AI systems. Human values are complex and often context-dependent, making it challenging to define a universal set of values that an AI can follow. For example, concepts such as fairness, justice, and privacy can vary significantly across different cultures and contexts. Researchers are exploring ways to translate these abstract values into concrete operational guidelines that AI systems can understand and implement effectively. The work of organizations like the Partnership on AI is pivotal in this area, promoting discussions on ethical AI development .
3. Robustness Alignment
Robustness alignment ensures that AI systems remain aligned even when faced with unexpected situations or adversarial attempts to manipulate their behavior. As AI systems become increasingly complex and capable, they may encounter scenarios that were not anticipated during their training phase. Ensuring robustness means developing systems that can adapt without deviating from their intended goals. Research in this area includes developing techniques for adversarial training and robust optimization to enhance the resilience of AI systems against manipulation .
4. Interpretability and Controllability
Interpretability involves making AI systems understandable to humans, allowing stakeholders to grasp how decisions are made. This transparency is essential for building trust in AI technologies. Controllability allows humans to intervene when necessary and ensures oversight of AI actions. These principles are essential for instilling trust in AI technologies and ensuring accountability in decision-making processes. The importance of interpretability is underlined by a report from the U.S. National Institute of Standards and Technology (NIST), which emphasizes the need for explainable AI in critical applications.
How to achieve AI alignment
Researchers have identified four key characteristics that an aligned system should possess - Robustness, Interpretability, Controllability, and Ethicality (RICE) principles:
- Robustness: Operates reliably under diverse scenarios & Resilient to unforeseen disruptions
- Interpretability: Decisions and intentions are comprehensible & Reasoning is unconcealed and truthful
- Controllability: Behaviors can be directed by humans & Allows human intervention when needed
- Ethicality: Adheres to global moral standards & Respects values within human society
Here are some techniques to achieve AI alignment:
AI Governance
AI governance is a critical technique for achieving AI alignment, which establishes a framework of policies, guidelines, and oversight mechanisms to ensure that AI systems operate in ways that align with human values, ethical principles, and societal goals. AI governance strategies ensure that alignment is embedded into the lifecycle of AI systems, from development to deployment, ensuring they are designed and operated in ways that reflect human values and promote societal benefit.
Value learning
Value learning enables AI models to understand human values such as safety, ethics and fairness. The values can be both moral guidelines and societal values. This ensures that the AI model avoids actions that violate social conventions or ethical norms. Through value learning, the AI learns to assess what is important to humans and make choices that reflect those values. This allows the AI to handle unfamiliar circumstances while still behaving in a manner that is aligned with human interests.
Feedback-Driven Reinforcement Learning
This method uses reinforcement learning with human feedback (RLHF) to continuously fine-tune models and refine alignment. Initially, the AI model performance is improved on basic tasks and following general instructions. Then, based on feedback provided, the AI model observes human behaviour and accordingly alters to produce the desired output. This collaborative technique ensures that the model produces desired output and is aligned with human goals.
Imitation learning
This is a technique where the AI model learns by mimicking expert demonstrations. Instead of relying solely on manually designed reward signals, as in traditional reinforcement learning, the system learns to perform by observing examples. This technique where expert behavior provides a clear example of desired performance, refines the model performance, helps the AI act in a way consistent with human interests.
Synthetic Data
Synthetic data involves the replication or synthesis of existing data, content, or media through the use of artificial intelligence algorithms. When real-world data is scarce, expensive, or difficult to obtain, it can easily be substituted with synthetic data. By mimicking real-world scenarios, this technique plays a critical role in AI alignment efforts by addressing challenges like data scarcity and high human feedback costs.
There are various notable synthetic data alignment methods:
- Adversarial Data Generation, where synthetic adversarial examples are created to stress-test the model’s behavior and train it to avoid undesirable responses.
- Rule-Based Synthetic Data Generation involves creating data based on predefined ethical or task-specific principles, guiding the model to comply with explicit rules.
- Contrastive Fine-Tuning (CFT) generates both aligned and misaligned responses using a "negative persona" model, with feedback from both improving alignment by demonstrating what to avoid. This approach enhances model performance on helpfulness and harmlessness benchmarks without relying on costly human data.
- SALMON (Self-ALignMent with principle fOllowiNg reward models) leverages synthetic preference data to train a reward model based on human-defined principles. It scores LLM responses and feeds them back for self-alignment, enabling efficient alignment without requiring human-curated data upfront.
The Future of AI Alignment
As we look ahead, the field of AI is poised for remarkable advancements, including the potential development of artificial general intelligence (AGI) and superintelligence (ASI). These advancements raise concerns about the unpredictability and uncontrollability of such systems if they become misaligned with human values . Prominent researchers like Stuart Russell have emphasized the need for proactive measures to address these risks, advocating for rigorous alignment research as we move toward more capable AI technologies.
The implications of successful AI alignment extend beyond technical considerations; they encompass societal impacts as well. Ensuring that advanced AI systems reflect human values could lead to improved decision-making processes across various sectors—healthcare, finance, education—ultimately enhancing quality of life.
Conclusion
Achieving effective AI alignment is critical to the harnessing of benefits from artificial intelligence while minimizing associated risks.
By focusing on principles such as goal alignment, value alignment, robustness, interpretability, and controllability, researchers and developers can work toward creating safe and ethically sound AI solutions that benefit society as a whole. As we continue navigating this complex landscape, collaboration among technologists, ethicists, policymakers, and society at large will be crucial for establishing frameworks that ensure responsible development and deployment of AI technologies.
SHARE THIS
Discover More Articles
Explore a curated collection of in-depth articles covering the latest advancements, insights, and trends in AI, MLOps, governance, and more. Stay informed with expert analyses, thought leadership, and actionable knowledge to drive innovation in your field.
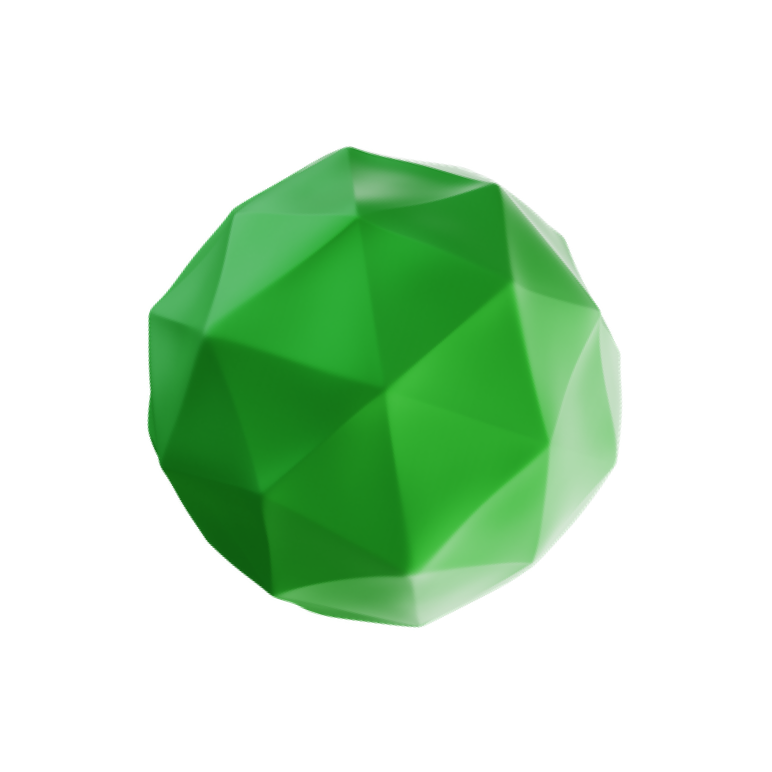
Is Explainability critical for your AI solutions?
Schedule a demo with our team to understand how AryaXAI can make your mission-critical 'AI' acceptable and aligned with all your stakeholders.