Understanding MLOps and LLMOps: Definitions, Differences, Challenges, and Lifecycle Management
10 minutes
April 30, 2025
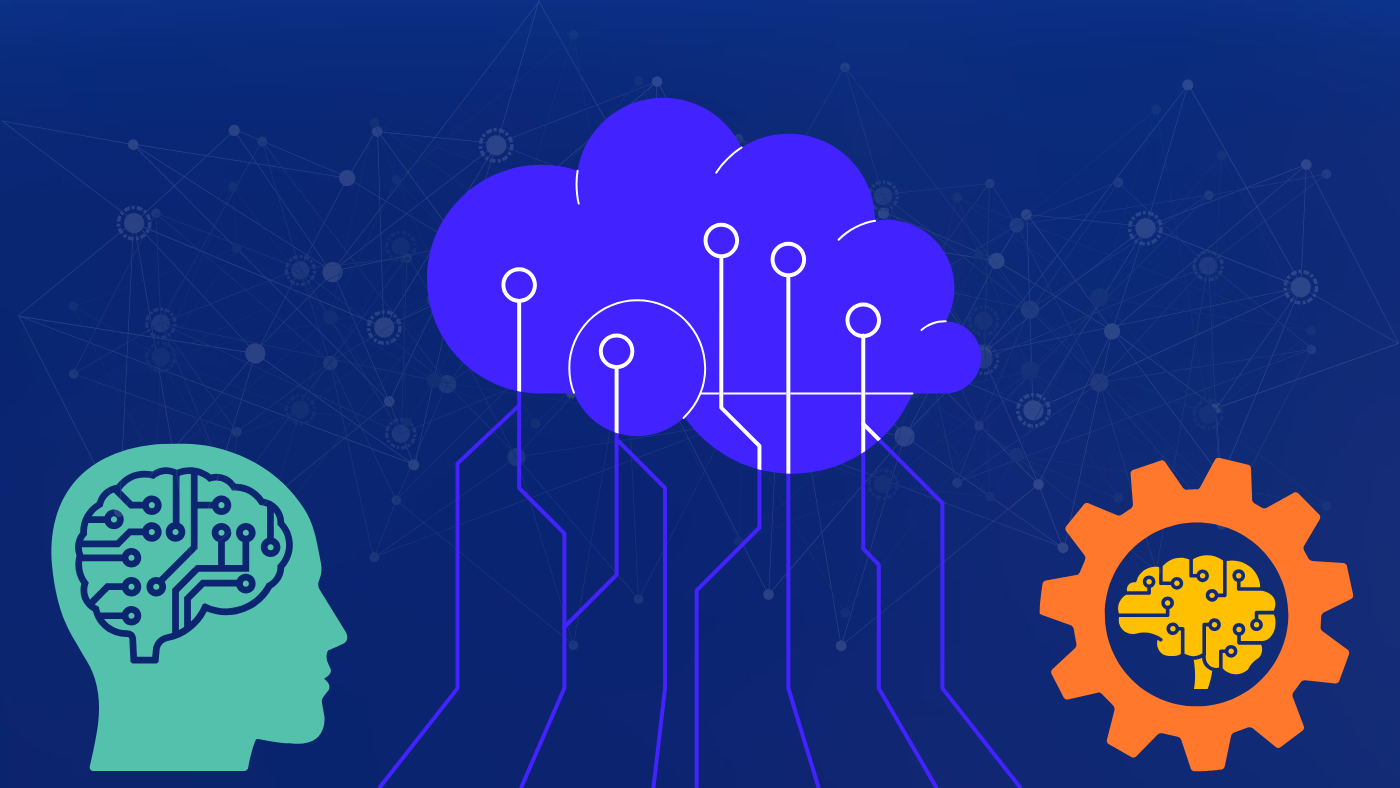
In the rapidly advancing field of artificial intelligence (AI), effectively operationalizing machine learning (ML) models is vital for organizations that want to fully harness the capabilities of data-driven decision-making. Two significant paradigms have emerged to meet this need: Machine Learning Operations (MLOps) and Large Language Model Operations (LLMOps). MLOps focuses on the comprehensive deployment and maintenance of ML models, while LLMOps is specifically designed to tackle the unique challenges presented by large language models (LLMs). This article rigorously explores the definitions and distinctions between these paradigms, delves into the inherent challenges of LLMOps, proposes actionable solutions, and outlines the critical lifecycle management of LLMOps.
Introduction to MLOps and LLMOps
MLOps is a powerful set of practices that ensures the reliable and efficient deployment and maintenance of machine learning models in production. By integrating machine learning, DevOps, and data engineering, MLOps streamlines the entire ML lifecycle—from model development to deployment and ongoing monitoring. Its primary mission is to effectively bridge the gap between development and operations, guaranteeing that ML models are not only reproducible and scalable but also highly maintainable.
On the other hand, LLMOps is an essential subset of MLOps that specializes in the operationalization of large language models (LLMs). Models like OpenAI's GPT series stand out due to their immense number of parameters and extensive training data, allowing them to excel at understanding and generating human-like text. LLMOps tackles the unique challenges of deploying, fine-tuning, and sustaining these models in production environments with expertise and precision.
Differences Between MLOps and LLMOps
While both MLOps and LLMOps focus on the operationalization of machine learning models, they differ in crucial ways:
- Model Complexity: Large Language Models (LLMs) are vastly more complex than standard machine learning models, often containing billions of parameters. This complexity demands specialized infrastructure and advanced optimization techniques to ensure optimal performance.
- Resource Requirements: Deploying LLMs requires considerable computational resources, including high-performance GPUs or TPUs, as well as enhanced memory and storage capacity.
- Latency and Throughput: LLMOps effectively addresses the challenges of inference latency and throughput, guaranteeing that LLMs can deliver real-time predictions without sacrificing performance.
- Data Management: LLMs rely on extensive training data, necessitating robust data pipelines and storage solutions to efficiently manage data ingestion, preprocessing, and augmentation.
- Ethical Considerations: Given their capacity to generate human-like text, LLMs create distinct ethical challenges, including the potential for biased or harmful content. It is imperative to integrate these considerations into the LLMOps framework to ensure responsible deployment.

Challenges with LLMOps
Operationalizing large language models (LLMs) poses significant challenges that organizations must decisively tackle to ensure efficient deployment and maintenance. These challenges arise from the immense scale of LLMs, their considerable computational requirements, and their substantial impact on users and businesses.
- Scalability stands out as a critical issue in LLMOps. Unlike traditional machine learning models, LLMs demand vast computational resources, often requiring thousands of GPUs or TPUs to operate simultaneously. As model sizes continue to grow, ensuring that infrastructure meets these increasing demands without compromising performance is essential. Organizations must strategically invest in high-performance computing environments and employ distributed computing techniques to make LLMs practical for real-world applications.
- Cost management is another vital concern. Training and deploying LLMs can be prohibitively expensive due to the need for powerful hardware, high energy consumption, and significant data storage. Many companies are finding success by optimizing resource usage while maintaining model accuracy and performance. Implementing strategies such as model compression, quantization, and fine-tuning smaller, domain-specific LLMs is crucial for making large-scale AI models financially sustainable.
- Monitoring and maintenance are imperative to ensure that LLMs perform reliably in production. Unlike traditional software, LLMs can experience model drift, where performance declines over time due to changes in input data distributions. Moreover, identifying and mitigating unintended behaviors, biases, and hallucinations—where the model generates incorrect or misleading outputs—are essential tasks. Employing advanced monitoring tools that track real-time performance metrics, flag anomalies, and support continuous retraining is necessary to uphold model integrity.
- Security and compliance must be prioritized due to the extensive amounts of data used for training LLMs. Safeguarding sensitive and proprietary data is paramount, especially when handling personally identifiable information (PII) or confidential business data. Additionally, adherence to global regulations like GDPR and CCPA is not optional but a priority. Organizations need to implement robust encryption methods, access controls, and audit trails to safeguard their LLM pipelines effectively.
- User trust and transparency are foundational to the successful adoption of LLM-powered applications. Given that LLMs can generate human-like text, it is vital that their responses are accurate, unbiased, and interpretable. Users frequently struggle to grasp how these models derive their outputs, leading to skepticism regarding misinformation. By establishing explainability features, bias mitigation techniques, and clear usage guidelines, organizations can build confidence in LLM-based systems, enhancing their reliability and ethical standing.
Confronting these challenges demands a proactive approach that combines advanced technologies, strategic planning, and rigorous oversight. As LLMOps continues to evolve, organizations must adopt best practices that optimize efficiency, mitigate risks, and bolster the reliability of large-scale AI models.
Solutions to LLMOps Challenges
Addressing the challenges of LLMOps (Large Language Model Operations) requires a combination of technological advancements, strategic planning, and continuous monitoring. By implementing the right strategies, organizations can optimize the deployment, maintenance, and performance of large language models while ensuring cost efficiency, security, and reliability.
Infrastructure Optimization
One of the most effective ways to manage the computational demands of LLMs is through infrastructure optimization. Utilizing distributed computing allows organizations to divide the workload across multiple GPUs, TPUs, or cloud instances, reducing bottlenecks and improving efficiency. Model parallelism—where different parts of the model run on separate hardware units—can further enhance performance by ensuring that large models can be processed without overwhelming individual components. Additionally, leveraging hardware accelerators, such as NVIDIA TensorRT and TPUs, can significantly speed up inference while reducing energy consumption.
Cost-Effective Practices
Given the high operational costs of LLMs, implementing cost-effective practices is essential. Techniques such as model pruning help reduce the number of parameters in a model without significantly affecting its performance, thus lowering computational and storage costs. Quantization, which converts high-precision model weights into lower-bit representations, can also minimize memory and processing requirements. Moreover, utilizing cloud-based solutions allows businesses to scale resources on demand, rather than investing in expensive on-premise infrastructure. Many cloud providers offer specialized AI hardware at optimized pricing, making it more feasible for companies to run LLMs efficiently.
Advanced Monitoring
Maintaining the integrity of LLMs in production requires robust monitoring and maintenance strategies. Real-time monitoring systems track model performance metrics, latency, and resource usage to ensure smooth operations. These systems can detect anomalies, such as sudden drops in accuracy or biased outputs, allowing teams to intervene promptly. Automated alert mechanisms can flag potential issues, such as model drift, and trigger retraining processes. Organizations can also use tools like Prometheus, Grafana, and MLFlow to log, visualize, and continuously analyze model behavior.
Robust Security Measures
Security and compliance are critical concerns when working with large language models, especially those handling sensitive data. Implementing robust security measures ensures data privacy, prevents unauthorized access, and mitigates risks associated with data breaches. Encryption protocols should be applied to both data storage and communication between AI services. Enforcing strict access controls helps prevent unauthorized modifications to models, datasets, or system configurations. Additionally, conducting regular security audits ensures that vulnerabilities are identified and patched before they can be exploited. Compliance with global regulations, such as GDPR (General Data Protection Regulation) and CCPA (California Consumer Privacy Act), should also be a priority for organizations handling user data.
Enhancing Transparency
Building user trust in AI-driven applications requires transparency and explainability. Since LLMs often function as "black boxes," where their decision-making processes are not easily interpretable, incorporating explainable AI (XAI) techniques can help make their outputs more understandable. Tools like SHAP (SHapley Additive Explanations) and LIME (Local Interpretable Model-agnostic Explanations) can explain why a model generates specific outputs. Conducting bias assessments ensures that models do not produce harmful or skewed results, promoting the ethical use of AI. Additionally, organizations should maintain clear documentation on how models are trained, tested, and validated to foster accountability and trust among users.
LLMOps Lifecycle Management
The LLMOps lifecycle consists of several key stages, each crucial for ensuring the efficiency, reliability, and continuous improvement of large language models (LLMs).
- Data Collection and Preparation: The foundation of any LLM is high-quality data. Organizations must gather extensive datasets from a variety of sources, including text corpora, structured databases, and real-world interactions. Ensuring data quality, accuracy, and diversity is essential to prevent biases and improve generalization. After collection, the data undergoes preprocessing steps, such as cleaning, deduplication, and normalization. Data augmentation techniques can further enhance training samples, thereby improving the model's robustness. Additionally, anonymization and compliance with privacy regulations (e.g., GDPR, CCPA) are necessary to protect sensitive information.
- Model Training and Fine-Tuning: Training LLMs requires substantial computational resources, often distributed across cloud-based or on-premises GPU/TPU clusters. Organizations typically start with pre-trained models (such as GPT or Llama) and fine-tune them for specific domain applications. Optimization techniques like quantization, mixed-precision training, and model distillation help enhance efficiency and reduce computational overhead. Hyperparameter tuning and continuous evaluation on benchmark datasets are vital to achieving optimal performance before deployment.
- Deployment: After training, the model needs to be deployed in a scalable and cost-effective environment. Organizations can choose between on-premises, cloud, or hybrid solutions based on performance and budget constraints. To optimize inference speed, techniques such as model pruning and tensor parallelism are employed to ensure low-latency responses. APIs and microservices facilitate integration into applications, while auto-scaling mechanisms dynamically adjust resources according to demand, ensuring consistent performance.
- Monitoring and Evaluation: Continuous monitoring is critical after deployment to track model performance, detect anomalies, and prevent degradation. Real-time monitoring tools like Prometheus, Grafana, and MLFlow enable organizations to analyze model behavior and resource usage. To maintain accuracy, mechanisms for detecting model drift and bias should be implemented, allowing for timely retraining. Incorporating explainability tools also helps provide insights into model decisions, enhancing transparency and trustworthiness.
- Feedback and Improvement: LLMs need to evolve based on real-world interactions. Collecting user feedback allows for iterative model enhancements, while human-in-the-loop (HITL) approaches enable expert intervention when necessary. Regular updates with new data and domain knowledge help keep the model relevant and effective. Conducting periodic security audits and compliance checks ensures ethical AI deployment while adhering to data protection laws.
By following a structured lifecycle, organizations can improve the reliability, scalability, and ethical deployment of LLMs, ensuring their models remain effective and trustworthy over time.
Conclusion
MLOps has been essential in streamlining machine learning operations, and LLMOps builds on this foundation to tackle the specific challenges associated with large language models (LLMs). These challenges include high computational demands, cost management, ethical considerations, and real-time monitoring. Unlike traditional machine learning models, LLMs require vast datasets, ongoing fine-tuning, and specialized infrastructure. To address these needs, advanced strategies such as model parallelism, quantization, and explainability techniques are necessary to ensure efficiency and reliability.
By implementing effective LLMOps practices, organizations can fully leverage the capabilities of LLMs for various applications, including AI-driven chatbots, content automation, and real-time analytics. This approach supports scalability, transparency, and adherence to ethical AI standards. As the adoption of AI continues to grow, businesses that invest in best practices for LLMOps will gain a competitive advantage, enabling sustainable, efficient, and responsible innovation in AI.
SHARE THIS
Discover More Articles
Explore a curated collection of in-depth articles covering the latest advancements, insights, and trends in AI, MLOps, governance, and more. Stay informed with expert analyses, thought leadership, and actionable knowledge to drive innovation in your field.
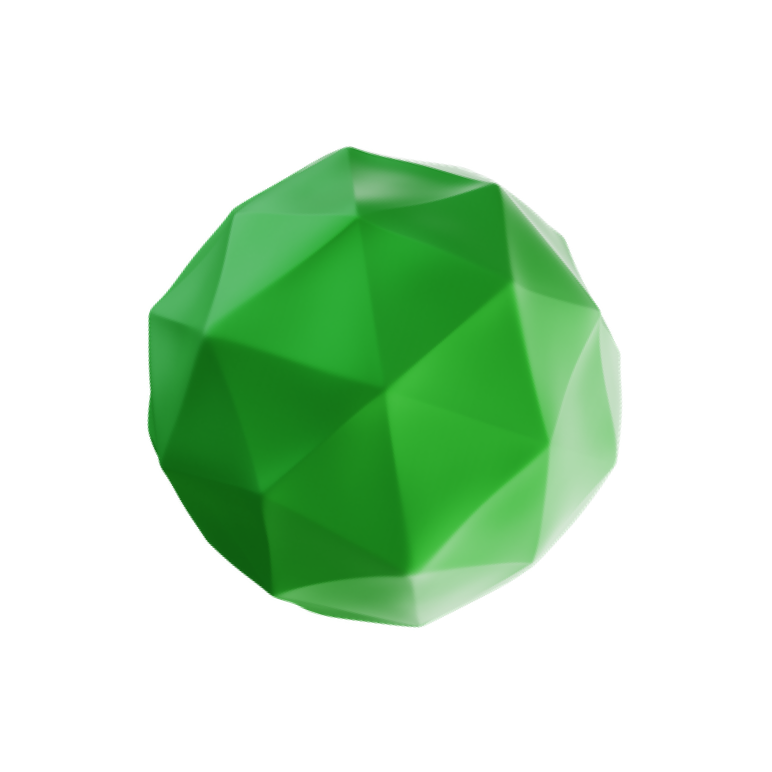
Is Explainability critical for your AI solutions?
Schedule a demo with our team to understand how AryaXAI can make your mission-critical 'AI' acceptable and aligned with all your stakeholders.