On-demand workshop: ML Observability + Deep Learning in Fraud Monitoring
November 1, 2022
Tackling fraud is and has always been an elusive challenge for the BFSI industry. Proactively detecting fraud before claims are settled and upgrading AI capabilities are currently one of the enterprise's main priorities in dealing with fraud. However, even the most mature organizations face significant challenges in identifying, researching and stopping fraud.
Most of the current fraud monitoring systems are largely ‘Rule’ based or handcrafted trigger-based systems and need manual experts to identify the triggers and configure them from time to time. They are usually do catching up rather than stay ahead of the curve. Then few use ML models to identify suspicious transactions. In both cases, evidence provided along with prediction can matter a lot on how well the predictions can be used. And performance during testing doesn't guarantee similar SLAs in production.
Using the ML Observability framework can drastically improve the usable of ML models for fraud monitoring and provide critical information on data drifts and model drifts.
Workshop Agenda:
Understand how advanced ML techniques (like Deep Learning) can be used as an additional line of defense against fraud so that you can focus only on the highest-value, highest-probability cases of fraud. And how to use ML Observability to make them acceptable, extract more value and ensure the models are consistent in production.
- Rule based (triggers) vs AI/ML in Fraud Monitoring.
- Introduction to AryaXAI - ML Observability framework.
- Additional explainability for ML models in fraud monitoring.
- Deliverables of ML Observability platform during production.
- Case study walk through: Using DL +AryaXAI Health Insurance Claims Fraud monitoring
Unlock More Videos
Access a wide range of videos covering the latest advancements, insights, and trends in AI, MLOps, governance, and more. Watch expert presentations, workshops, and deep dives to stay updated.
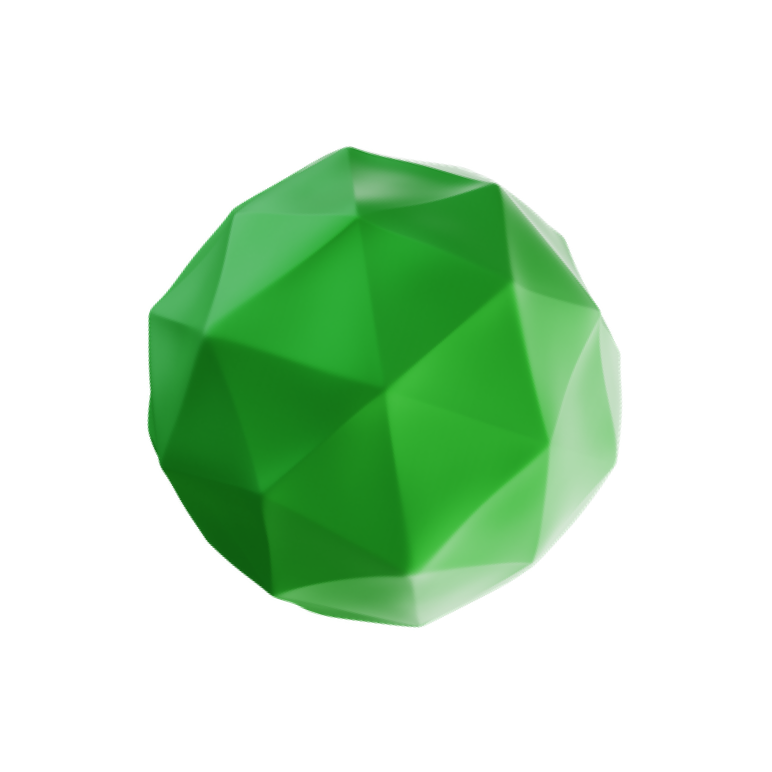
Is Explainability critical for your 'AI' solutions?
Schedule a demo with our team to understand how AryaXAI can make your mission-critical 'AI' acceptable and aligned with all your stakeholders.