On demand workshop: How to use ML Explainability to identify ‘more fraud’ and better recall rates in claims fraud monitoring
January 11, 2023
In our previous workshops, we discussed the overview schema of using ML Observability and Deep Learning in Claims Fraud Monitoring.
In this workshop, we focus primarily on the role of ML Explainability in successful fraud detection. Model Explainability is expected as a fundamental part of any AI solution. But it has a very crucial role in Fraud detection. While the AI/ML models can learn in-depth patterns and identify the fraud, without enough pieces of evidence to support the model prediction and explanations, the manual investigation may have needed directional feedback resulting in failed identification.
For example: In Health Insurance, let's say the model predicted ‘high-risk’, but there were no explanations provided. The investigator may investigate a different aspect of the profile resulting in the failed outcome, and the case may get accepted as ‘Genuine’. This is a very strong example of how AI should work with human experts and provides evidence and explanations that can help the experts to prove the fraud nature effectively and at scale.
We discuss various XAI methods that can be used for ‘Fraud Monitoring’ use case. We also go through AryaXAI case studies on claims fraud monitoring in Health Insurance. Watch the session to learn:
- Why do you need ML Explainability in Fraud Monitoring
- What are the different types of ML Explainability
- How do these support the human experts for investigation
- Case studies on using AryaXAI in Claim Fraud Monitoring
Unlock More Videos
Access a wide range of videos covering the latest advancements, insights, and trends in AI, MLOps, governance, and more. Watch expert presentations, workshops, and deep dives to stay updated.
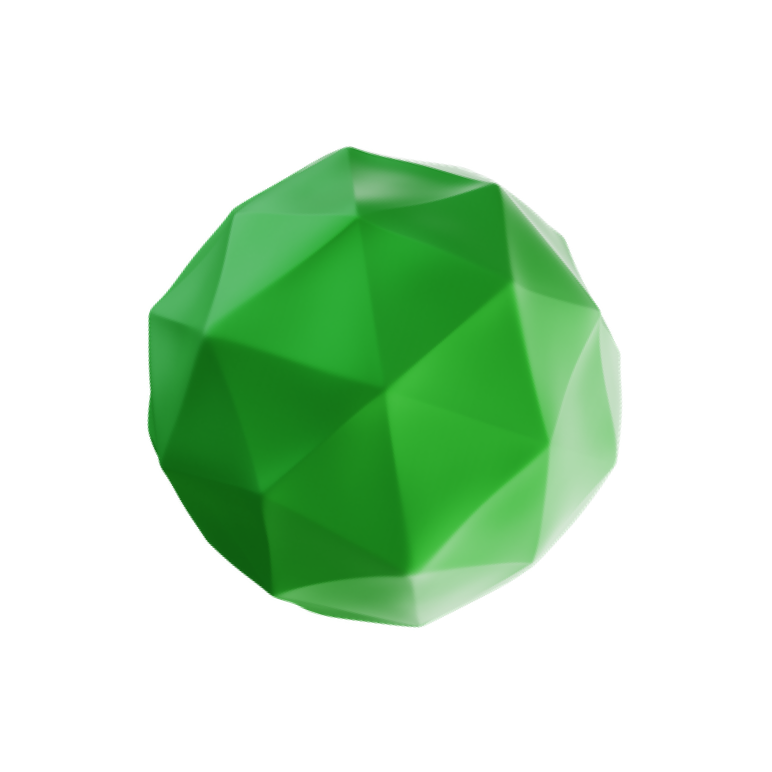
Is Explainability critical for your AI solutions?
Schedule a demo with our team to understand how AryaXAI can make your mission-critical 'AI' acceptable and aligned with all your stakeholders.