On-demand webinar: Reviewing the risks of AI Technical Debt (TD) in Financial Services Industries (FSIs)
October 23, 2024
Key Takeaways:
- A comprehensive review of AI Technical Debt (TD) in FSIs
- Deep dive on the critical TDs like Explainability Debt, Regulatory debt, auditability debt etc.
- Key insights into the risks associated with AI systems in financial services
Session Overview:
The session began with a paper presentation by Vinay Kumar, Founder and CEO of Arya.ai, based on his recently published paper titled Risks of AI Technical Debt in FSIs. In this presentation, Vinay outlined the various types of AI technical debt that pose risks to the financial services industry, emphasizing their impact on model performance and regulatory compliance. The discussion focused on categorizing various types of AI technical debt and reviewing empirical evidence of associated risks. Below is a summary of the core points:
- Data Inconsistencies Debt: Occurs when discrepancies between training and production data cause models to underperform. This leads to risks such as performance degradation and errors. Causes include non-standard data capturing and outdated legacy systems.
- Feature Omission Debt: Arises when critical features are excluded from models due to statistical or business oversight issues, reducing accuracy and increasing bias. This is particularly problematic in high-stakes domains like loan underwriting.
- Over-Engineering Debt: Occurs when raw data features are overly simplified or complicated, hindering model learning and explainability. This debt complicates compliance and risk management.
- Insufficient Testing Debt: Involves inadequate testing of models across real-world scenarios, risking unreliable and non-compliant models in FSIs. Causes include poor test data selection and outdated testing strategies.
- Explainability Debt: Relates to the inability to explain or misunderstand ML model decisions, threatening transparency and compliance in FSIs. This debt is exacerbated by unstable post-hoc explanation techniques and ad hoc resolution heuristics.
- Drift Monitoring Debt: Occurs when there is inadequate monitoring of data and model drift during production. It increases the risk of models becoming obsolete, especially in evolving financial environments.
- Bias/Fairness Debt: Arises from deploying biased models, leading to discriminatory outcomes and reputational damage. The absence of standardized bias metrics and evolving regulations like the EU AI Act adds to the challenge.
- Auditability Debt: Results from deploying models without appropriate audit frameworks, leading to unchecked biases and regulatory compliance risks.
- Model Attack Debt: Occurs when vulnerabilities, like adversarial attacks or model poisoning, are not addressed, threatening the integrity and security of AI systems.
- Shadowy Pre-Trained Models Debt: Refers to using undocumented pre-trained models, leading to compliance risks, especially in data privacy regulations.
- Delayed or Unchecked Feedback Debt: Arises when feedback on model performance is delayed or unchecked, leading to model degradation and poor decision-making.
- Reproducibility Debt: Occurs when results of models cannot be consistently replicated, undermining stability in risk management.
- Compliance & Governance Debt: Stems from the misalignment between fast-evolving AI technologies and regulatory frameworks, leading to potential legal repercussions for FSIs.
- Stakeholder Debt: Occurs due to limited participation in AI development, resulting in a lack of transparency and misaligned system effectiveness.
Vinay also provided empirical evidence to illustrate how these debts can manifest in real-world scenarios. These technical debts underscore the importance of addressing AI's complex challenges in FSIs to ensure compliance, transparency, and robust performance.
During the Q&A session, a query from the audience focused on existing regulations in India that could apply to AI. Vinay responded by highlighting the Model Risk Management guidelines from the Reserve Bank of India (RBI), which are applicable to any model used for credit risk management.
Following the presentation, an expert panel discussion between Vinay and Alban Bellenger, Head of Strategy at Aurionpro, delved deeper into the implications of AI technical debt in financial institutions. The session concluded with more audience questions and insightful responses, underscoring the importance of proactive risk management in AI systems.
Meet our Speakers:
Vinay Kumar, CEO & Founder of Arya.ai
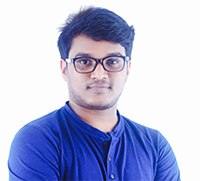
Vinay Kumar is the CEO & Founder of Arya.ai and heads R&D/Product for AryaXAI. He did his M.Tech and B.Tech from IIT Bombay with research on Deep Learning. He started Arya.ai in 2013 from IIT Bombay. Vinay was listed in Forbes 30 under 30, presented multiple tech talks in Nvidia GTC (SF), ReWork (SF & London), TEDx etc. He authored a patent on explainable 'AI' and published multiple papers on model explainability and alignment.
Alban Bellenger, Head of Strategy at Aurionpro
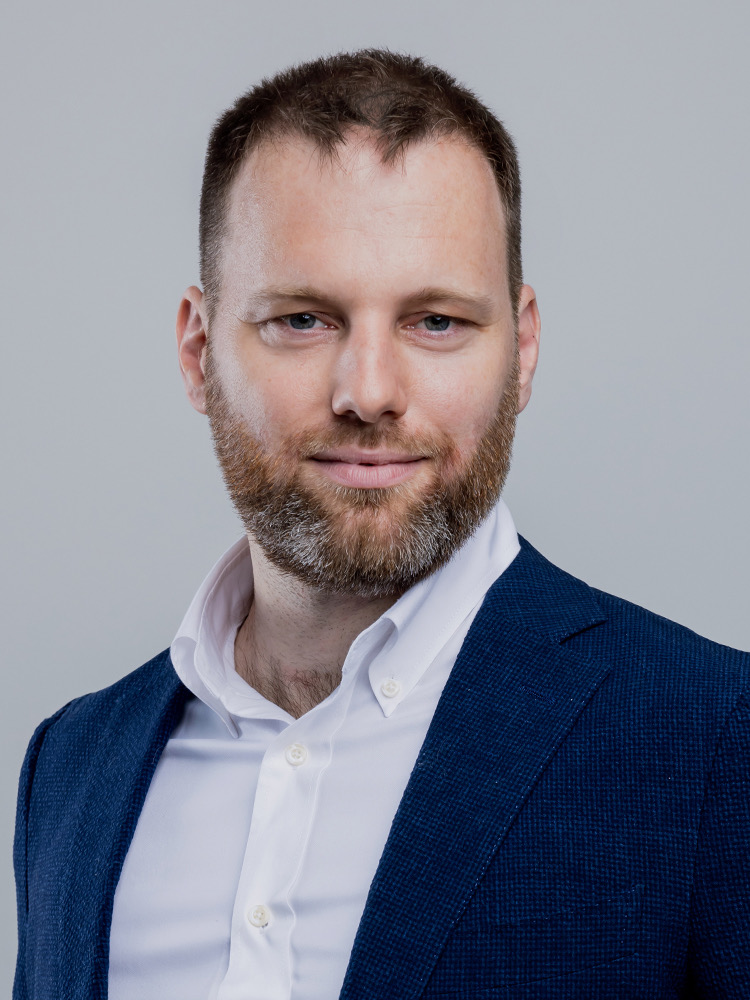
Alban Bellenger is the Head of Strategy at Aurionpro. With over 16 years of experience in the financial technologies sector, he has a proven track record of executing strategies for enterprise software. He currently oversees Aurionpro's strategy across multiple verticals such as AI, banking software, payments, and smart mobility.Before joining Aurionpro, Alban served as Director and Head of Strategy for Capital Markets Sales at FIS Global in Singapore, where he led transformative initiatives for the sales organization.
Unlock More Videos
Access a wide range of videos covering the latest advancements, insights, and trends in AI, MLOps, governance, and more. Watch expert presentations, workshops, and deep dives to stay updated.
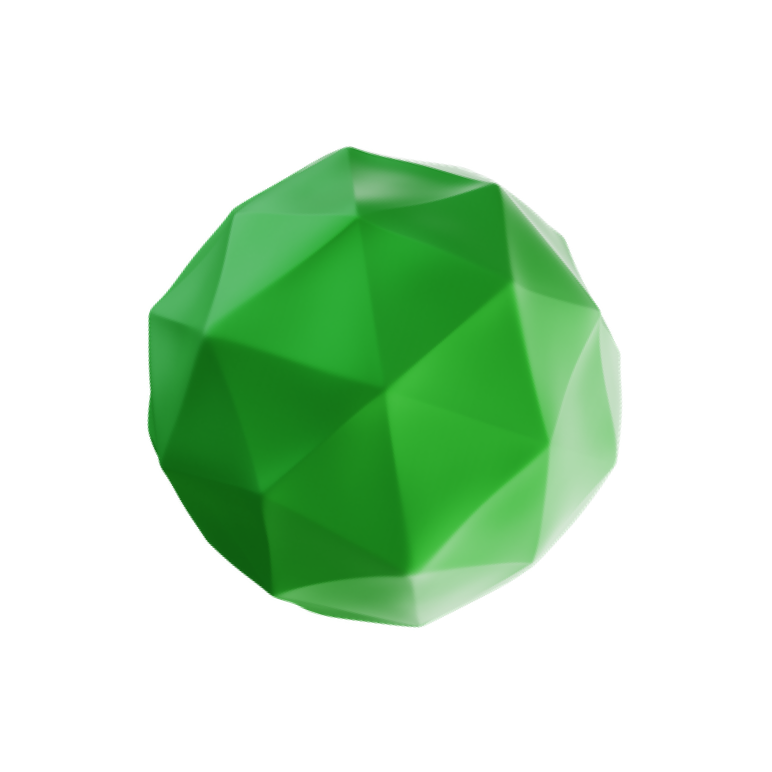
Is Explainability critical for your 'AI' solutions?
Schedule a demo with our team to understand how AryaXAI can make your mission-critical 'AI' acceptable and aligned with all your stakeholders.