AryaXAI Synthetics: Using synthetic ‘AI’ to compliment ‘ML Observability’
March 14, 2023
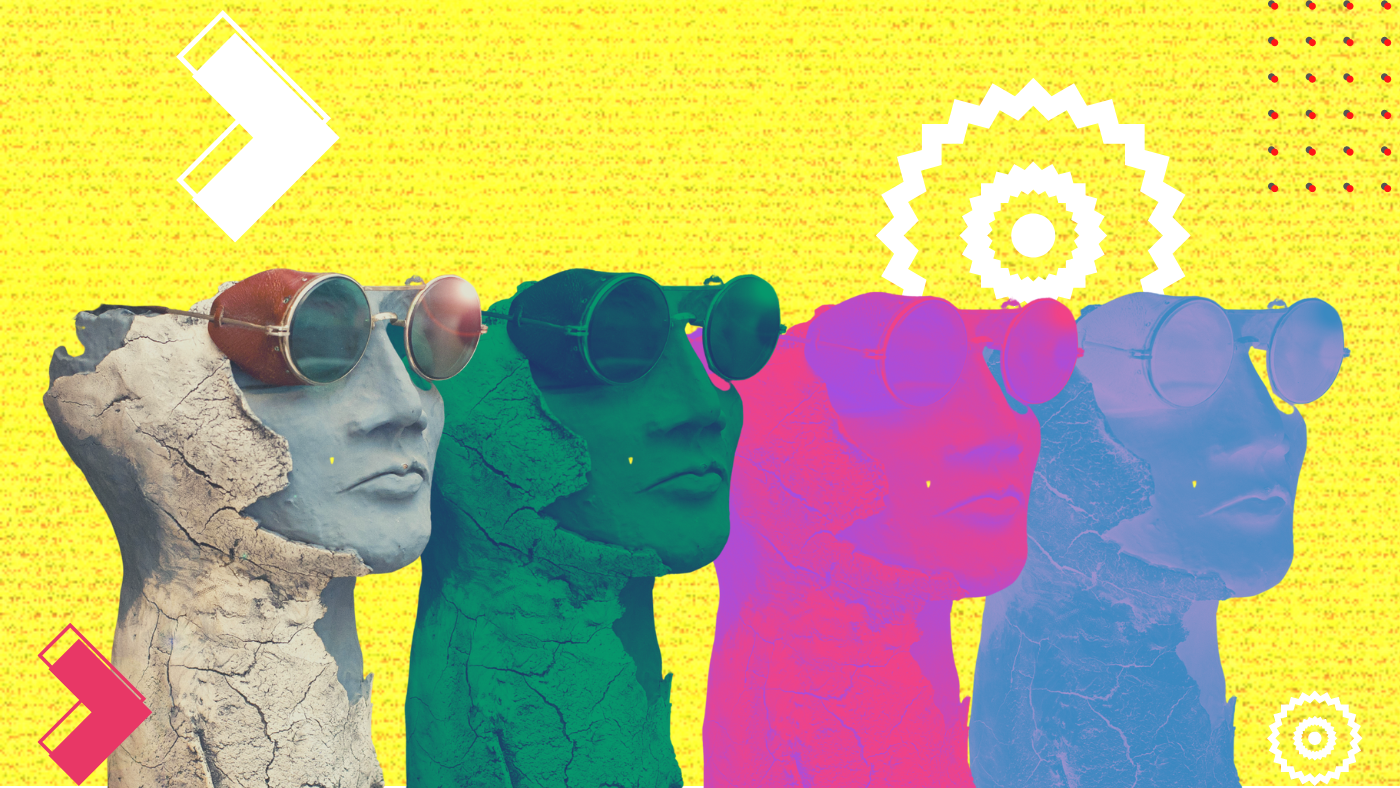
A good ML observability should not only identify the source of the error but also provide the context behind the error and its resolution.1
Data scientists and researchers spend tremendous effort in building models and making them ready to use. Data becomes the critical source of information for these models to be effective and functional. This is the dependent variable based on which all the model predictions are formed - if this goes wrong, the ML model goes downhill.
Such gaps in the data can create multiple challenges during training and in production. Let’s take a look at some of the challenges:
Data Privacy:
Based on geography, data privacy laws are clearly defined on limiting or restricting the usage of PII and other critical/ sensitive data. While access to these crucial data points can improve model performance, such strict regulations often restrict ML engineers and data scientists from working with real customer data. Exposing these sensitive data can risk leakage. Alternatively, skipping these features might hinder model performance, preventing it from making well-rounded, informed and novel predictions.
Data Skewness:
Class imbalance affects the model performance. If there are any imbalances in the data, they must be corrected before pushing it for processing. But, collecting real-world data for every plausible scenario and rare case on extreme values is simply not possible. To ensure that the ML model's capabilities are not affected, skewed data needs severe modifications.
Biased Models:
Sensitive classes need to be protected from any societal or selection bias in the models. This may also be needed as part of the regulations. If there are any inherent biases in the training data, the model learns these patterns and applies them to its predictions.
Model Risk Assessment:
Failure of AI/ML production can be critical to the business. So, it is necessary to stress test the models on various scenarios to ensure the gaps in the models are highlighted. Such identified gaps can be resolved through constructive feedback to the model or through ‘policies/ guardrails’. Brainstorming and testing the model on multiple scenarios will validate the model to anticipate risks better, reducing the harm to customers and other stakeholders. Generating such samples is tough and impossible for many use cases.
Introducing ‘AryaXAI Synthetics’:
Introducing ‘AryaXAI Synthetics’, now with issue resolution added to its already impressive issue identification capabilities.
‘General Adversarial Networks (GANs)’ has been a powerful technique to generate data like images/ text/ voice etc. But GANs can also be used to generate high-quality synthetic data sets. We are using a combination of GANs and statistical models to generate high-quality synthetic data. Users can use AryaXAI synthetics and resolve critical data gaps, test models at scale and preserve data privacy.
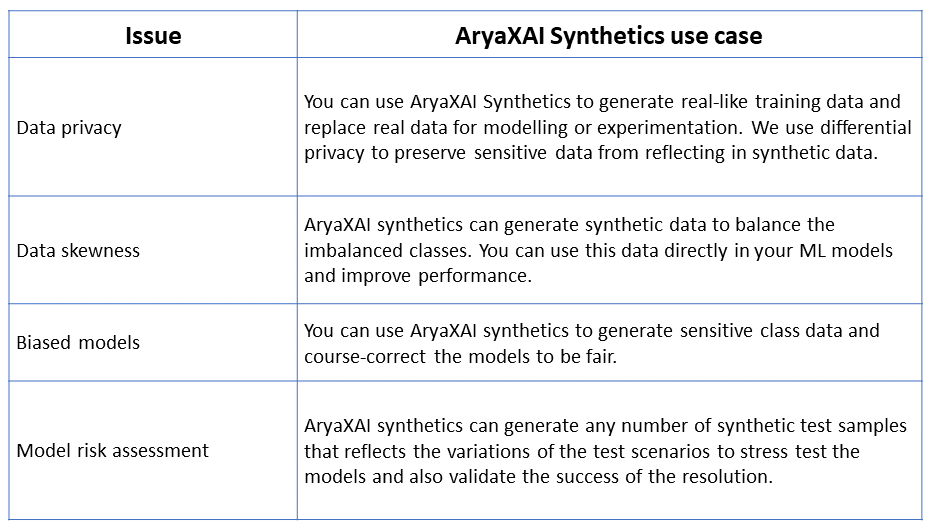
AryaXAI Synthetics case study on imbalance data:
For this case study, we are using the vehicle insurance fraud data from Kaggle2. Fraud detection is a classic example of data skewness. The use case is similar to any other transactional fraud monitoring use case. The challenge here is the data skewness. In this use case, genuine cases are “94%’, and Frauds are only “6%”.
In machine learning, there are various techniques to augment the imbalance class like Upsampling, Downsampling, SMOTE etc. While these methods can improve performance, sampling using these techniques can result in overfitting of the model. Generating synthetic samples that can make the model learn these less dense patterns without overfitting can improve recall rates.

Using AryaXAI Synthetics, we created a synthetic AI model and used it to generate synthetic data. We evaluated the model with the statistical correlation of ‘Real’ & ‘Fake’ data and ML Modelling.
Post training the GANs, we generated only synthetic frauds of various sample sizes to test the best class distribution for improved model performance, We also tested the quality of synthetic data using ML modelling methods (LR and XGBoost) and drift monitoring using AryaXAI.
Results from ML Modelling:
In order to test the ML models, we randomly selected an out of sample data in addition to test data that is defined while modelling.
Performance on real data (as-is)

Using AryaXAI Synthetics:
We built three models using different compositions of real data -
Test 1 - Balancing the classes: Added enough synthetic fraud data to balance with the genuine data.
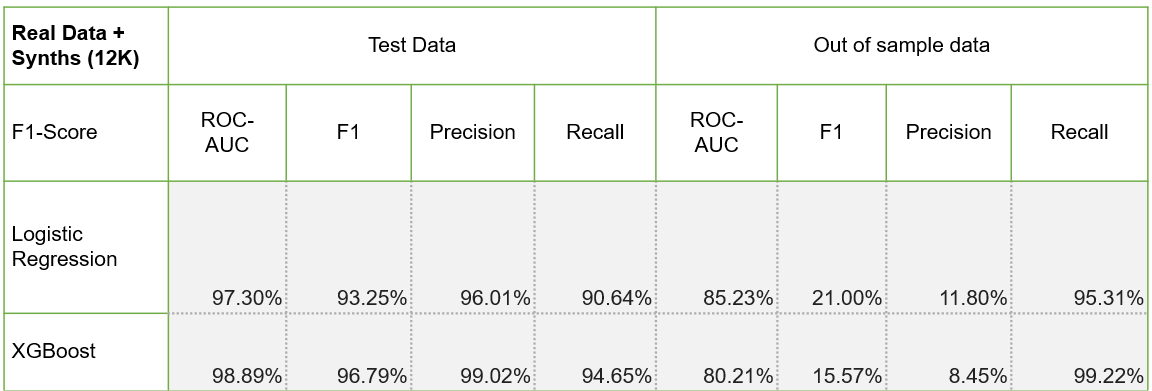
Test 2 - More frauds: Added more frauds (~3.5x)
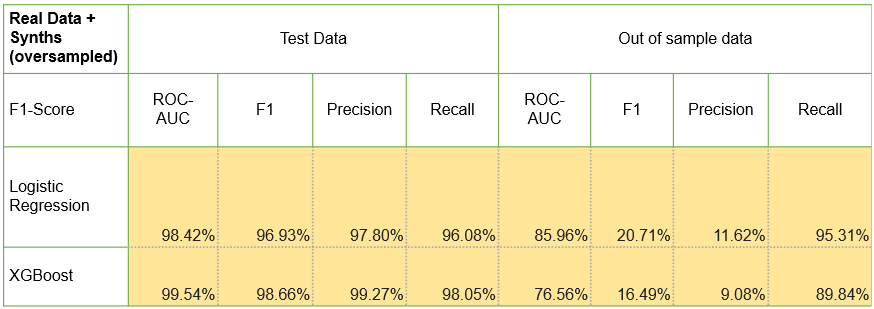
Test 3 - Only Synthetic Data: used only synthetic data without any real data. The dataset has both Fraud and Genuine in an almost equal distribution.

Validating statistically:
Using Data Drift Monitoring:
We performed data drift using AryaXAI. We used PSI as it had both categorical and numerical features. The average PSI for the top 10 columns is 0.055. A snapshot of the ML monitoring dashboard is attached below. A PSI of 0.35 is observed in the DayOfWeekClaimed variable, and the rest of them have PSI below 0.2.
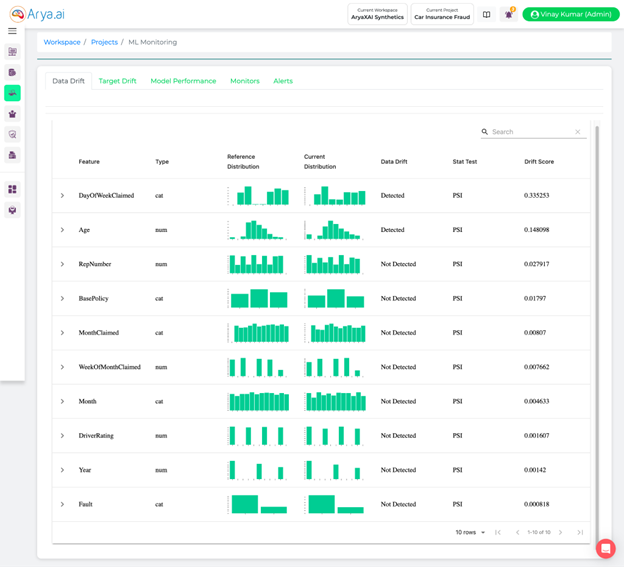
Comparing the datasets:
We compared Real and Synthetic data statistically, and the synthetic data can capture 86% of column shapes and 76% of column pair trends very well.
Overall Quality Score: 80.69%
Properties:
- Column Shapes: 85.66%
- Column Pair Trends: 75.72%
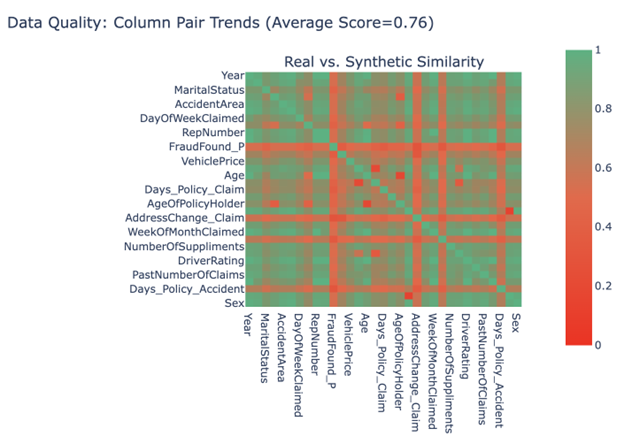
Conclusion:
AryaXAI, as an ML Observability platform, can not only help users in issue tracking but also issue resolution with ‘Synthetic AI’. It can be used to create high-quality synthetic data to solve data skewness, preserve data privacy and stress test models. In the case study, we discussed how AryaXAI Synthetics created synthetic data for a highly imbalanced data set of car fraud detection from Kaggle. By using synthetic data, the ML models had a better recall rate in out of sample testing using both LR and XGBMs (in Test 2). In an experimental approach (Test 3) can even match the performance of real data without using any real samples.
Users can access the synthetic datasets under the ‘AryaXAI Synthetics’ workspace. This is currently open for all users in AryaXAI.
Note: AryaXAI is currently an invite-only platform. You can request an invitation here.
SHARE THIS
Discover More Articles
Explore a curated collection of in-depth articles covering the latest advancements, insights, and trends in AI, MLOps, governance, and more. Stay informed with expert analyses, thought leadership, and actionable knowledge to drive innovation in your field.
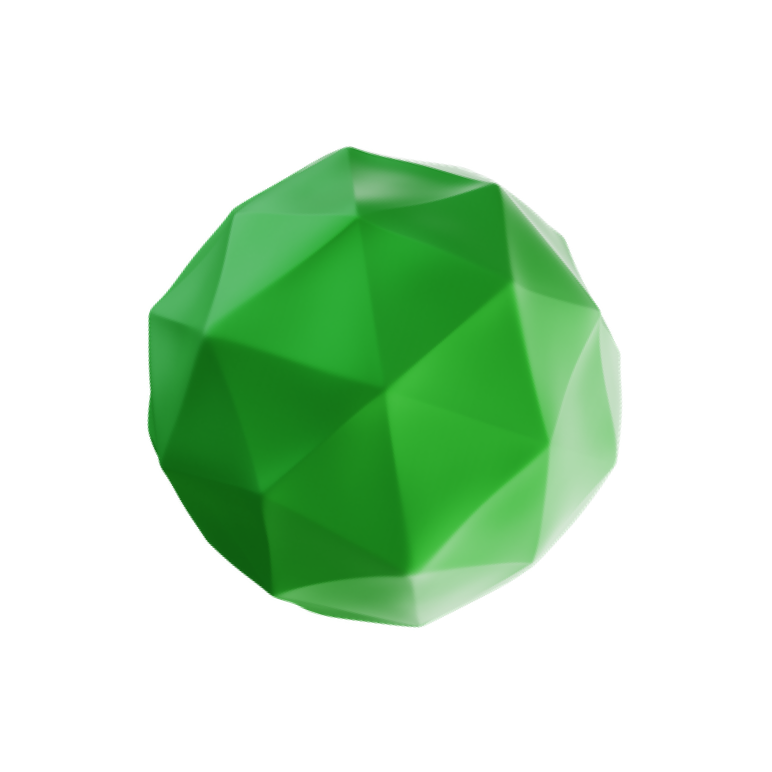
Is Explainability critical for your AI solutions?
Schedule a demo with our team to understand how AryaXAI can make your mission-critical 'AI' acceptable and aligned with all your stakeholders.