The Growing Importance of Explainable AI (XAI) in AI Systems
10 minutes
February 10, 2025

As artificial intelligence (AI) becomes increasingly integrated into everyday applications, the demand for Explainable AI (XAI) has grown exponentially. Explainable AI (XAI) is not only a means of enhancing user trust but also serves as a crucial competitive differentiator in an AI-driven market.
However, as highlighted in the latest research, ‘Bridging the Gap in XAI—The Need for Reliable Metrics in Explainability and Compliance’, the lack of standardized and reliable metrics in XAI diminishes its practical value, trustworthiness, and ability to meet regulatory requirements.
This blog post delves into best practices for integrating explainability into AI systems, highlighting its importance in reducing failures, improving user adoption, and overcoming technical and organizational challenges.
Enhancing AI Adoption Through Explainability
User-Centric Design for AI Explainability
Integrating explainability early in the AI development process is essential to foster user adoption. Engaging users from the beginning—through design workshops, focus groups, and user research—helps capture their expectations and understanding levels when interacting with AI outputs. By considering user needs and mental models during the design phase, organizations can tailor explanations to be intuitive, ensuring users can easily interpret AI outcomes. This personalized approach not only enhances user trust but also contributes to better decision-making by users.
Clear and Contextually Relevant AI Explanations
Providing transparent, contextually relevant explanations is vital for improving user comprehension. Employing interpretable models, such as decision trees or rule-based systems, simplifies the AI decision-making process, allowing users to visualize how outputs are generated. These transparent models enable users to understand the rationale behind decisions, reducing skepticism around AI. Feedback mechanisms also play a crucial role—by actively refining explanations based on user input, companies can continuously improve clarity and ensure the relevance of explanations in varied contexts.
Explainable AI as a Competitive Advantage
Why AI Explainability is a Market Differentiator
In today's hyper-competitive landscape, AI products that emphasize explainability stand out from the crowd. Companies operating in highly regulated industries like healthcare, finance, and insurance are increasingly turning to XAI to foster trust and transparency with their customers, regulators, and stakeholders. For example, when AI-driven decisions are explainable, organizations mitigate reputational risks and increase compliance with regulations.
Case Studies: XAI in Action
- Leading tech companies like Google, IBM, and Microsoft have successfully incorporated XAI into their products, helping users better understand machine learning predictions. This approach has proven especially beneficial in high-stakes industries where trust is crucial.
- In environments like healthcare, where AI decisions can directly affect patient care, providing clear and interpretable explanations empowers users—whether doctors or patients—to make informed decisions. The result? A stronger competitive edge, driven by enhanced accountability and improved user engagement.
- AryaXAI, an Explainable AI and Alignment platform, provides state-of-the-art components to explain and align mission-critical AI models. It is particularly valuable for high-risk AI applications where transparency, reliability, and accuracy are essential. By incorporating explainability at multiple levels, AryaXAI helps organizations deploy AI models that are not only high-performing but also interpretable and accountable.
How Explainable AI (XAI) Reduces Failures in High-Stakes AI Applications
Minimizing AI Bias and Errors with Explainability
The stakes of AI failure are high in mission-critical fields like healthcare, autonomous driving, or finance. Incorrect or biased decisions can lead to devastating consequences. XAI helps reduce the risk of these failures by shedding light on how AI models make predictions, providing invaluable insights into model behavior.
Accountability and Trust in AI Decision-Making
XAI enhances accountability by allowing organizations to trace decision-making processes back to their roots. This transparency helps identify the cause of errors, enabling corrective actions before issues reach the user or the public. It also establishes an environment where continuous improvement and rigorous testing are prioritized, ultimately improving the robustness of the AI system.
For instance, if an AI model in a self-driving car makes an unsafe decision, the ability to trace that decision and understand the underlying factors can prevent similar failures from happening in the future.
Compliance with AI regulations
AI regulations have emerged as one of the most pivotal drivers of the need for explainable AI. Across the globe, regulatory bodies are collaborating to establish frameworks that prioritize transparency and accountability in AI systems. Regulatory frameworks like the EU AI Act and GDPR mandate transparency in AI-driven decisions, particularly in high-risk sectors. Explainable AI ensures that organizations meet the regulatory requirements through interpretable and traceable decision-making processes.
Key AI Explainability techniques:
Several methods for achieving explainability are suited to different needs and model complexities.
1. Interpretable Models for AI Transparency:
These models, such as decision trees or rule-based systems, are inherently transparent and easy to interpret. They provide a direct explanation for their outputs, making them ideal for scenarios where understanding the reasoning behind the AI's decisions is critical.
2. Post-Hoc Explainability Methods
For complex, black-box models like deep neural networks, techniques like LIME (Local Interpretable Model-agnostic Explanations) or SHAP (SHapley Additive explanations) can offer insights into model predictions. These techniques help explain the behavior of models that are otherwise difficult to interpret, offering users a clearer view of how different features impact outcomes. However, as research dictates, post-hoc methods often suffer from inconsistencies and manipulation risks.
DL-Backtrace: A Model-Agnostic Explainability Technique
A notable advancement to tackle these challenges is DL-Backtrace, a model-agnostic explainability technique developed by AryaXAI. DL-Backtrace provides stable and accurate explanations for any deep learning model, addressing limitations of existing methods like LIME and SHAP. By offering a more reliable understanding of model decisions, it enhances transparency and trust in AI-driven outcomes. Learn more about DL-Backtrace here.
3. AI Visualizations for Model Interpretability
Graphical representations of data, such as heatmaps, saliency maps, and decision graphs, can help users quickly grasp model behavior and outcomes. Visualizations are particularly useful when working with complex data and models, as they simplify information and improve the interpretability of model decisions.
Each approach has its place in AI systems, depending on the context and application. The key is to choose the right combination of techniques to match the needs of the model and the target audience.
Overcoming Challenges in Implementing Explainable AI
While integrating explainability into AI solutions is crucial, it comes with its own set of challenges, both technical and organizational.
Balancing AI Performance with Explainability
One of the primary challenges is balancing model performance with interpretability. Many high-performance models, particularly deep learning systems, are inherently opaque. Developing models that are both powerful and interpretable requires significant investment in research and development. As AI technologies evolve, companies must invest in innovative approaches, such as hybrid models that combine the power of deep learning with interpretable layers, to ensure that explainability does not compromise performance. Research also shows that developing context-sensitive, manipulation-resistant evaluation metrics help ensure explainability methods genuinely reflect model behavior.
Securing Organizational Buy-In for AI Transparency
Getting support from all relevant stakeholders is key to the successful implementation of XAI. Such successful implementation involves educating business leaders, technical experts, and legal advisors about the benefits of explainability in AI. Establishing cross-functional governance teams that are aligned on the importance of transparency and accountable AI deployment is essential. These teams can drive strategic decisions and ensure that AI solutions meet both performance and explainability goals.
Building a Culture of AI Transparency
A culture of transparency begins with continuous education. Organizations should invest in training their teams—ranging from data scientists to business leaders—on the importance of explainability and how it can be integrated into their day-to-day workflows. When everyone involved understands the role of explainability, they can contribute more effectively to developing AI systems that prioritize user trust and accountability.
Conclusion: The Future of Explainable AI
Incorporating explainability into AI systems is not just about meeting regulatory requirements or gaining user trust—it's about creating responsible, transparent solutions that are accessible and reliable. As the role of AI in our lives continues to expand, prioritizing explainability will ensure that AI solutions are both ethical and effective.
As highlighted in the research, establishing reliable XAI evaluation standards is also essential to ensuring that AI systems meet technical and regulatory requirements while maintaining credibility and trust. By adopting best practices for explainability, organizations can set themselves apart in the marketplace, foster a culture of transparency, and reduce the risks associated with deploying AI in high-stakes environments.
The future of AI will depend on the trust it can garner from users, and explainability is the cornerstone of that trust.
Drive AI Performance with Explainability and Alignment
Enhance AI transparency and reliability with AryaXAI – the leading platform for explaining and aligning mission-critical AI models. Explore AryaXAI Today!
SHARE THIS
Discover More Articles
Explore a curated collection of in-depth articles covering the latest advancements, insights, and trends in AI, MLOps, governance, and more. Stay informed with expert analyses, thought leadership, and actionable knowledge to drive innovation in your field.
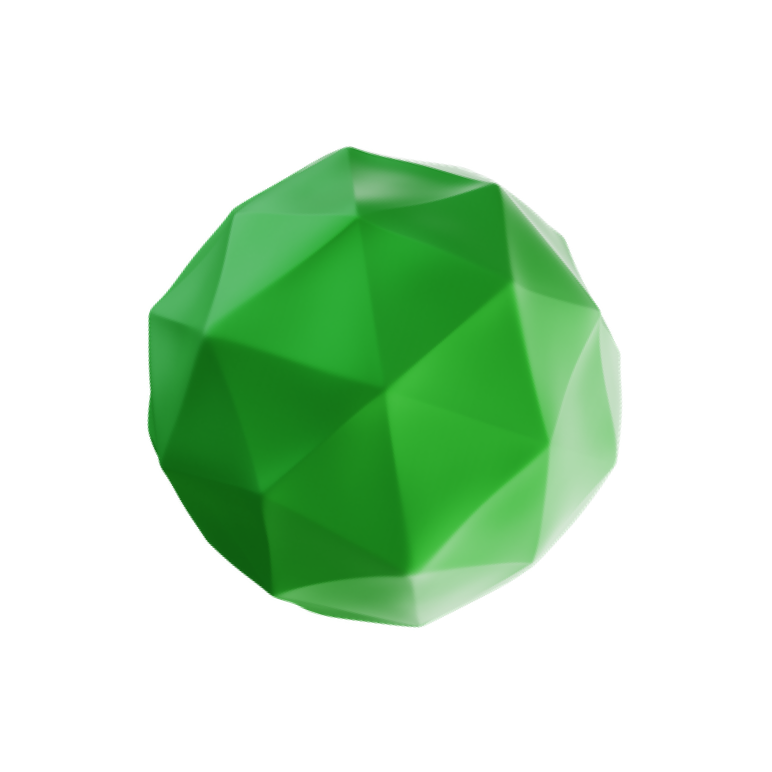
Is Explainability critical for your AI solutions?
Schedule a demo with our team to understand how AryaXAI can make your mission-critical 'AI' acceptable and aligned with all your stakeholders.