Managing AI Technical Debt in Financial Services: Why Explainability Matters
November 28, 2024
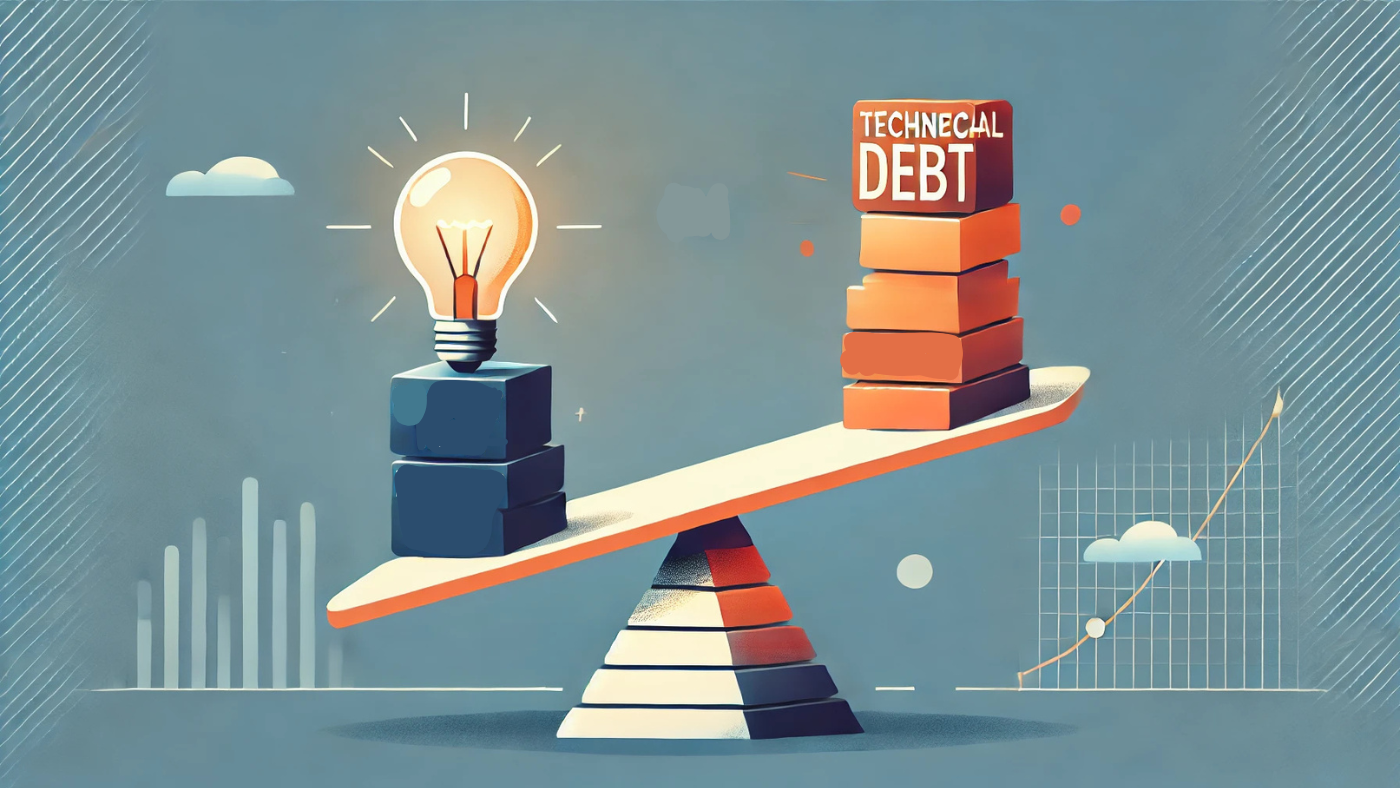
AI has transformed the Financial Services Industry (FSI), improving efficiency, innovation, and customer insights. However, FSIs face significant obstacles due to complex regulatory environments, data privacy concerns, and the growing challenge of AI Technical Debt (TD) — the cumulative cost and risk resulting from compromises, deferred maintenance, and the need for AI systems to adapt to rapid technological advancements.
Vinay Kumar, Founder and CEO of Arya.ai, highlights in his recent research that AI TD can stifle growth and introduce compliance risks. Addressing AI TD helps FSIs mitigate these risks, improve model reliability, and ensure long-term sustainability.
First, let us understand AI technical Debt:
As AI systems become complex, the costs associated with short-term technical compromises accumulate, leading to “Technical Debt.” In the context of AI, this debt encompasses the long-term operational costs and risks that arise from postponed tasks, design flaws, and hasty implementation decisions in AI models. Unlike traditional software TD, AI TD includes unique challenges such as algorithmic biases, data inconsistencies, model drift, and explainability gaps.
Explainability - Key driver in AI Technical Debt Management
Among the complexities contributing to AI TD, explainability has emerged as the key driver in managing and reducing this debt.
AI explainability refers to interpreting and understanding how AI models arrive at their decisions. In complex AI systems like deep learning and ensemble models, explainability tools help break down decision-making processes, clarifying which features or inputs drive specific outcomes. This is particularly crucial in heavily regulated sectors, such as Financial Services (FSIs), where explainability is essential for meeting strict requirements around transparency, fairness, and accountability, enabling stakeholders across the enterprise to trust, audit, and refine AI systems.
Explainability is vital for regulatory compliance and the cornerstone of managing AI TD. When stakeholders—from product managers to risk officers—understand how AI models function, they are better equipped to identify and mitigate biases, monitor model drift, and align AI outputs with business and compliance goals.
The Strategic Importance of Explainability for Key FSI Profiles
Explainability not only improves model performance but also enhances alignment across various departments within FSIs:
- Product Management: Explainability allows product teams to understand model outputs, ensuring new products with AI-driven features (like predictive analytics) meet customer needs and comply with regulatory standards.
- Risk and Compliance: Explainable AI models support regulatory adherence and audit preparation for risk and compliance teams By monitoring model decisions and identifying biases, these teams can validate fairness and mitigate fines under frameworks such as the EU AI Act and GDPR.
- AI/ML Teams: Explainability improves model monitoring and troubleshooting, helping AI/ML teams understand factors contributing to model drift and identify performance issues, reducing technical debt costs.
- Customer Experience (CX) Teams: Explainability enables CX teams to respond effectively to customer questions about AI decisions. Customer-friendly explanations help representatives clarify decisions, which can improve satisfaction and trust.
- CXOs and Executives: Explainability supports responsible AI governance at the executive level, empowering CXOs with insights into model performance, regulatory compliance, and areas for improvement. This approach ensures confidence when scaling AI across the organization.
Conclusion
To effectively manage AI TD in FSIs, enterprise clients should adopt a proactive approach incorporating best practices in AI governance and cross-disciplinary collaboration. Key strategies include:
- Implementing Governance Frameworks with Explainability at the Core: Establish standards for model transparency, ensuring compliance and facilitating collaboration with regulatory bodies.
- Continuous Model Monitoring and Drift Management: Use explainable AI tools to track model performance and identify drift, ensuring accuracy and alignment with evolving data patterns.
- Cross-Disciplinary Collaboration: Engage data scientists, risk managers, and regulatory experts to leverage explainability, reducing potential risks and ensuring AI systems are both compliant and aligned with business goals.
By embedding explainability into AI governance, enterprise clients in FSIs can proactively manage AI TD, ensuring a robust, compliant, and sustainable AI infrastructure. Explainable AI empowers FSIs to innovate confidently, maintain customer trust, and uphold a competitive edge in an AI-driven landscape.
SHARE THIS
Discover More Articles
Explore a curated collection of in-depth articles covering the latest advancements, insights, and trends in AI, MLOps, governance, and more. Stay informed with expert analyses, thought leadership, and actionable knowledge to drive innovation in your field.
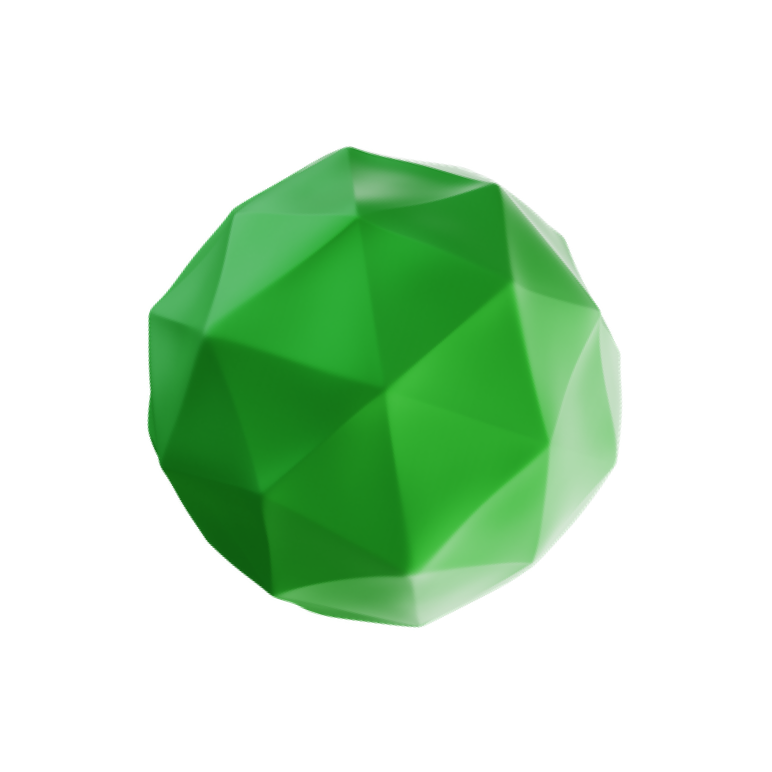
Is Explainability critical for your 'AI' solutions?
Schedule a demo with our team to understand how AryaXAI can make your mission-critical 'AI' acceptable and aligned with all your stakeholders.