Global Trends on AI Regulation: Transparent and Explainable AI at the Core
10 minutes
December 19, 2024
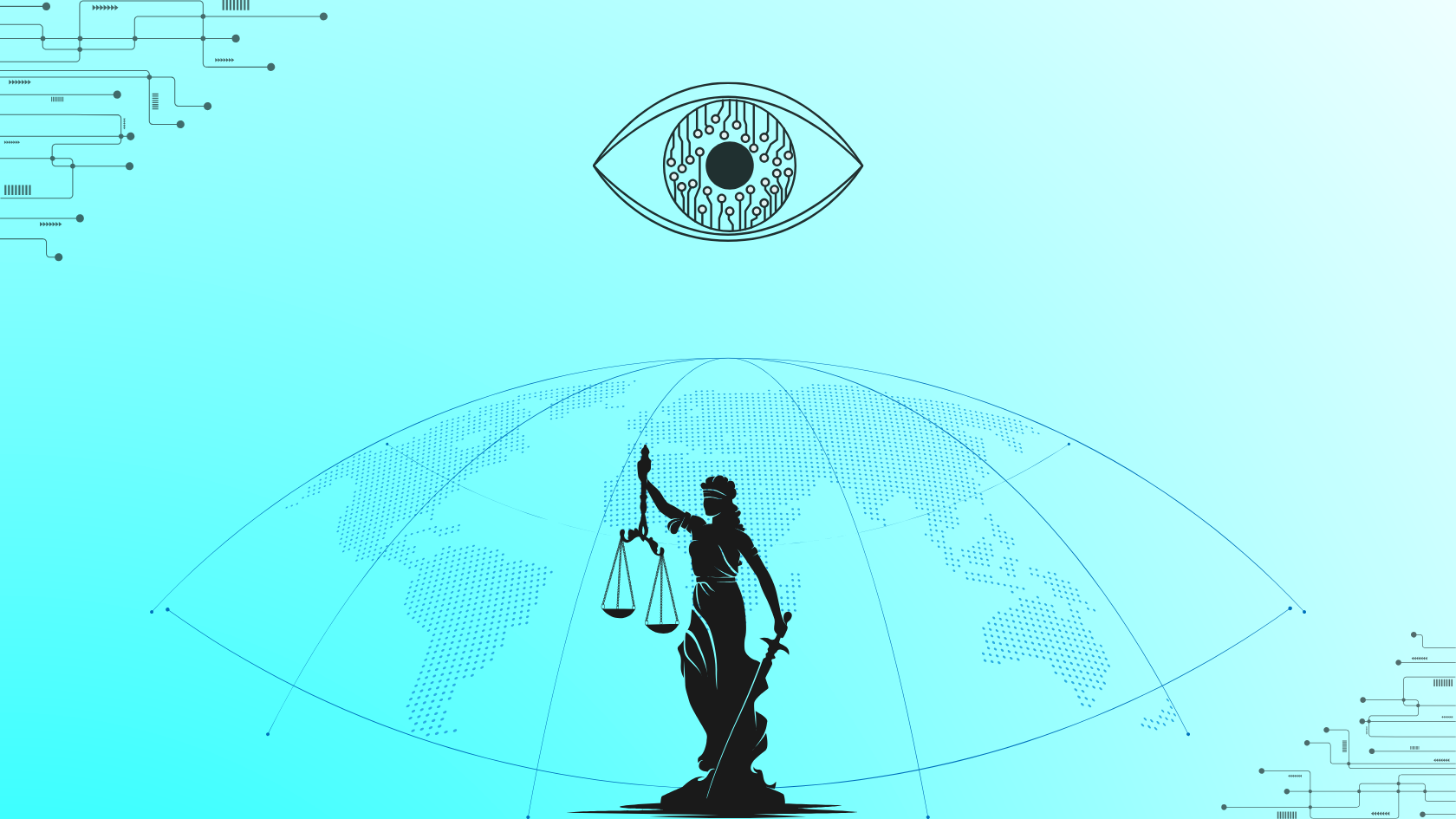
The accelerating capabilities of Artificial intelligence, especially in Generative AI (GenAI) and Large Language Models (LLMs), have pushed the need for AI regulations to the top of policymakers' agendas worldwide. The emphasis lies on fostering innovation while establishing safeguards to manage associated risks, with transparency and explainability emerging as key focus areas.
India
India has a pro-innovation approach to AI. The focus is on unlocking the full potential of AI while putting in place adequate guardrails to manage the associated risks.
In line with this, the Ministry of Electronics and Information Technology (Meity) published a blueprint for a new Digital India Act, which includes specific provisions for the regulation of high-risk AI systems. The blueprint calls for the definition and governance of these systems through legal and institutional quality testing frameworks.
The act emphasizes AI explainability as a key requirement for the regulation of high-risk AI systems in several ways:
- Algorithmic Transparency: AI models, especially those in high-risk scenarios, must be explainable to ensure accountability. This involves clearly describing how decisions are made to demonstrate fairness, reduce bias, and avoid harmful outcomes.
- Quality Testing Frameworks: Explainability will be part of the legal and institutional frameworks that test the reliability, robustness, and compliance of AI systems.
- Risk Mitigation for Zero-Day Threats: Explainable AI tools can help assess vulnerabilities and diagnose potential risks in AI systems, making them crucial for security and resilience.
- Justifications in Ad-Targeting & Content Moderation: Explainability ensures AI systems can provide clear, understandable reasons behind ad placement or flagged/moderated content to meet ethical and regulatory standards.
The planning commission NITI Aayog, in collaboration with the World Economic Forum, has published frameworks on Responsible AI (RAI). The latest paper in the series, “Responsible AI for All: Adopting the Framework – A use case approach on Facial Recognition Technology”, addresses the twin challenges of accuracy and interpretability in AI systems. It highlights how increasingly complex algorithms, while delivering more accurate results, often compromise explainability.
The paper also highlights:
- Challenges of balancing accuracy and explainability.
- Principles like Self-Explainable Systems, Meaningful Explanations, and Accurate Decision Justifications to build trust and accountability.
European Union
In March 2024, the European Parliament passed the AI Act, the world’s first comprehensive AI law, which will be effective from August 2026.
The AI Act underscores transparency as crucial for high-risk AI systems to counter their complexity, ensuring users can understand and effectively use them. The act also prioritizes transparency in high-risk AI systems through measures like:
- Design Transparency: Clear documentation of AI operations, potential risks, and fundamental rights considerations.
- Interaction Clarity: Informing users about AI-generated content and the automated nature of systems, especially in emotion detection and content manipulation.
These transparency measures aim to empower individuals to understand and navigate AI systems and content.
Australia
In September 2024, the Australian Government issued a Policy for the responsible use of AI in government, , marking a significant step toward positioning itself as a global leader in the safe and ethical use of AI. The policy underscores the need for AI to be used in an ethical, responsible, transparent, and explainable manner. It mandates that Australian Public Service (APS) officers must be able to explain, justify, and take ownership of AI-driven advice and decisions.
Earlier, in June 2024, Australia’s Data and Digital Ministers issued a National Framework for the assurance of artificial intelligence in government, outlining how governments can apply eight AI Ethics Principles to their AI assurance processes:
- Human, societal and environmental wellbeing
- Human-centred values
- Fairness
- Privacy protection and security
- Reliability and safety
- Transparency and explainability
- Contestability
- Accountability
Key measures for AI transparency and explainability include:
- Disclose AI Usage: Governments must inform users when AI is employed, maintaining a public register detailing the AI system’s purpose, intended use, and limitations.
- Reliable Data Practices: Governments should adhere to legal and policy standards for recording decisions, testing, and data assets, enabling scrutiny, knowledge continuity, and accountability.
- Clear Explanations: Governments must provide understandable explanations for AI outcomes, including:some text
- Inputs, variables, and their influence on system reliability.
- Testing results and human validation.
- Implementation of human oversight.
When explainability is limited, governments should document reasons for using AI and apply increased oversight.
- Human Accountability: In administrative decision-making, humans remain accountable for AI-influenced decisions, which must be explainable.
- Frontline Staff Support: Staff should be trained to explain AI outcomes, prioritizing human-to-human communication, especially for vulnerable groups or those uncomfortable with AI use.
United States
The National Institute of Standards and Technology (NIST) in June 2024 published the ‘Artificial Intelligence Risk Management Framework: Generative Artificial Intelligence Profile’, as a companion to the AI RMF 1.0, addressing cross-sectoral risks in Generative AI (GAI). Key trustworthy AI characteristics include fairness (with bias mitigation), safety, validity, reliability, explainability, and interpretability.
The framework highlights risks like GAI outputs producing confabulated logic or citations, misleading users into overtrusting the system. Suggested measures include applying explainable AI (XAI) techniques, such as gradient-based attributions, counterfactual prompts, and model compression, to continuously improve system transparency and mitigate risks. It also emphasizes validating, documenting, and contextualizing AI outputs to support responsible use and governance, recommending interpretability methods to align GAI decisions with their intended purpose.
US President Joe Biden issued an executive order (EO) on the 'Safe, Secure, and Trustworthy Development and Use of AI' in October 2023. The Executive Order calls on independent regulatory agencies to fully utilize their authority to protect consumers from risks associated with AI. It emphasizes the need for transparency and explainability, requiring AI models to be transparent and mandating that regulated entities can explain their AI usage.
The Blueprint for an AI Bill of Rights by the White House Office of Science and Technology Policy (OSTP) provides a non-binding set of guidelines for the design, development, and deployment of artificial intelligence (AI) systems. The document acknowledges the challenges posed by the opaque and complex decision-making processes of automated systems, which can undermine accountability. It stresses that these complexities must not be used as an excuse to avoid explaining critical decisions to those affected. Clear and valid explanations are identified as a baseline requirement to uphold transparency and accountability in automated decision-making.
Global Trends and Challenges in Explainable AI (XAI)
Global regulations are struggling to keep pace with the rapid evolution of AI technologies and their applications. The lack of a universally accepted definition of AI complicates efforts to regulate the field. Additionally, the absence of standardized metrics for measuring explainability poses difficulties for enforcing compliance.
Other key challenges include:
- AI systems are multi-faceted: AI systems are inherently diverse, with applications varying significantly across industries. Hence, a ‘one size fits all’ approach to regulating AI risks being overly stringent in some contexts while insufficient in others, highlighting the need for tailored regulatory frameworks that consider industry-specific requirements and challenges.
- Trade-off between accuracy and interpretability: AI systems, like deep neural networks and Large Language Models (LLMs) are inherently complex. Achieving higher accuracy often means increased model complexity, which in turn makes these systems more difficult to explain. Even when explanations are available, simplifying them for non-technical stakeholders remains a significant challenge, as it can risk losing critical nuances of the underlying decision-making process.
- Navigating Proprietary Algorithms: Many AI systems are built on proprietary algorithms, creating tensions between the need for transparency and the protection of intellectual property rights.
- Cultural Considerations: Global AI regulations must respect the diverse legal frameworks, societal values, and cultural norms across countries. As AI capabilities develop unevenly worldwide, there are challenges in policy and governance, with advancements and regulatory efforts often concentrated in more developed regions, potentially creating disparities in global AI standards and practices.
Conclusion
The need for robust regulations has never been more urgent. As AI technologies continue to shape industries and societies, it is imperative for global regulatory frameworks to evolve alongside them. Countries like India, the EU, Australia, and the US are taking crucial steps toward integrating explainability into their AI regulations, focusing on transparency, fairness, and accountability.
While there are considerable challenges in establishing effective AI regulations, these trends highlight the ever growing need for explainable AI (XAI), to ensure that AI predictions are not only accurate, but also understandable and justifiable to both technical and non-technical stakeholders. As regulations continue to catch up with the rapid advancements in AI, it is up to the organizations to ensure that transparency and accountability are prioritized in their AI deployments. This will not only ensure regulatory compliance, but also build trust with users, mitigate risks, and ultimately drive the ethical deployment of AI technologies.
SHARE THIS
Discover More Articles
Explore a curated collection of in-depth articles covering the latest advancements, insights, and trends in AI, MLOps, governance, and more. Stay informed with expert analyses, thought leadership, and actionable knowledge to drive innovation in your field.
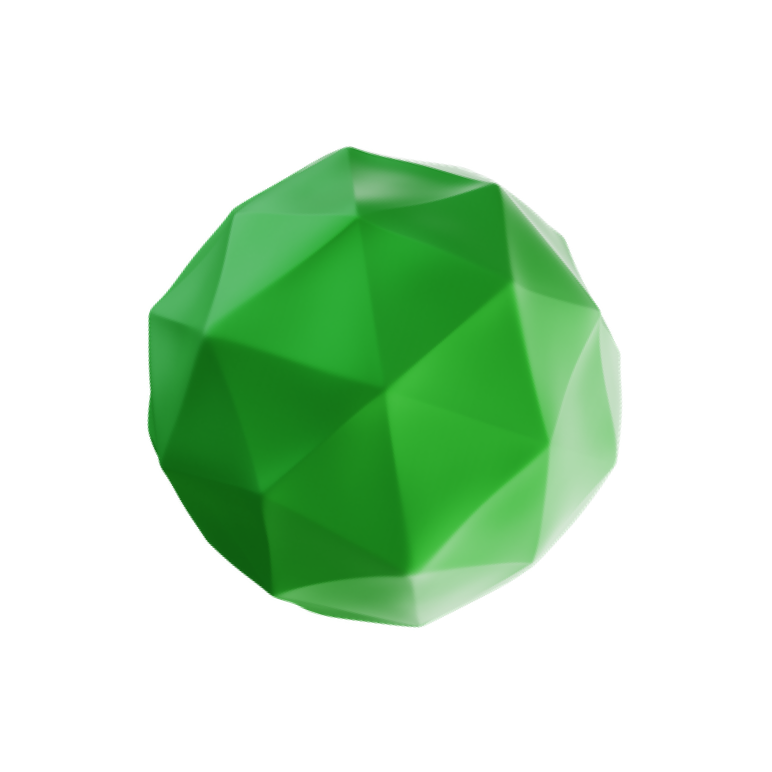
Is Explainability critical for your AI solutions?
Schedule a demo with our team to understand how AryaXAI can make your mission-critical 'AI' acceptable and aligned with all your stakeholders.