AI vs. Generative AI: Key Trends and Insights for 2025
8 minutes
February 7, 2025

Generative AI (Gen AI) is a transformative force that is reshaping industries and redefining business operations. As we navigate 2025, several significant trends illustrate both the potential benefits and the challenges of this technology. This blog discusses these developments and offers recent articles, reports, and expert insights on the subject.
What is Generative AI?
Generative AI is a class of AI systems that can generate new content by learning from patterns and structures in the training data. These systems utilize deep learning techniques and neural networks to create realistic outputs that closely resemble human-generated content.
Difference between Traditional AI vs. Generative AI
Unlike traditional AI, which analyzes data to make decisions or predictions, generative AI focuses on creating entirely new material, such as generating realistic images, crafting coherent text, or synthesizing audio.
Traditional AI is used for tasks like fraud detection and building recommendation systems, whereas Generative AI powers tools like ChatGPT and DALL·E, which facilitate the creation of dynamic and innovative content. By leveraging deep learning, Generative AI adapts to unstructured data, making it more flexible and innovative compared to traditional AI.
Generative AI Use Cases in BFSI:
- Fraud Detection: Gen AI has a positive effect on (fraud) detection by identifying intricate patterns and creating risk scenarios for risk analysis.
- Credit Scoring & Risk Assessment: Generate alternative credit risk models based on behavioral and transactional data.
- Customer engagement and Personalization: AI-powered chatbots and virtual assistants provide personalized financial advice and services.
- Document Automation: Streamline the creation of financial reports and legal documents, saving time and reducing the risk of human error.
- KYC Automation: Automate document verification, identity checks, and anomaly detection for compliance.
Generative AI Use Cases in Healthcare:
- Medical Imaging: Automate image analysis, assist with diagnostics, and enhance medical imagery for better decision-making.
- Drug Discovery & Development: Generative AI boosts drug discovery process by modeling chemical reactions, predicting compounds and simulating drug interactions.
- Virtual Health Assistants & Chatbots: Automate consultations, provide medication reminders, and monitor patient health metrics.
- AI-Generated Medical Literature & Research: Gen AI has the capability to summarize clinical research, predict drug interactions, and create knowledge graphs to support medical research.
- Personalized Treatment Plans: Based on patient data, Gen AI generates individual treatment plans that enhance the outcome.
Key Trends in Generative AI for 2025
Statistics on Generative AI
- Market Growth: The global generative AI market size is projected to reach US$62.72bn in 2025
- Adoption Rates: A 2024 global survey by McKinsey also presented that 65% of respondents in their organizations are regularly using gen AI in at least one business function. This adoption is most often in marketing and sales and in product and service development and IT functions.
- Increased investments: A 2024 report by Capgemini stated that 80% of organizations increased their investment in generative AI from last year, and 24% of organizations are now integrating generative AI into some or most of their locations or functions
1. Rise of Agentic AI
What is Agentic AI?
Agentic AI is designed to plan, execute, and learn through iterative feedback loops. This makes it far more dynamic and capable of handling complex, multi-step operations
Implications for Businesses:
Organizations using agentic AI can anticipate dramatic gains in efficiency. For example, in customer service, a customer might ask an AI chatbot about the status of their order, and the AI can provide an immediate response, reducing the need for human intervention. This transformation not only increases operational efficiency but also enhances customer satisfaction by significantly decreasing wait times.
2. Multimodal AI Development
Understanding Multimodal AI: Multimodal AI systems can simultaneously process and fuse multiple modalities of data, e.g., text, images, audio, and video. If there are richer interactions and more accurate outputs, this capacity is a major advantage. For instance, in the field of healthcare, multimodal deep reasoning AI can take into consideration patient medical records (text), medical image (visual) and even voice notes of physicians (audio), to achieve deep diagnostic understanding.
3. Rise of domain-specific LLMs
Generative AI's ability to tailor models for specific industries, applications, and datasets enables the training, fine-tuning, and optimization of large models for specialized domains. This empowers industries to leverage AI to generate precise, domain-specific insights, driving increased productivity, efficiency, and innovation across sectors such as healthcare, finance, legal, and beyond.
4. Composite AI will transform complex problem-solving
Composite AI integrates multiple AI techniques—such as machine learning (ML), knowledge graphs, natural language processing (NLP), deep learning, and symbolic reasoning—to tackle more complex and multi-faceted problems that a single AI approach cannot effectively solve on its own. By utilizing these diverse techniques, composite AI improves the accuracy, interpretability, and adaptability of AI systems across various domains.
Generative AI fuels Composite AI by enabling the integration of diverse techniques, creating systems that are more adaptive, context-aware, and capable of solving increasingly complex problems across industries. This approach is key to solving high-stakes, data-intensive, and multi-dimensional challenges in domains such as finance, healthcare, cybersecurity, and autonomous systems, where single AI models often struggle.
5. Importance of Unstructured Data
The Shift Towards Unstructured Data: Generative AI is revitalizing the significance of unstructured data—information that does not have a predefined data model or organization (e.g., emails, social media posts). Unlike structured data (like databases or spreadsheets), unstructured data doesn’t fit neatly into tables, making it more difficult to process and analyze using traditional methods.
Understanding how to analyze and process unstructured data is key for organizations looking to unlock the full potential of the vast amounts of information generated daily, allowing them to make data-driven decisions and improve operations across various domains.
6. Enhanced Conversational AI
The Evolution of Conversational Interfaces: With the continuing breakthroughs in natural language processing (NLP), Conversational AI is becoming more intuitive and capable of persistent and intricate interactions. These systems can now learn context, sentiment, and even nuances in speech, like emotion.
7. Focus on Data Security and Ethics
Navigating Ethical Challenges: As generative AI becomes more widespread, concerns about data security and responsible usage are on the rise. To safeguard confidential information, industries and organizations must exercise caution while also leveraging proprietary data to develop tailored algorithms.
8. Increased focus on AI Regulations
Regulation of AI Models: As Generative AI technologies continue to advance, there has been an increasing focus on their ethical implications, potential risks, and impact on society, leading to more government intervention. Governments are facing increasing pressure to establish clear policies, regulations, and ethical guidelines that address its risks and maximize its benefits. The scrutiny is aimed at ensuring that the technology is developed and deployed in ways that are safe, equitable, and transparent, promoting innovation while safeguarding society against potential harm.
Conclusion
Generative AI sits at the forefront of redefining the future of work by enhancing effectiveness, personalization and decision-making across all sectors. Organizations have to be aware of these trends and adapt their strategies to really benefit from generative AI.
To stay ahead in this rapidly evolving technological landscape, organizations should focus on key emerging trends such as agentic ability, multimodal capabilities, integration of unstructured data, and advanced conversational interfaces. By embracing these changes, companies can not only improve operational efficiencies but also maintain a competitive edge in an increasingly automated environment.
SHARE THIS
Discover More Articles
Explore a curated collection of in-depth articles covering the latest advancements, insights, and trends in AI, MLOps, governance, and more. Stay informed with expert analyses, thought leadership, and actionable knowledge to drive innovation in your field.
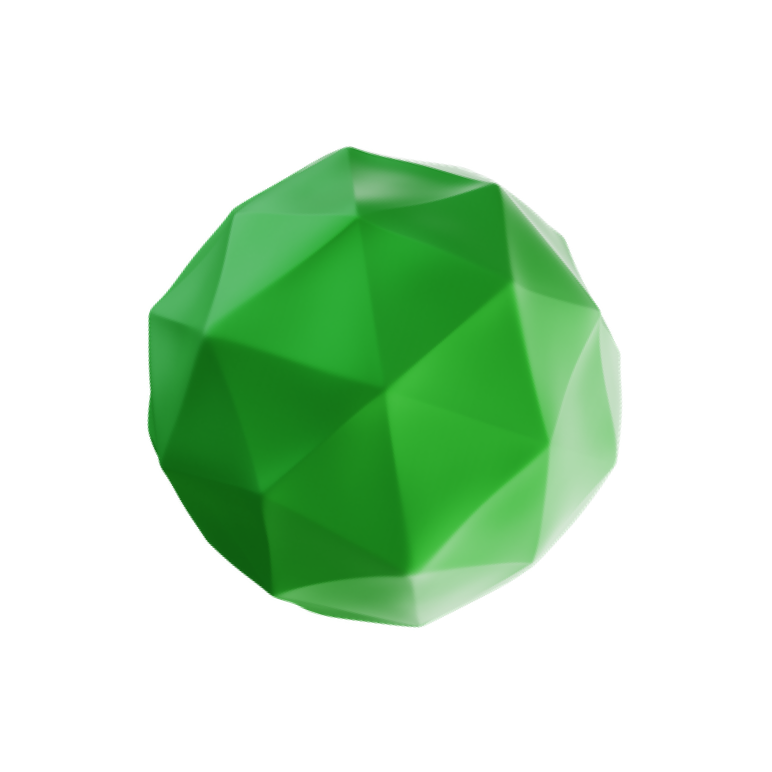
Is Explainability critical for your AI solutions?
Schedule a demo with our team to understand how AryaXAI can make your mission-critical 'AI' acceptable and aligned with all your stakeholders.