AI Broadcast Series #4: The Future of Agentic Stack
December 2, 2024
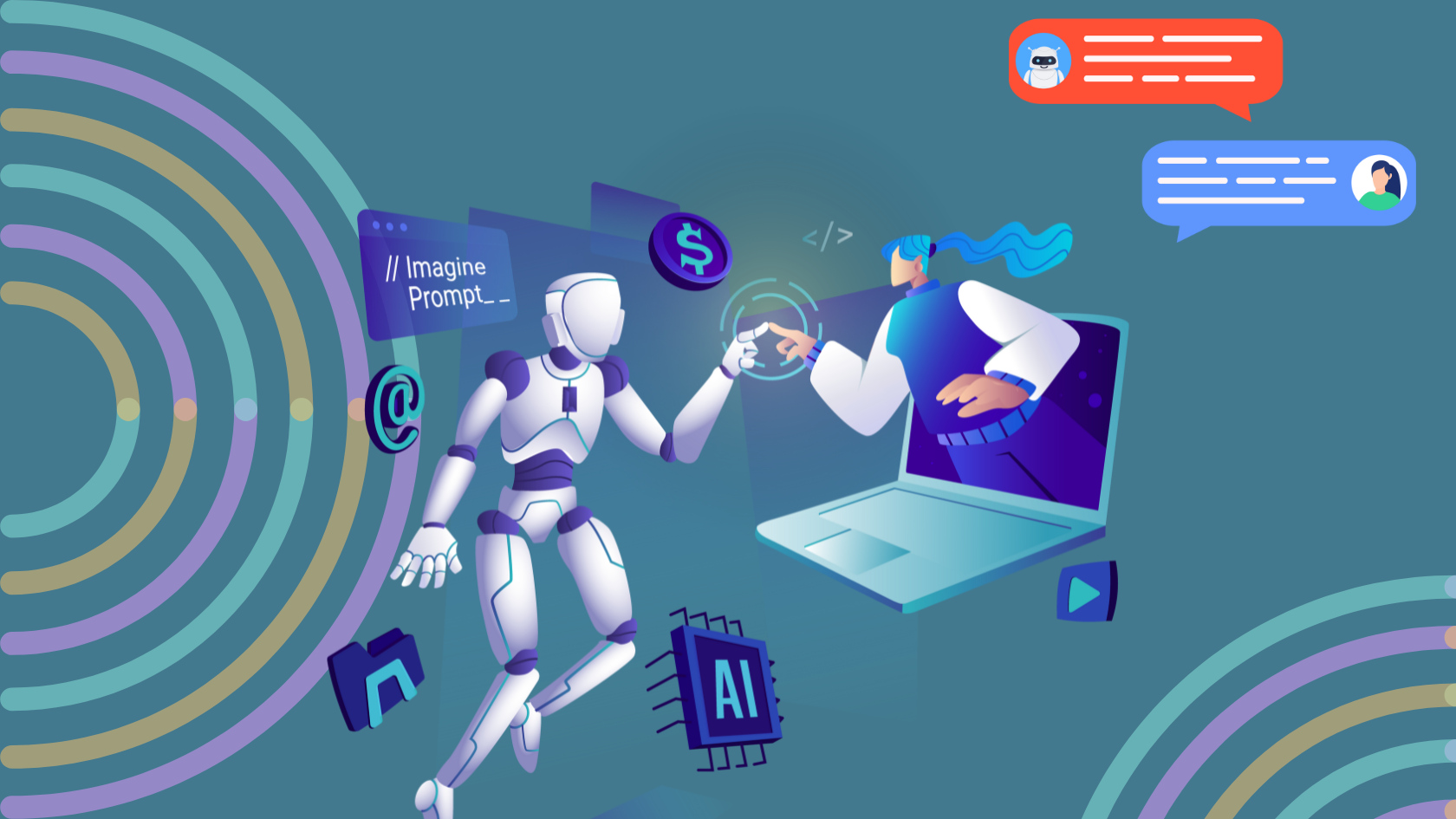
Listen to the fireside chat below:
Disclaimer - The information provided in this podcast is for informational purposes only and should not be construed as legal advice. The discussion of copyright and patentability of AI outputs is complex and subject to change, and it is important to consult with a qualified legal professional before making any decisions based on the information presented here. The statements made in this podcast are those of the speaker and do not necessarily reflect the views of any other organization or individual. The speaker does not warrant the accuracy, completeness, or timeliness of the information provided and disclaims any liability for any damages resulting from the use of the information herein.
Introduction
The AI Broadcast by Arya.ai is a bi-monthly event series that delves into the latest advancements, trends, and challenges in artificial intelligence. Each episode features in-depth discussions on groundbreaking AI topics such as Machine Learning, Generative AI, Responsible AI, and more. We’re excited to launch a brand-new theme: The Future of Agentic Stack in which we’ll explore how intelligent, autonomous AI agents—capable of making decisions, collaborating, and adapting in real time—are evolving and reshaping industries.
In our latest session, Vinay Kumar, Founder and CEO of Arya.ai, shared his thoughts on the transformative potential of Agentic AI and how it is shaping industries. The session explored the evolution of AI, key challenges, and how businesses can leverage agentic AI to stay competitive.
What is Agentic AI?
Agentic AI is shaping up to be a transformative leap in the field of artificial intelligence. As Vinay explained, it represents a significant evolution from traditional AI models that are often confined to predictive tasks or rule-based automation.
“Agentic AI is an evolution in how intelligence is consumed,” Vinay noted. “From knowledge assistants, co-pilots to autonomous agents, we’ve seen a gradual shift. These agents don’t just follow static rules; they interact with their environment, adapt, and improve over time.”
Unlike earlier AI systems, which primarily respond to predefined inputs or perform limited tasks, agentic AI is designed to plan, execute, and learn through iterative feedback loops. This makes it far more dynamic and capable of handling complex, multi-step operations. That said, the journey toward fully realized agentic AI comes with its share of challenges. Vinay acknowledged that current systems are still in their infancy. “What we see today are early versions—mostly focused on executing predefined actions rather than dynamically learning and evolving in real-time,” he explained. These systems remain largely one-directional, with limited reasoning or adaptability. Despite these limitations, agentic AI is poised to redefine how industries leverage artificial intelligence, opening the door to unprecedented capabilities and applications.
Applications & Challenges of Agentic AI
In the evolving landscape of AI, agentic AI represents an exciting and transformative step forward. As Vinay explained, agentic AI builds on foundational principles from reinforcement learning (RL). At its core, agents are learning functions that interact with their environment, reason, and act. The essential capability of an agent is to plan, execute, and learn.
However, Vinay pointed out that most current agentic AI systems are still quite limited in their learning and adaptability. “Right now, most agents are primarily one-directional,” he explained. “They either perform planning combined with some limited execution, or they don’t reason at all. There’s very little actual learning happening inside these agents. They focus on single-reason outputs rather than adapting dynamically, which limits their real potential.”
At the moment, the adaptation we see in these agents is primarily driven by structured prompts or CoT or basic reasoning capabilities, but this is still very rudimentary. Given the current scope of agentic AI and as we continue to develop these systems and expand the ecosystem, we’re likely to see more complex functions emerge.
Despite these constraints, Vinay pointed to some of the primary use cases for agentic AI today. One of the most successful applications is in knowledge distillation. For example, in customer care or customer success management (CSM), we see agents being used to sift through vast knowledge bases to provide precise, distilled answers. Instead of traditional IVR systems that require customers to press buttons, these agents facilitate fluid, conversational interactions,” Vinay explained. “This is where we’re seeing tremendous success and impact today.”
Beyond customer service, Vinay shared that agentic AI is also being deployed in sales pipeline automation and outreach management. “This is one of the most attractive use cases for agents right now. These agents automate tasks like cold outreach, follow-ups, and lead management. They’re performing a series of actions, but the reasoning behind those actions is still quite basic,” he said.
While the reasoning capabilities of these agents remain limited, the integration of function calling and template-based planning has made it easier to build and deploy such agents.
Overall, the use cases for agentic AI today are primarily focused on knowledge distillation and basic professional task automation, where these agents are augmenting human efforts rather than fully automating complex functions. Vinay acknowledged that while the technology is still in its early stages, the future holds great promise. “We’re beginning to see a shift from simple automation to more complex, adaptive agents. As these systems evolve, they will be able to tackle more sophisticated challenges,” he concluded.
Evolution from Traditional Automation to Agentic AI
A common mistake in AI development is confusing predictive models with agents. For example, a weather prediction model is a single-function system that takes input and generates an output, such as predicting the weather for the next hour. In contrast, agents use that output to take action and plan the next steps, refining their approach over time.
Currently, there's a lot of hype surrounding agentic AI, with marketing and sales often overselling its capabilities. “There's a significant gap between what agents are marketed to do and what they can actually achieve,” said Vinay Kumar. This mirrors the early days of ChatGPT in 2022, where expectations were set far beyond what the technology could deliver.
Vinay explained that tasks which seem simple can be complex from a model or agent architecture standpoint, while some complex tasks are easier to implement. “The challenge is properly categorizing and benchmarking these systems,” he said.
To build an effective agent, key questions must be asked: What is the agent supposed to do? Is there planning involved, or is it just executing predefined steps? Without planning, it’s simply a model or simple agent.
Understanding the Growing Interest in Agentic AI
The growing interest in agentic AI is driven by its potential to transform tasks that require planning and reasoning. Traditionally, planning is a bidirectional process involving execution, feedback, and revision. Agents, unlike single-function models, can handle this feedback loop, allowing them to improve and adapt over time.
Today, we are moving from simple, single-function models to multi-functional agents. These agents can not only predict outcomes, like weather forecasts, but also use that information to plan next steps, such as organizing travel. This capability opens up vast opportunities across various fields, from coding to sales to advisory roles.
In coding, for instance, feedback from logs helps refine the next steps in building applications or features. Agents can automate this process, making it more efficient and scalable. The excitement around agentic AI lies in its potential to tackle complex tasks that once required human reasoning.
However, we’re still in the early stages—what we’d call version 0.1. As Elon Musk says, it takes at least three product iterations to create a scalable product, and we’re just beginning this journey.
Evaluating Agentic AI for Business Needs
To understand the growing interest in agentic AI, it's useful to consider the complexity of tasks in professional careers. Let's take a data entry job - It can be simple like entrying invoice information or it can be complex like writing a analyst report! Both requires different skills even though the task is similar. Minimal reasoning tasks like invoice entry can be easily automated, as they follow straightforward steps. However, more complex tasks—like creating analyst reports—require higher cognitive skills, as they involve analyzing financial documents and synthesizing information. This illustrates how tasks within a single job can vary greatly in complexity.
Vinay highlighted, “Current agents can add significant value when tasks involve multiple steps and require some reasoning. However, as the number of steps increases, current agent architectures may struggle due to memory and processing limitations. That said, tasks with simpler feedback, like navigation, are easier for agents to handle, even with more steps.”
Complex tasks, like coding, are more challenging for agents since each step often depends on the previous one. In these cases, agents may not be able to perform effectively yet but it is slowlly becoming better through vertical agent focus.
Real-World Applications and Business Value of Agentic AI
Agentic AI is delivering significant value in well-defined, executed use cases. A great example is customer service, which has traditionally been human-dependent. While earlier automation attempts, like rule-based systems, struggled, AI-driven solutions are now transforming this space. For instance, Siri, introduced with iPhone 4S, was one of the first scaled efforts at automating customer service, and today, we are seeing realistic automation with substantial ROI. Companies like Uniphore and Nutanix have shifted from conversational analysis products to full conversational automation platforms, improving the value they offer.
Another growing area is product assistants or copilots, such as Microsoft’s solutions. These tools streamline tasks in software workflows, reducing manual steps and adding efficiency.
Sales and revenue operations also see an influx of investment, with AI automating tasks like cold calling and sending personalized messages. Agents can now leave personalized voicemails in a fine-tuned tone for the salesperson, automating outreach that traditionally requires human effort.
Core Components Enabling Agentic AI
The development of agentic AI is driven by key investments in memory management, function calling, orchestration, and planning. Efficient memory management is crucial, as it enables agents to tackle more complex tasks. Function calling is another important area, with startups providing a market place of functions that are plug-and-play to integrate tools like Google Drive or HubSpot etc, allowing users to focus on end-use cases instead of building these integrations from scratch.
Large language models (LLMs) are central to these advancements, improving agents’ ability to reason, plan, and process feedback. Once complex tasks, such as analyzing the feedback like in software testing, are now streamlined thanks to LLMs.
Emerging opportunities include optimizing learning, where agents can apply knowledge from past tasks, and improving computational efficiency. There are multiple experimental efforts around standardization like MPC introduced by Anthropic. But the ecosystem needs a lot to plan and execute. The benefits can outweigh the associated costs by making these systems more resource-efficient.
Introduction to Key Concepts like Autonomous Agents and Agentic Mesh
Reasoning is critical to making agents capable of solving complex problems. For example, in loan underwriting, models are trained to predict outcomes and analyze data, similar to how human underwriters use experience to make decisions. However, underwriting also requires staying ahead of trends and adapting, which is where reasoning becomes crucial. Predictive models carry extremely good historical memory, whereas reasoning agents are the answer to ‘on-event’ or multi-disciplinary decisioning.
To achieve this, we’re exploring how to distribute tasks across smaller specialized agents and combine their outputs for better reasoning. This concept is known as Agent Mesh, where multiple agents work together, sharing data and insights to improve the decision-making of the primary agent.
Humans are limited in scaling multi-disciplinary reasoning, but agents can be designed to handle such complex tasks. Like underwriting, agents combine validation, planning, and reasoning to provide more sophisticated outputs.
In the future, agents can also perform reverse validations and apply multiple critical reasoning processes before delivering a decision. This shift from rule-based systems to autonomous agents with reasoning capabilities will revolutionize how machines solve complex problems, allowing them to handle multi-layered tasks far beyond simple predictions.
Orchestration and Process Optimization
Orchestration and process optimization are crucial for the success of agentic AI. Effective orchestration enables agents to interact seamlessly with multiple tools and applications. Without this capability, agents become limited in their functionality. For example, if an agent needs to use five different tools, it must be able to interact with all of them thoroughly to execute tasks. Without exposing necessary functions, the agent can’t progress, highlighting the importance of orchestration.
This challenge is similar to the early days of robotic process automation (RPA), where systems struggled to scale due to integration limitations. Now, agents need the ability to connect to core systems like CRM and email to complete tasks effectively. Anthropic’s MCP protocol aims to standardize tool integration, making it easier for agents to interact across various applications.
However, integrating these functions requires substantial engineering, particularly with legacy systems that aren’t designed for this level of connectivity. While newer software uses APIs, exposing more complex functions requires balancing integration with security to prevent exploitation.
In conclusion, while the potential for agentic AI is immense, achieving seamless integration with various tools presents technical and security challenges. We are still in the early stages of this journey, but the rewards of overcoming these challenges are significant.
Explainability in Agentic AI
Full explainability in large language models (LLMs) is still challenging, making it harder to build clear explainability in AI agents. Today, explainability mostly relies on referencing the sources used to generate answers, but this doesn’t reveal how the model works internally. While models like perplexity can cite sources, they don’t explain the decision-making process.There have been growing efforts to make this happen. Like at our organization (AryaXAI), we’re focused on improving model transparency to enhance alignment, safety, and optimization, but scaling this remains a computational challenge. Anthorpic also has a reasonably large investment in model interpretability, whereas most other organizations focus more on applied AI.
How to benchmark agents?
There are multiple methods to benchmark agents. For example, a direct as-is comparison with a task like in customer service AI can be assessed by query resolution rates and Net Promoter Scores (NPS), just like human agents.
Traditional methods like the Turing Test aren’t ideal, as they focus on conversational quality rather than reasoning or task execution. Reasoning benchmarks need to evaluate two key factors: the number of steps to complete a task and the quality of those steps. Metrics like step count, step quality, cost, and task accuracy provide a realistic measure of performance.These are still in nascent stages. Businesses treat AI like hiring employees—choosing between humans and AI based on return on investment (ROI). The best resource is the one that delivers optimal value and outcomes.
Investments and Market Landscape
We’re witnessing a significant shift in enterprises moving from experimentation to execution in areas like customer care, product assistants, and knowledge distillation bots. . These have matured with a clear tested and validated architectures on implementation and robust guardrails have matured into a practical and scalable application. Use cases like advanced search and internal knowledge management have also gained traction. Tools such as Snowflake, Databricks, Perplexity, You.com etc have seen tremendous enterprise investments as these use cases have already delivering great ROI,
That said, more complex use cases, such as reasoning-driven agents, remain largely in the experimental phase. .
Beyond these, emerging areas like orchestration, explainability, guardrails, observability, and model serving are gaining attention. While foundational elements like scalability are stabilizing, innovations such as agent operating systems are creating new opportunities within the ecosystem. The market is ripe for innovation in these areas, but significant work remains to realize their full potential.
At this stage, fundamentals matter. Many investors prioritize end solutions addressing clear significant problems and delivering clear value to end users. As these use cases get validated, we will see more investments in the core agent stack. We can see a clear trend line of Silicon Valley investing more in the stack, whereas others investing more in the end use cases.
Summing up: Are these Stepping stones to achieving AGI?
The discussion wrapped up with an exploration of whether we are progressing toward Artificial General Intelligence (AGI). At its core, AGI requires reasoning across diverse tasks and delivering effective outcomes. Generalization is key—AGI must adapt its reasoning to tackle a wide range of problems.
While LLMs have shown rapid advancements, I believe AGI will emerge from a mix of technologies. LLMs are essential for task understanding and execution, but reinforcement learning (RL) is crucial for reasoning at scale. RL has already demonstrated its potential in reasoning applications like DeepMind’s AlphaGo.
Currently, these systems are experimental, but they point in a promising direction. AGI may initially achieve in 5-10% of tasks in the next 5 years but will grow over time as it bridges gaps across more domains.
However, we are still some distance from true AGI and our current focus should be on efficiency instead of brute force methods of scaling intelligence. As Ilia Sutskever noted, we’re reaching the limits of current training data, signaling a need to optimize architectures. The future of AGI lies in exploring new approaches, like mixture-of-experts models and layer swapping, which move away from brute-force methods to thought-driven solutions.
The path to AGI requires diversified research and development. We’re on the right track, but there’s still much to explore before we achieve true AGI.
References:
Unlock More Podcasts
Dive deeper into our podcast collection, featuring expert discussions, industry insights, and the latest trends in AI. Explore episodes that cover everything from cutting-edge innovations to practical applications in technology and beyond.1 SeenLike
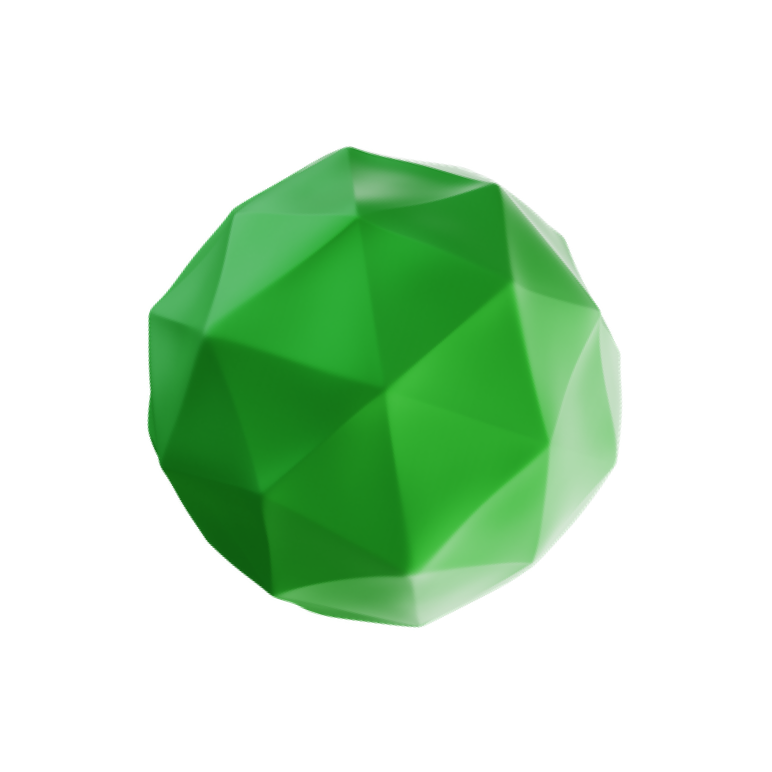
Is Explainability critical for your AI solutions?
Schedule a demo with our team to understand how AryaXAI can make your mission-critical 'AI' acceptable and aligned with all your stakeholders.