AI Broadcast Series #1 - What's next after ChatGPT in Banks, Insurers and Financial Services?
June 27, 2023
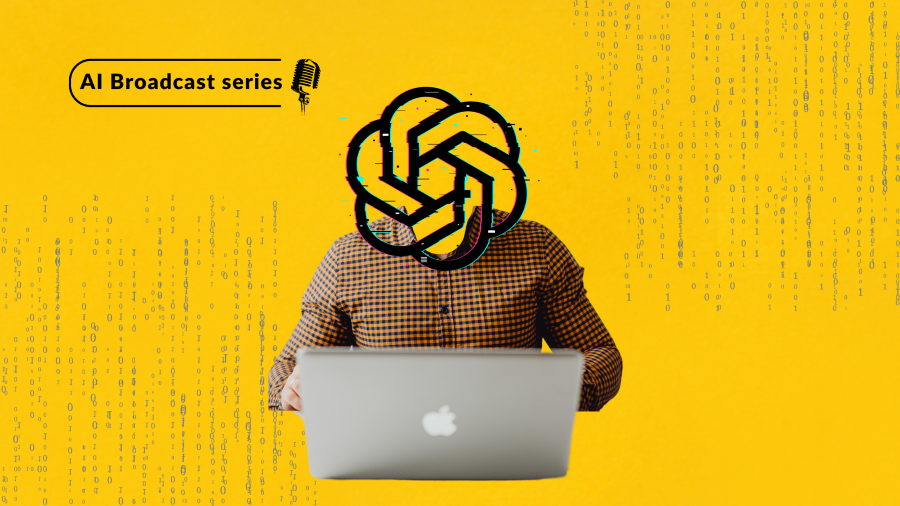
Listen to the podcast of this fireside chat below:
Disclaimer - The information provided in this podcast is for informational purposes only and should not be construed as legal advice. The discussion of copyright and patentability of AI outputs is complex and subject to change, and it is important to consult with a qualified legal professional before making any decisions based on the information presented here. The statements made in this podcast are those of the speaker and do not necessarily reflect the views of any other organization or individual. The speaker does not warrant the accuracy, completeness, or timeliness of the information provided and disclaims any liability for any damages resulting from the use of the information herein.
The 'AI Broadcast' by Arya.ai is a fortnightly event series where we explore the latest advancements, trends, and implications of AI. In each session, we will dive into an engaging discussion on a specific AI topic - like Machine Learning, Generative AI, NLP, responsible AI, and much more!
In the first session of the series, Vinay Kumar, Founder and CEO of Arya.ai, shares his insights on ChatGPT and Generative AI, examines how the narrative around generative AI evolves and how businesses can stay ahead by adapting to the current advancements and anticipating future developments.
There has been a lot of research, and a lot of talks around generative AI. A new research report from Bloomberg Intelligence predicted that the generative AI market, led by models like ChatGPT, is projected to surge from $67 billion to $1.3 trillion by 2032. We are poised to see an explosion of growth in the generative AI sector. We asked Vinay whether this would be another 'AI Hype' or if LLMs are here to stay.
"I think it all depends upon how well we can navigate the risk of using these models in production", said Vinay. "Of course, LLMs are already able to find use cases to which they are well adapted. For example, the code generator is a classic use case where LLMs are being deployed exhaustively today, but many more use cases exist. To support the hype, LLMs have to be a lot more democratized across multiple use cases."
“While there is a high probability that this is not a hype cycle”, said Vinay, “a lot of correction is happening from an expectations standpoint. The last six or nine months is the initial hype cycle. Now, we are figuring out to what extent it could be deployed, and what the challenges are. So this is more than hype, unlike the earlier cycles. There is a strong business opportunity that can be generated in the cycle”.
Applicability in FSIs
On the point of going beyond typical use cases, we asked Vinay about the most exciting and promising applications of LLMs beyond chatbots and customer support in FSIs. "I think LLMs are going to be really interesting for the financial industry", he explained. “Financial advisory is one of the very high-touch and very sensitive businesses. Typically, in financial advisory, the task is to do a lot of research, comprehend and then provide the suggestion. LLMs are very good at summarization; if not as direct advisors, LLMs are great assistants to these advisory functions.”
“Second, of course, is product support services. For example, in insurance, people may want to know what the policy contract is, how it is structured, what is covered, and what’s not covered, etc. This is beyond typical customer support and primarily around product support. This could be a realistic use case where we can see usage already happening in the market. And then you have more complex use cases like product assistants or famously called ‘copilots’, which are more advanced use cases.” Vinay explains further, “Let's say a user wants to do a specific transaction from A to B. Instead of doing these steps from the UI, they could probably give the command in simple text, and Product Assistants can execute the function in the application to complete the task”. While he thinks product-based AGIs will take a little more time, functions like advisory, customer support, or product support could see many use cases immediately in the financial services space.
Opensource vs Commercial LLMs
On the deployment side, there are also open-source options to explore in the market. We asked about the key differences and considerations between commercial foundation models and open-source foundation models and how organizations can navigate this decision. "In recent times, there has been a lot of buzz, particularly from the open-source community", Vinay continued, "OpenAI is pretty much a closed box at the moment. We know the GPT 3.5 architecture, whereas, for GPT 4, there is no official information regarding how these models are built. There is only a rumor that, it is an 8-way mixture model of 220B parameters each. This became a challenge for the open-source community, and many foundation models were being published." He shared a few studies published recently and a few examples of open-source models.
But he explains that there are problems with using open-source models.
“The first is benchmarking. Many open-source models or LLMs publish information such as performance metrics, which are not entirely reliable.” He gave an example of a recently published paper explaining that these matrices overfit the test data. “Many foundation models use data from ShareGPT, an open-source collection of conversations between ChatGPT and the customers. It was observed that some of these open-source LLMs are overly optimized on ShareGPT data, skewing the benchmarking metrics. Hence, it may seem like they are performing very well on ShareGPT data, but, as soon as they are applied to use cases, their performance degrades. This seems to be evident because, just for the sake of benchmarking, many open-source models are continuously focusing on achieving better-performing metrics, which may not be universalized or may be biased. So if FSIs are planning to use open-source LLMs, they should focus on building the capacity and capability to test these open-source models.
Secondly, there are techniques to induce attacks on these LLMs. For example, one could create training data more aligned with their goals and agendas and build a high-performing model on that training data. But eventually, it is skewed towards the builder's intentions. You could foresee more of such training data poisoning attacks.” Vinay shared another paper, highlighting a bunch of attacks and their examples. One is an attack at the LLM application layer. He further explained that anyone can induce prompts without the knowledge of the end user or the customer. The person can exploit these prompts, such as accessing sensitive customer information, phishing, providing wrong facts etc. Another attack is through plugins - backdoor access to plugins can be built to exploit the systems. So people are finding new ways to exploit the system and attacks are increasing.
“There are pros and cons to both approaches”, he adds, “Open-source has challenges related to how they have been trained, testing criteria etc. Whereas for commercial applications, the challenges could be data privacy, security, user experience, etc. The right investment for FSI is in figuring out the right testing metric or data and building the safety layer. This can differentiate one financial institution from another. In conclusion, he says that as long as the organizations can figure out the right acceptability criteria and the framework, they can deploy either model - open-source or commercial LLMs. Unless this layer is figured out, the challenges will remain, whether it is a commercial or an open-source model.”
Building proprietary LLMs
On asking whether financial institutions build the LLMs internally, Vinay responded that this would be the next phase. “We have seen very large LLM models with billions of parameters and trained on billions of tokens; the next phase is verticalization, like BloombergGPT. The players in Financial Services (like JP Morgan or Goldman), and brokering firms already have a ton of data they would want to train the model on. Hence, soon enough, people will start building their own LLMs.” He also shed some light on techniques that organizations can use to optimize the building process. (Mentioned in the resources section)
Hallucinations
The conversation shifted to model acceptance and safety aspects, tackling the question: Model hallucination and the possibility of mitigating hallucination. He responded that it's not achievable today, further explaining that's because of the design of the current transformer architecture. He said, “It works by learning to predict the next word in the sequence with a probability. This means, there is always a probability of seeing hallucination in the models.”
On controlling model hallucinations, he says, “One method is to define a context. When you are building an application on top of a foundation model, you can define and fix the context to control the hallucination”. Other technique mentioned was self-contradictory statements. He shared an example of a paper, which explains how contradictory statements can be identified. He added, "Changing the prompt to be more specific and reducing the number of response tokens are the other techniques which can be used to minimize hallucination. But on a foundation model level, it isn't possible to exclude hallucination, at least in the current state of architecture.”
Black box and transparency
Continuing the conversation about acceptance, he continued “The next challenge for the full-scale adoption of LLMs is explainability. Transformer models are quite challenging to explain. He mentioned OpenAI had tried to do explain the neural networks, but it truly doesn't explain the model functioning or exactly explain what is happening inside the model.” Although he did mention that a future architecture will be capable of providing us that explainability, or it can also continue to be a black box.
AI Regulations
With a lot of buzz around AI regulations, the conversation shifted to their impact on the usage of LLMs in financial services. "The regulations are coming not just for Generative AI, but the entirety of AI usage", he answered. "There is growing attention on bringing in regulations as soon as possible, because currently two very disturbing things are happening - One, LLMs are now making it quite easy to propagate misinformation at a mass scale, which is not possible to control. This is quite concerning, not just from AI safety but also from the societal factors standpoint. The impact would be quiet tremendous - our future generation simply can't use the historical data as it’d be simply full of biased or wrong facts! Second, it is now being democratized. So what stops somebody with wrong intentions from building a model for immoral purposes? Currently, there is nothing that can stop them. These are two very concerning things happening quite quickly, which is why many governing bodies across geographies are looking to regulate this as soon as possible.”
AGI and use cases in FSIs
Our concluding discussion was on whether AGI is close to being a reality and how can we see AGI being used in FSIs. Vinay's response was simple, yet backed by methodical reasoning: "Yes, why not? We have already seen nascent applications like BabyAGI - very rudimentary examples of how AGI would look like. But one of the fascinating things is, we actually proposed an architecture about 5-6 years back regarding how AGI would look. We thought it would be a reinforcement learning layer that defines tasks and then optimizes them. But LLMs are good at doing that already. This is a surprise for many people in the community. Now, OpenAI believes that LLMs can create that better quality general intelligence. This could be possible because AGI should outline, execute and modify the tasks. If it can do all these things efficiently, then that's a very good AGI.” He adds, "Maybe a year, or maybe 5-10 years down the line, but we are going to see this happen very quickly."
On how the Financial services industry can leverage AGI, he says, what we see is going to happen immediately is ‘Product AGI’, an AGI specific to the product. He shared a few possible use cases, explaining that every product will have an AGI bot, which can do everything inside the application over a command line conversation. “This can happen very soon, whereas strong AGI may take longer to deploy.”
The discussion concluded with a broader look at the future of LLMs. Vinay was of the opinion that “Input token limit is not going to be a challenge as there are now LLMs which support larger context, like Magic.dev claims to take in 5 million token context width.” Vinay reiterated the safety concerns of LLMs and having guardrails for efficient scaling. Organizations that experiment and develop a framework of validation and acceptance of such models will be the biggest benefactors of the LLM wave.
References used in the conversation:
- The false promise of proprietary LLMs: Link https://arxiv.org/abs/2305.15717
- Not what you've signed up for: Compromising Real-World LLM-Integrated Applications with Indirect Prompt Injection: https://arxiv.org/abs/2302.12173
- Go smol or go home: https://www.harmdevries.com/post/model-size-vs-compute-overhead/
- Textbooks Are All You Need https://arxiv.org/abs/2306.11644?ref=upstract.com
- Understanding Large Language Models (https://magazine.sebastianraschka.com/p/understanding-large-language-models
- LLM-Blender: Ensembling Large Language Models with Pairwise Ranking and Generative Fusion: Link (https://arxiv.org/abs/2306.02561)
- Three Takeaways After Exploring the Capabilities of Smaller, Open-Source LLMs: Link https://www.wwt.com/wwt-research/three-takeaways-after-exploring-the-capabilities-of-smaller-open-source-llms
- vLLM: Easy, Fast, and Cheap LLM Serving with PagedAttention https://vllm.ai/
- Self-contradictory Hallucinations of Large Language Models: Evaluation, Detection and Mitigation: https://arxiv.org/abs/2305.15852
- Language models can explain neurons in language models https://openai.com/research/language-models-can-explain-neurons-in-language-models
- LTM-1: an LLM with a 5,000,000 token context window https://magic.dev/blog/ltm-1
Unlock More Podcasts
Dive deeper into our podcast collection, featuring expert discussions, industry insights, and the latest trends in AI. Explore episodes that cover everything from cutting-edge innovations to practical applications in technology and beyond.1 SeenLike
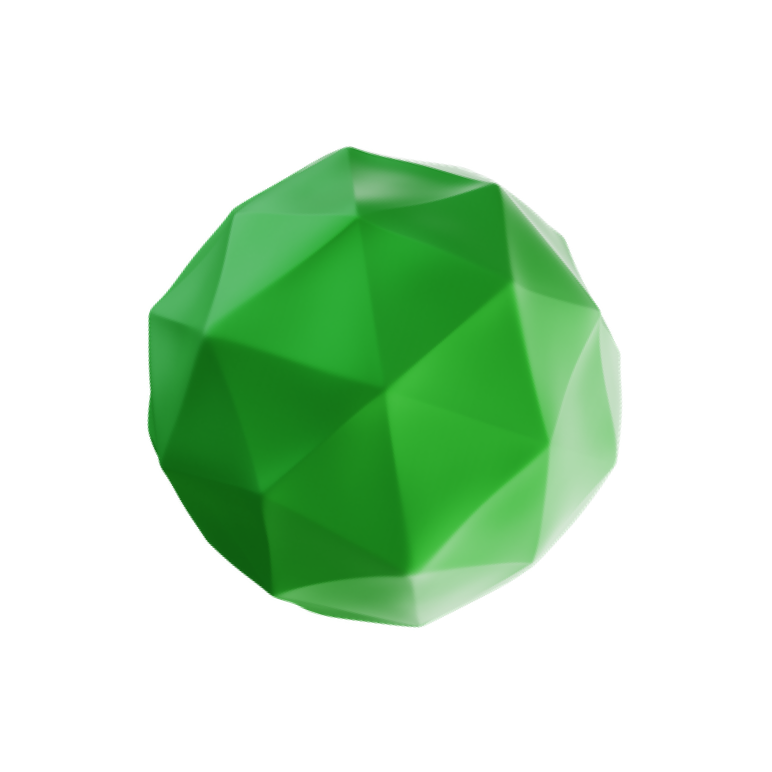
Is Explainability critical for your AI solutions?
Schedule a demo with our team to understand how AryaXAI can make your mission-critical 'AI' acceptable and aligned with all your stakeholders.