Why AI Risk Management Matters: Key Challenges and Strategies
7 minutes
February 3, 2025

Introduction
Artificial Intelligence (AI) has become a cornerstone of modern business and innovation, transforming industries from healthcare to finance, manufacturing to retail. With its ability to process vast amounts of data, automate complex processes, and enable predictive decision-making, AI has unlocked unparalleled efficiencies. However, alongside these advancements come significant risks that organizations must address to ensure ethical, transparent, and secure deployment of AI systems.
AI risks range from data privacy concerns, algorithmic biases, and security threats to regulatory non-compliance and lack of transparency in decision-making. As AI adoption accelerates, organizations must prioritize risk management frameworks to build trust, ensure fairness, and align AI applications with ethical standards.
Challenges in AI Risk Management
1. Data Privacy and Security
AI systems rely heavily on vast datasets to function effectively, but this dependence introduces serious privacy and security vulnerabilities. The more data an AI system processes, the greater the risk of exposure to cyberattacks, data leaks, and unauthorized access.
Key concerns include:
- Unauthorized Data Access: AI models often process sensitive personal, financial, or healthcare data, making them prime targets for cybercriminals. If not properly secured, these systems can lead to data breaches, identity theft, or financial fraud.
- Lack of Data Anonymization: Many AI models require personal user data to function. If organizations fail to anonymize or encrypt sensitive data, they risk violating privacy laws and exposing users to potential misuse.
- Regulatory Compliance Issues: Data privacy regulations such as the GDPR (General Data Protection Regulation) in the EU and the CCPA (California Consumer Privacy Act) impose strict rules on data collection, storage, and processing. Failure to comply can result in substantial fines and legal repercussions.
- AI Model Exploitation: Malicious actors can manipulate AI systems through adversarial attacks, feeding manipulated data into models to mislead their predictions. This is particularly dangerous in critical sectors like finance, healthcare, and cybersecurity.
2. Bias and Fairness in AI
AI models are trained on historical data, which often reflects societal biases. If not addressed, these biases can lead to unfair and discriminatory outcomes, negatively impacting hiring processes, loan approvals, law enforcement, and more.
Challenges associated with AI bias include:
- Discriminatory Hiring Practices: AI-powered recruitment tools have been found to favor certain demographics while discriminating against others. For example, Amazon’s AI-driven hiring tool was discontinued after it was found to penalize resumes containing the word “women’s.”
- Racial and Gender Bias in AI: Studies have shown that AI facial recognition systems are significantly less accurate in identifying individuals from marginalized racial and gender groups, leading to wrongful arrests and discrimination.
- Credit Scoring and Loan Approval Biases: Financial institutions using AI-based credit scoring systems risk denying loans to specific demographic groups due to historical biases in their training data.
Difficulty in Identifying and Mitigating Bias: Biases in AI models are often subtle and difficult to detect without extensive auditing and fairness assessments.
3. Lack of Transparency (Black Box Problem)
One of the most significant challenges in AI risk management is the lack of explainability in complex models. Many deep learning systems operate as "black boxes," meaning that even developers struggle to understand how they arrive at decisions.
Key transparency concerns include:
- Uninterpretable Decision-Making: AI-driven decisions in critical areas like healthcare, finance, and criminal justice must be explainable. A lack of interpretability can lead to distrust and potential legal liabilities.
- Challenges in Auditing AI Models: Organizations struggle to audit and validate AI systems, particularly in highly regulated industries. If AI decision-making processes remain opaque, companies may face compliance challenges.
- Accountability Issues: When AI systems make mistakes, determining who is responsible—the organization, the developers, or the AI itself—becomes a complex issue. This raises ethical and legal questions that must be addressed.
4. Regulatory and Compliance Challenges
AI regulations are evolving rapidly, making compliance a moving target for organizations. Governments worldwide are implementing AI-specific laws, requiring businesses to stay ahead of regulatory changes to avoid legal consequences.
Key challenges include:
- Global Variability in AI Laws: Different regions have different AI regulations. For instance, the EU AI Actcategorizes AI systems based on risk levels, while the U.S. follows a more sector-specific approach. This inconsistency complicates compliance for multinational companies.
- Difficulty in Defining AI Liability: Legal frameworks are still evolving to determine liability when AI systems cause harm. Establishing who is responsible for AI errors—whether developers, businesses, or the AI itself—is a growing challenge.
- AI Ethics vs. Business Objectives: Companies must balance ethical AI deployment with profitability. Ensuring compliance while maintaining efficiency can be challenging, particularly in competitive industries.
Best Practices for AI Risk Management
1. Establish Robust Governance Frameworks
AI governance ensures that organizations follow ethical, transparent, and regulatory-compliant practices when developing and deploying AI models.
- Create AI Ethics Committees: Cross-functional teams including ethicists, legal experts, and AI researchers should oversee AI-related decisions.
- Implement AI Policies & Guidelines: Organizations should establish clear policies on AI usage, data privacy, and ethical AI development.
- Align AI Governance with Industry Standards: Companies should follow globally recognized frameworks such as NIST’s AI Risk Management Framework to ensure compliance and best practices.
2. Continuous Monitoring and Auditing
Real-time monitoring and periodic audits help detect AI errors, biases, and security threats before they escalate.
- Deploy AI Risk Assessment Tools: Automated tools can track AI system performance, flagging potential biases or risks in real time.
- Use Model Interpretability Techniques: Techniques such as LIME (Local Interpretable Model-Agnostic Explanations) and SHAP (SHapley Additive Explanations) help explain AI decision-making.
- Establish AI Compliance Dashboards: Real-time dashboards can provide insights into AI performance, helping businesses stay compliant with evolving regulations.
3. Stakeholder Engagement and Transparency
AI development should involve multiple stakeholders, including businesses, policymakers, academia, and the general public.
- Engage with Regulators & Industry Groups: Partnering with AI regulatory bodies ensures alignment with legal requirements.
- Open Source & Explainable AI Initiatives: Encouraging open-source AI development promotes transparency and collaboration.
4. Employee Training and AI Awareness
AI governance is only effective if employees understand their role in responsible AI deployment.
- Implement AI Ethics Training Programs: Regular training sessions can educate employees about AI risks, bias detection, and compliance standards.
- Define Clear AI Usage Policies: Employees must be aware of the limitations and appropriate uses of AI within the organization.
Case Studies: Organizations Successfully Managing AI Risks
1. RAZE Banking: Fraud Detection with AI
Challenge:
RAZE Banking, a multinational financial institution, was facing a surge in fraudulent transactions, leading to significant financial losses and reputational risks. Traditional fraud detection systems, which relied on rule-based algorithms, struggled to keep up with evolving fraud tactics such as synthetic identity fraud, account takeovers, and real-time phishing attacks.
AI Implementation:
To address these challenges, RAZE Banking deployed AI-driven predictive analytics and machine learning-based fraud detection models that continuously analyze transaction patterns in real time. The system was designed to:
- Monitor millions of transactions per second, flagging unusual activity based on historical transaction behavior.
- Utilize neural network-based anomaly detection to identify deviations from a customer’s normal spending patterns.
- Implement adaptive learning algorithms that evolve with emerging fraud patterns, reducing false positives and improving detection accuracy.
Outcomes:
- 45% reduction in fraudulent transactions within the first six months.
- 20% improvement in regulatory compliance efficiency by automating fraud investigations and reducing manual intervention.
- Enhanced customer trust, as real-time fraud alerts and automated authentication improved security while maintaining a seamless user experience.
2. Network International: AI for Secure Payments
Challenge:
Network International, one of the largest payments providers in the Middle East and Africa, faced increasing risks of credit card fraud, unauthorized transactions, and cybersecurity breaches. With a growing number of online transactions, manual fraud detection methods were proving to be insufficient, leading to high rates of chargebacks and revenue losses.
AI Implementation:
The company adopted machine learning-based fraud detection to enhance transaction security. Their AI system was trained using vast datasets containing millions of historical transactions, allowing it to:
- Identify fraudulent transactions in real time by detecting anomalies in purchasing behavior.
- Implement behavioral biometrics, analyzing keystrokes, device usage, and location-based data to detect fraudsters impersonating genuine users.
- Reduce false positives by using a multi-layered AI model, which combines supervised and unsupervised learning to distinguish genuine transactions from fraudulent ones.
Outcomes:
- Significantly reduced financial losses by detecting fraudulent transactions within milliseconds, preventing unauthorized purchases before they were completed.
- Improved transaction approval rates, ensuring that legitimate customers experienced fewer payment disruptions.
- Enhanced regulatory compliance, reducing the burden of fraud-related audits and chargeback disputes.
3. TowneBank: AI for Compliance & Risk Management
Challenge:
TowneBank, a U.S.-based financial institution, faced increasing regulatory pressure to comply with anti-money laundering (AML) laws, the Bank Secrecy Act (BSA), and other financial regulations. The manual compliance process was time-consuming, prone to errors, and inefficient, leading to increased operational costs and regulatory scrutiny.
AI Implementation:
To streamline compliance and reduce human error, TowneBank implemented AI-driven compliance automation, focusing on:
- Automated document processing: AI-powered natural language processing (NLP) systems were used to analyze and verify compliance reports, reducing the need for manual reviews.
- Real-time transaction monitoring: AI models continuously scanned transactions to flag suspicious activities that might indicate money laundering or fraud.
- Regulatory reporting automation: AI-generated reports ensured that compliance filings met regulatory deadlines with accurate and up-to-date data.
Outcomes:
- Reduced compliance costs by automating manual reporting and document verification.
- Lowered regulatory risk by ensuring real-time tracking of suspicious transactions and compliance with evolving financial regulations.
- Improved operational efficiency, allowing compliance teams to focus on high-risk cases rather than spending time on routine checks.
Conclusion
AI has immense potential to drive innovation and efficiency, but its risks must be managed proactively. Organizations must adopt robust governance frameworks, continuous monitoring, stakeholder engagement, and employee training to ensure responsible AI deployment.
By learning from real-world case studies and staying updated on evolving regulations, businesses can balance innovation with ethical AI practices, fostering trust among consumers and stakeholders.
As AI regulations continue to evolve, staying ahead in AI risk management will not only mitigate legal and reputational risks but also unlock long-term competitive advantages.
AI Risk Management Starts with Explainability – Use AryaXAI to monitor, align, and optimize your AI models for compliance and reliability. Talk to Our Experts today!
SHARE THIS
Discover More Articles
Explore a curated collection of in-depth articles covering the latest advancements, insights, and trends in AI, MLOps, governance, and more. Stay informed with expert analyses, thought leadership, and actionable knowledge to drive innovation in your field.
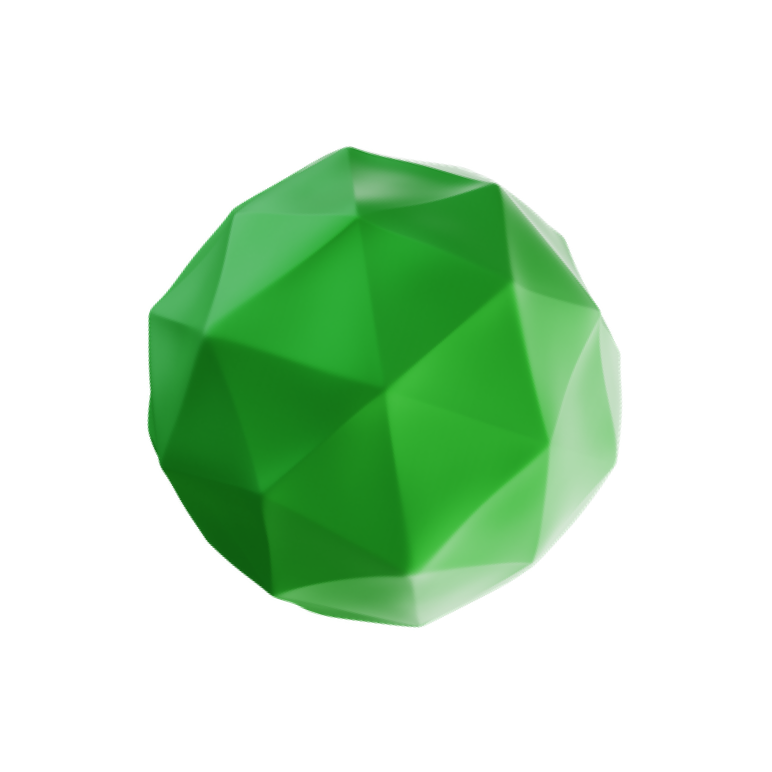
Is Explainability critical for your AI solutions?
Schedule a demo with our team to understand how AryaXAI can make your mission-critical 'AI' acceptable and aligned with all your stakeholders.