The AI black box problem - an adoption hurdle in insurance
August 29, 2022

AI usage in the insurance industry is in the early adoption phase, and its influence and capability has made remarkable progress in the past few years. While existing rule based systems used by insurers are handy, they require codifying the logic and rules, and can only handle standard cases. Codifying decision logic in these systems is challenging, and implementing it is time-consuming, requiring multiple iterations in the process. Even after the initial implementation of the decisioning system, making minor adjustments is a cumbersome task, which can be undertaken by only a handful of experts - rendering these systems ‘rigid’ with no flexibility to influence the outcome.
Insurance companies then turned to AI systems and predictive models, after realizing the need for an additional tool to automate decisions where it was not feasible to create rules-based logic. Many companies have already started their innovation programs by automating existing rule sets with machine learning models to bring automation in existing processes. These models can make automated decisions across vast quantities of data. Yet, insurers are quite skeptical about AI adoption for core processes, their main concern- the ‘black box’ of AI driven decisions.
Explaining AI decisions after they happen is a complex issue, and without being able to interpret the way AI algorithms work, companies, including insurers, have no way to justify the AI decisions. They struggle to trust, understand and explain the decisions provided by AI, since the system only provides a view of the input and output, but reveals nothing of the process and workings in between. Owing to the self-learning nature of AI and machine learning systems, the rules are continually being updated by the system itself. This was not the case with traditional rule based systems - the rules did not update or change on their own, and explainability was never a challenge since there were fixed outcomes based on the rules written by users.

So, how can a heavily regulated industry, which has always been more inclined to conservatism than innovation, start trusting AI for core processes?
This led to a new field of research research - Explainable AI aka XAI, which focuses on understanding and interpreting predictions made by AI models. Explainable AI lets us peek inside the AI ‘black box’, to understand the key drivers behind a specific AI decision. Users can more readily interpret the input that is driving the output, and decide on the level of trust on the decision.
This improves confidence of the users and business leaders, opening up new ways to create advanced controls on the system. Users who are managing the system become more wary of the variables driving the decisions, and can use their own expertise to optimize the model, and understand if additional variables might lead to further precision in the decision. Over time, this exercise also leads to a more fine-tuned and a precise model.
Moreover, this creates transparent communication between the customer and insurer, and even auditors. Insurance decisions and the logic for transactional decisions needs to be stored and retrieved for posterity for a variety of reasons like audit, claims, compliance, etc. Interpreting the decision logic ensures trust and transparency, and clear accountability in the decision process, without sacrificing the AI performance. This paves the way for regulatory compliance and helps overcome the dilemma between accuracy and explainability.
Adopting AI technology has become a survival criteria in the industry. AI has potential benefits that impact all stages of the business value chain, and will continue to do so. While the pressure mounts from all sides - customers, competition and regulators, explainable AI provides the much needed ‘insurance’ to insurers by ensuring that the companies enjoy the benefits of AI while remaining compliant.
SHARE THIS
Discover More Articles
Explore a curated collection of in-depth articles covering the latest advancements, insights, and trends in AI, MLOps, governance, and more. Stay informed with expert analyses, thought leadership, and actionable knowledge to drive innovation in your field.
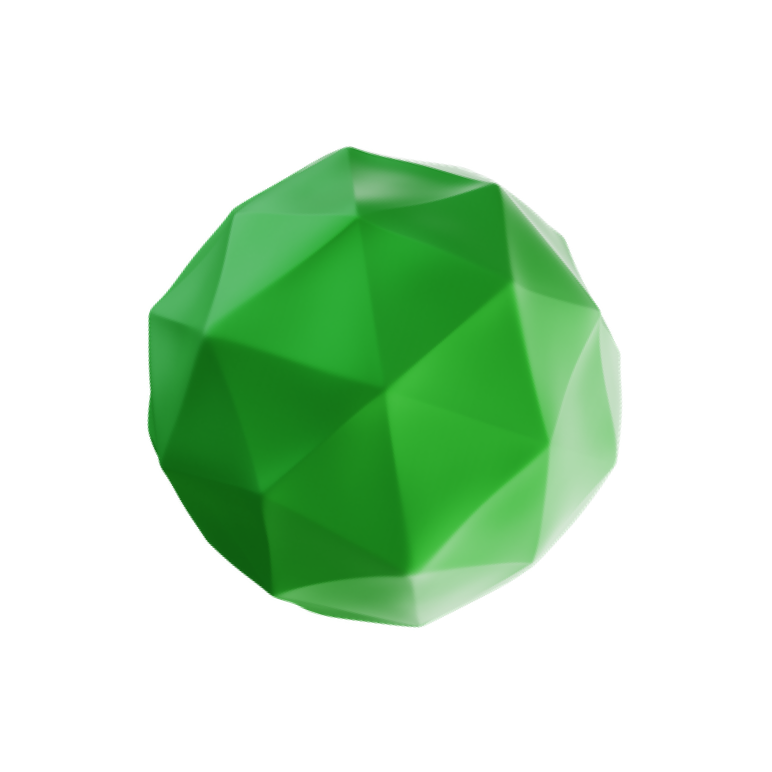
Is Explainability critical for your 'AI' solutions?
Schedule a demo with our team to understand how AryaXAI can make your mission-critical 'AI' acceptable and aligned with all your stakeholders.