Explainable AI: Enhancing Trust, Performance, and Regulatory Compliance
8 minutes
December 12, 2024

Artificial Intelligence (AI) is increasingly woven into the fabric of modern life, influencing everything from healthcare decisions to financial transactions. As these systems grow more complex, the ability to explain their decisions—known as AI explainability—has become a cornerstone for trust, adoption, and ethical responsibility. This blog explores the critical role of explainability in AI, with real-world examples, sector-specific benefits, and challenges in balancing interpretability with performance.
What is Explainable AI?
Explainable AI is a powerful method of understanding how and why an AI model has reached a particular decision, making AI explainable, transparent and reliable. Explaining a model entails explaining all aspects of the model that are:
- Prediction-related: How the model arrived at its prediction and the features that influenced it.
- Model-related: How the model processes data and what it has learned.
- Data-related: How training data was used and any potential biases.
- Influence and Controls: Factors affecting the system and mechanisms for control.
Why is explainability needed in AI?
No matter how accurate and robust an AI/ML model is, its decisions may not gain stakeholder acceptance without trust and understanding of how those decisions are made. This is particularly critical in highly regulated industries like Insurance and Financial Services, where confidence and accountability are paramount. To ensure that the solution enjoys confidence and trust, it is important to build additional transparency layers and controls for the users.
While state-of-the-art models continue to push the boundaries of AI, their complexity often comes at the cost of interpretability. Advanced models like deep neural networks deliver high accuracy but operate as "black boxes," making their decision processes opaque. Simpler models, such as decision trees, are inherently more interpretable but typically fall short in predictive performance compared to their complex counterparts. This trade-off between interpretability and performance remains one of the key challenges in AI.
As highlighted by McKinsey & Company, mastering explainability provides significant advantages for technology, business, and risk professionals, helping them enhance trust, improve decision-making, and manage risks effectively:
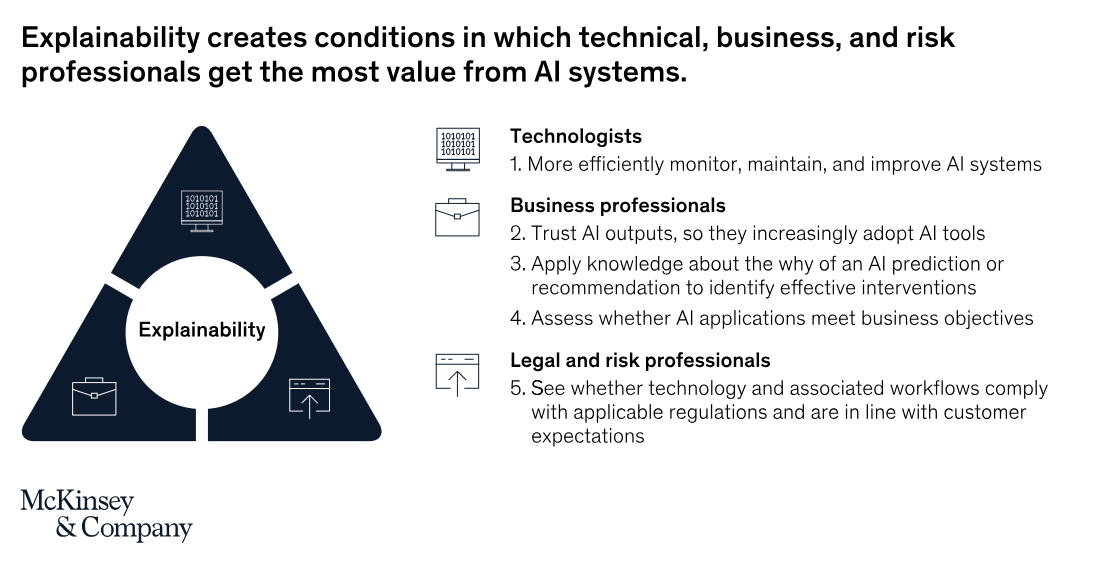
Additionally, due to the self-learning nature of AI and machine learning systems, the rules are continually being updated by the system itself, as they interact with new data. This dynamic behavior amplifies their complexity, making explainability an indispensable component of responsible AI development. Explainability is crucial for several key reasons:
- Model acceptance: The black box nature of AI models makes it difficult for users to trust, understand and explain the decisions provided by AI, since the system only provides a view of the input and output, but reveals nothing of the process and workings in between. Explainable AI bridges this gap between system functionality and human comprehension, fostering model acceptance. Users are more likely to trust and accept the model if they understand the inner workings of the model.
- Enhance model performance: To debug a DL model, one needs to investigate a broad range of causes. The models have larger error space and require longer iterations, making them uniquely challenging to debug. Further, the lack of transparency leads to lower trust and an inability to fix or improve the model. AI explanation will ensure that the model behaves as expected, and provide opportunities to improve the model or the training data.
- Investigate correlation: Traditionally ML models operate through opaque processes - you know the input and the output, but there is no explanation as to how the model reached a particular decision. Explainability brings complete transparency in the process, enabling the users to investigate correlations between various factors and determine which factors have the most weightage in the decision.
- Enable advanced controls: Transparency in the decision process can enable the user to supervise the AI model, and adjust model behavior rather than operating in the blind.
- Ensuring unbiased predictions: AI systems often inherit biases present in their training data. Knowing the reasons behind a particular decision prevents undesirable bias within the data.
- Ensuring compliance with regulations: AI regulations have emerged as one of the most pivotal drivers of the need for explainable AI. Across the globe, regulatory bodies are collaborating to establish frameworks that prioritize transparency and accountability in AI systems. For example, the EU's General Data Protection Regulation (GDPR) underscores transparency as crucial for high-risk AI systems to counter their complexity, ensuring users can understand and effectively use them.
The cost of unexplained AI
The absence of AI explainability has caused significant challenges across industries, leading to operational failures, legal complications, and public mistrust.
Recruitment Bias: Amazon’s Hiring Tool
Amazon's recruitment tool used machine learning to sort through job applications and identify the most promising candidates. The tool was trained on a dataset of resumes submitted to Amazon over a 10-year period, which predominantly featured male candidates. As a result, the tool learned to prioritize resumes that contained terms or phrases that were more commonly used by men, such as "executed" or "captured". Any ML model is trained to be a generalized query solver. However, because of the high male population in the I.T. Industry as well as the keywords used in their resume, the model was misguided and led to believe that if the gender is Male, the resume is more likely to be selected - purely because of data imbalance and keyword association. This led to the tool discriminating against female candidates, as their resumes were less likely to contain these terms and, therefore, less likely to be selected.
Autonomous Vehicles: Tesla and Uber Incidents
On March 18, 2018, a self-driving Uber test vehicle fatally struck a pedestrian in Arizona. The AI failed to identify her as a pedestrian or predict her movements, exposing critical gaps in decision-making. Similarly, Tesla is facing U.S. federal probes into its "Full Self-Driving" system following multiple crashes, including a fatality, raising concerns about its reliability and safety.
Financial Industry Failures: Credit Scoring Systems issue with Equifax
In 2017, credit reporting agency Equifax had an issue with its systems that led to inaccurate credit scores for millions of consumers. The discrepancies, in some cases exceeding 25 points, impacted approximately 300,000 individuals significantly. These inaccuracies potentially led to wrongful denials of credit for certain borrowers, raising concerns about the integrity of the scoring process.
While Equifax did not directly attribute the problem to its AI systems, there has been speculation that the company's use of AI in credit score calculation may have contributed to the errors.
Healthcare Misdiagnosis: IBM Watson for Oncology
IBM Watson for Oncology was designed to assist in cancer treatment recommendations. However, it produced incorrect or unsafe recommendations in some cases. Healthcare professionals found it challenging to trust the system due to the lack of clear explanations for its decisions. The project faced backlash, with hospitals eventually discontinuing its use in some cases.
Explainable AI use cases
Explainability opens new opportunities, particularly in high-risk sectors:
- Healthcare: Explainable models can guide clinicians by clarifying how input variables, such as symptoms or test results, contribute to a diagnosis. XAI can also help researchers in drug discovery. It can help understand why specific drug candidates are selected during the discovery process. This transparency supports better decision-making and reduces liability.
- Finance: Transparent credit-scoring systems, and fraud detection algorithms can foster fairness, ensuring compliance with anti-discrimination laws and building customer confidence.
- Legal: AI systems used in legal research and predictions must clarify how case precedents and laws were applied. This ensures accountability and ethical alignment
- Autonomous Vehicles: Self-driving systems require explainability to understand and mitigate failures, such as misclassification of objects on the road, to ensure safety and public trust.
Conclusion
Explainability is no longer an optional feature in AI systems—it is a necessity for ethical, trustworthy, and widespread adoption. By ensuring that AI decisions are transparent and justifiable, organizations can prevent failures, foster trust, and unlock vast opportunities in sensitive industries. Moreover, explainability is fundamental to Responsible AI, a framework that emphasizes fairness, accountability, and transparency in AI implementations. Businesses adopting this methodology embed ethical principles into their AI systems, ensuring trust and fostering widespread adoption.
As AI evolves, the focus must remain on balancing performance with interpretability to meet both technical and human-centric goals.
SHARE THIS
Discover More Articles
Explore a curated collection of in-depth articles covering the latest advancements, insights, and trends in AI, MLOps, governance, and more. Stay informed with expert analyses, thought leadership, and actionable knowledge to drive innovation in your field.
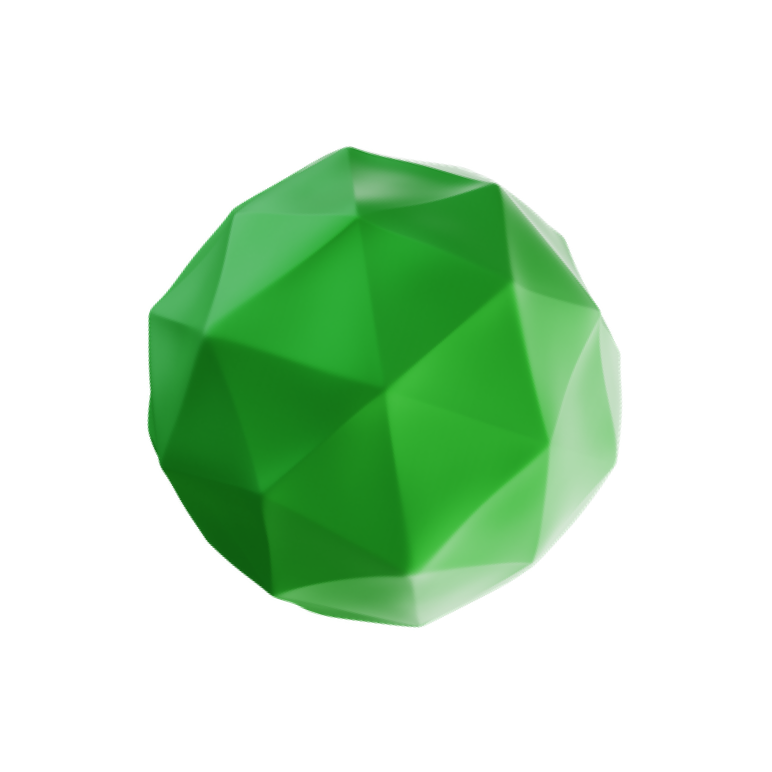
Is Explainability critical for your AI solutions?
Schedule a demo with our team to understand how AryaXAI can make your mission-critical 'AI' acceptable and aligned with all your stakeholders.