Track slippages and deliver consistency in production


Provide confidence.
Model Drift Monitoring: Track & identify data issues in production
Deliver consistency to your models in production by preemptively tracking for failure and intervening proactively when required.
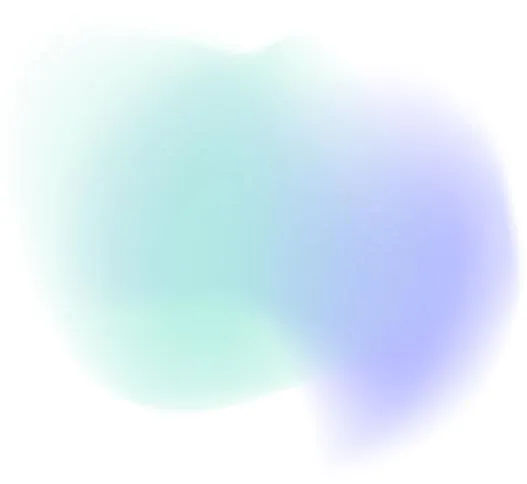
Monitor model drift
Model drift can severely impact model performance, one of the common reasons for model failure in production. If not tracked, it will untraceable and slippages will never be corrected.
AryaXAI tracks model drift in real-time and provides the root cause for the model failures. Users can define the drift thresholds and get notified proactively.
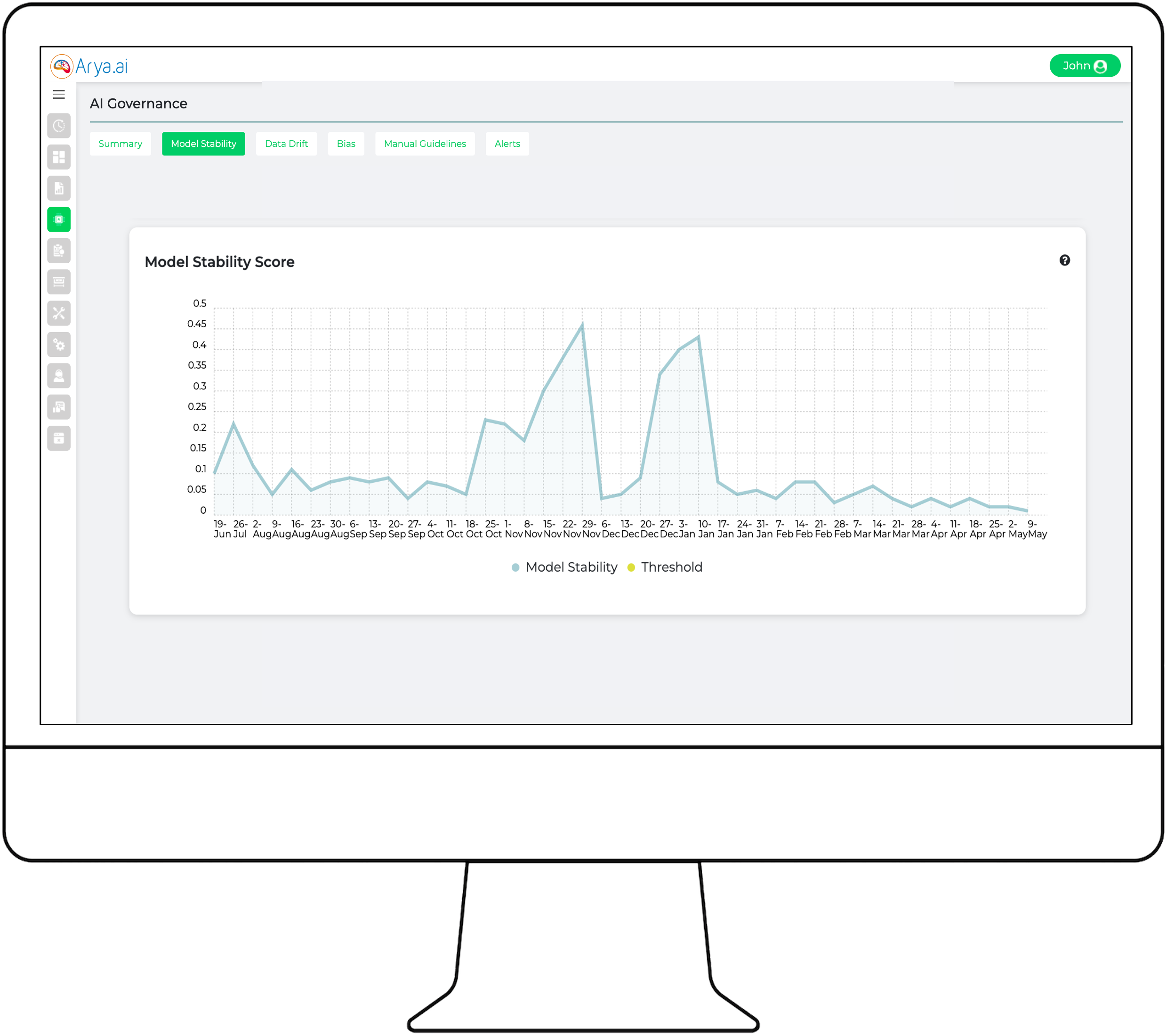
Track model drift proactively
Customize the thresholds as per your risk profile
Prevent model failure ahead of time
Define the preferred tracking metrics from the options
Monitor model performance
Tracking model performance is very tough for use cases where the feedback is coarse or delayed. For mission-critical applications, such delayed feedback can be risky.
In addition, to mapping actual model feedback, AryaXAI can estimate the model performance so that users can assess the model performance preemptively and course-correct the usage.
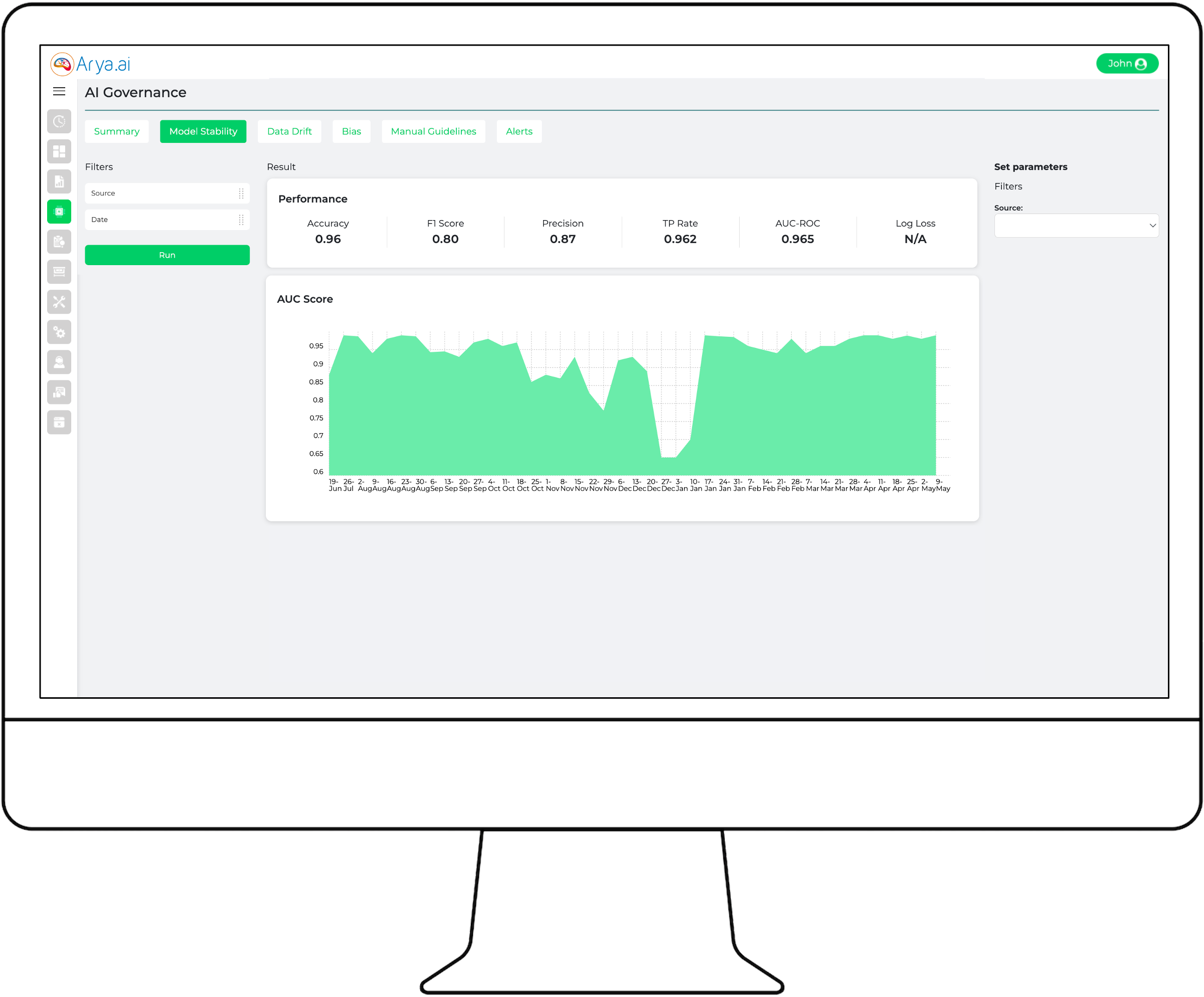
Track various model performance metrics
Define alerts and get notified about the performance deviation
Ensure consistency in model performance
Slice and dice the sample size and calculate dynamically
Track slippages.
Monitor data drift proactively and prevent decay in model performance
Track how the data drifts in production from simple variations to complex relational changes. Analyze predictions in relation to entire data sets or specific groups
Keep ML models relevant in production
Catch input problems that can negatively impact model performance.
AryaXAI helps monitor even the hard-to-detect performance problems to
prevent your model from growing stale.
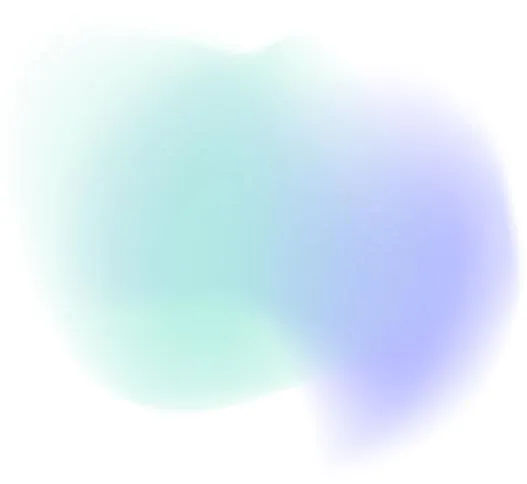

Better understand how to begin resolution
With real-time alerts, automatically identify the potential data and performance issues so you can take immediate actions. Easily pinpoint drift across thousands of prediction parameters.
Scalable monitoring in real time
Flag data discrepancies by slicing data into groups
Define the recursive actions for data drift scenarios
Complete visibility into model behaviour across training, test and production
Identify when and how to retrain models
Be fair.
Track and mitigate deep-rooted model bias before it is exposed
Detect, monitor and manage potential risks of bias to stay ahead of regulatory risks.
A more efficient way to debias ML models
AI bias in models can cost organizations heavily. ML models replicate or amplify existing biases, often in ways that are not detected until production
AryaXAI prevents your models from producing unfair outcomes for certain groups

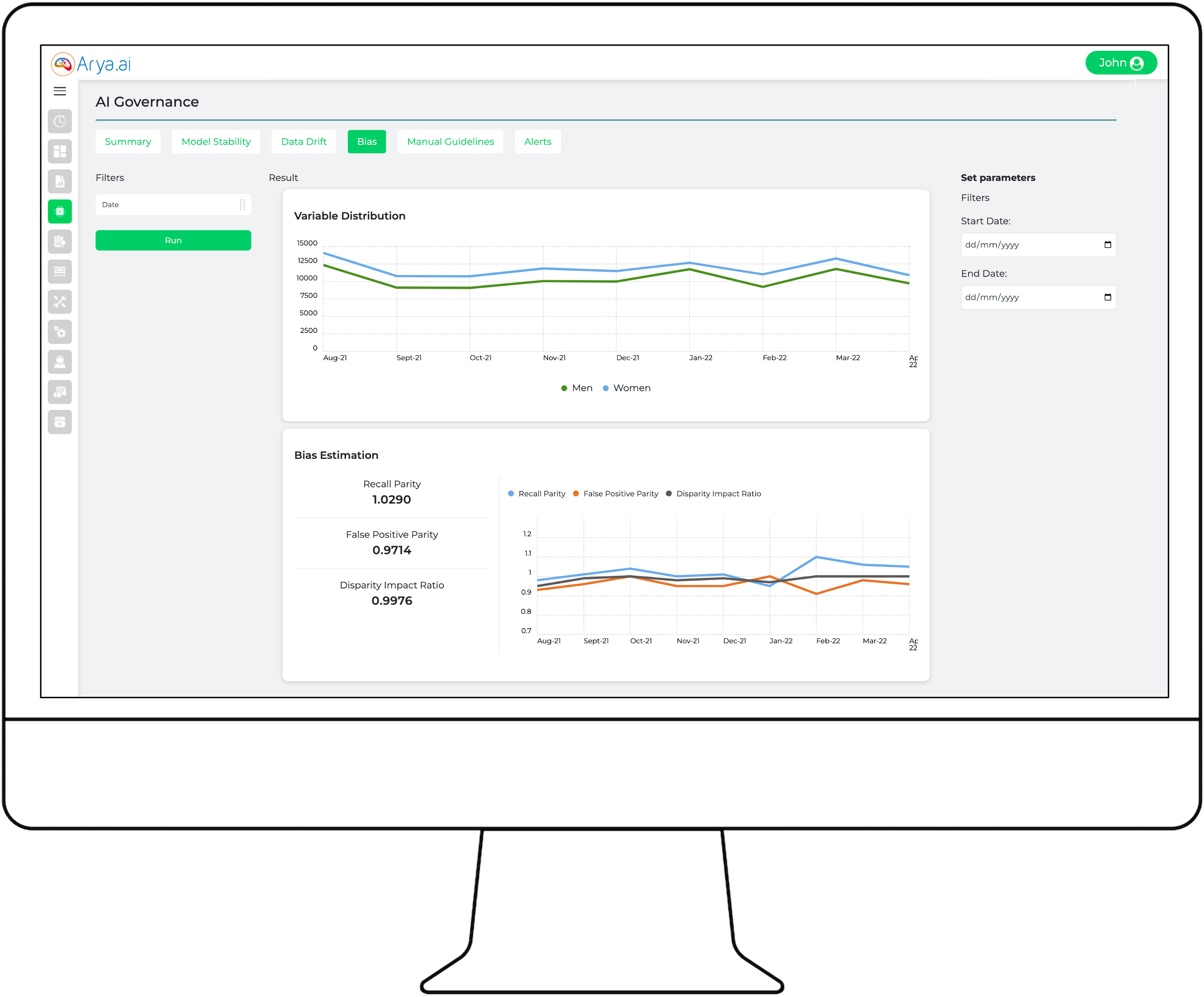
Navigate the trade-off between accuracy and fairness
Mitigate unwanted bias with a window into the working of your ML model. Routinely and meticulously scrutinize data to identify potential biases in the early stages and appropriately correct them.
Bias is real!
Selection bias
Measurement bias
Information bias
Sample bias
Exclusion bias
Algorithm bias
Ensure fairness in predictions
Detect and mitigate AI bias in production
AryaXAI uses multiple tracking metrics to identify bias
Track model outcomes against custom bias metrics
Customize bias monitoring by defining the sensitive pool
Improve model fairness to reduce business risk